Development and validation of a machine learning-based nomogram for prediction of intrahepatic cholangiocarcinoma in patients with intrahepatic lithiasis
Introduction
Intrahepatic cholangiocarcinoma (ICC), the second most common primary liver cancer in humans after hepatocellular carcinoma, is highly malignant and has an extremely poor prognosis (1-3). The incidence of ICC has been increasing over the last several decades (4-6), and the disease is more prevalent in Far Eastern countries, such as China, Japan, South Korea, and Thailand (1) than Western countries (7). The risk factors for ICC are complex, but intrahepatic lithiasis (IHL) had been confirmed as a strong risk factor recently. High odds ratio (OR) had been found for developing ICC with hepatolithiasis, up to 50 in Korea (8), 6 in China (9), and 7 in Italy (10). Studies have reported that 2.3% to 13.0% of hepatolithiasis patients eventually developed cholangiocarcinoma (11-17). In addition, it was estimated that 65–70% of patients who underwent resection for cholangiocarcinoma had concomitant hepatolithiasis in Taiwan (18,19).
Although the progression from IHL to ICC is not fully understood, it has been postulated that prolonged irritation and inflammation of the biliary epithelium by the calculi, bile stasis, and bacterial infections are the main causes of ICC caused by hepatolith (11,20). Ultimately, the chronic inflammation of the intrahepatic biliary tract epithelium and atypical epithelial hyperplasia can lead to malignant transformation of the epithelial cells (21-23). The progression of cholangitis to ICC is a long process, and both of them often exist simultaneously. It is very difficult for a clinical surgeon to identify ICC early in patients with IHL because there are no specific symptoms and radiological features. Although tissue biopsy can confirm a histological diagnosis, it is not routinely recommended or necessary in ICC (24), especially in IHL-ICC because a ‘negative’ biopsy does not exclude ICC given the potential for sampling error. In fact, the probability of ‘negative’ biopsy is higher in IHL-ICC due to concurrent chronic inflammation and necrosis caused by calculi. The preoperative diagnostic accuracy of IHL-ICC is low, generally ranging from 30% to 65% diagnosed by combination of imaging, biopsy, serum carcinoembryonic antigen (CEA), and cancer antigen 19-9 (CA 19-9) (7,11,12,17,25). Recently, we have increased the diagnostic accuracy to 78.5% through developing a nomogram for patients with IHL complicated by radiographically diagnosed mass (26). However, the nomogram did not include the IHL-ICC without radiographically diagnosed mass. Therefore, there is an urgent need to develop a nomogram to improve the diagnostic accuracy of ICC in all patients with IHL.
In this study, we aimed to identify the risk factors of ICC for IHL in a large sample, develop a user-friendly nomogram for preoperative identification of ICC among these patients IHL, and validate its predictive capacity in two independent data sets. We present the following article in accordance with the TRIPOD reporting checklist (available at https://hbsn.amegroups.org/article/view/10.21037/hbsn-20-332/rc).
Methods
Training population and study design
The study population consisted of all patients with IHL who underwent hepatectomy or biopsy in The First Affiliated Hospital of Wenzhou Medical University (WZMU) from January 2000 through July 2018. After checking the pathology reports of lesions, 35 patients were found ineligible, of which 26 individuals who underwent surgical resection for the lesions rather than those originally revealed by imaging, 9 cases were pathologically indicative for other types of tumors (3 cases of hepatocellular carcinoma, 4 cases of atypical hyperplasia and 2 cases of adenoma). The present study eventually included 2,269 patients with IHL who were confirmed as intrahepatic biliary inflammation (IBI) or ICC by postoperative histopathological examination. Those patients were divided into two groups including IHL with IBI (IHL-IBI) group and IHL with ICC (IHL-ICC) group. Except for 9 cases of IHL-ICC confirmed by biopsy, all the others were confirmed by hepatectomy.
Details for recruitment and selection of the patients in this study were shown in Figure 1. Preoperative comprehensive radiological diagnosis upon ultrasound imaging (US), computed tomography (CT) or magnetic resonance imaging (MRI) was performed by two senior board-certified radiologists who were not given any information about histopathological diagnosis and other clinical data, and was defined as follows: (I) inflammatory mass (IM) or inflammation of bile duct (IB) was considered when imaging exhibited (i) typical echogenic debris, septations, or gas on sonography; or (ii) hypodense hepatic mass on CT or high signal intensity in center at DWI on MRI with regular margin compared with normal liver tissue through the arterial phase to delayed phase; or (iii) uniform thickening of bile duct wall; (II) suspicious cancer or suspicious lesion was suggested when imaging lacked typical features of abscess and ICC; (III) cancer was considered when imaging demonstrated heterogeneous minor peripheral enhancement with gradual enhancement centrally and irregular margins; and (IV) any IM or suspicious cancer could not been found. If different results were got separately, the two radiologists would discuss together to determine the final results. Inter-observer agreement rate was got by calculating the Kappa-Cohen index.
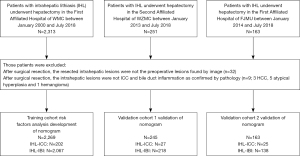
The study was conducted in accordance with the Declaration of Helsinki (as revised in 2013). This retrospective study was reviewed and approved by the Institutional Review Board (IRB) of The First Affiliated Hospital of Wenzhou Medical University (No. WZhosp1-2000-1). All procedures performed in studies involving human participants were in accordance with the ethical standards of IRB, and a waiver of written informed consent was granted by the IRB due to the retrospective nature of this study in which de-identified data were used and analyzed.
Statistical analysis and development of nomogram
Continuous variables were presented as mean ± standard deviation (SD) or median (range) and compared using Mann-Whitney U test. Frequency data were presented as number and percentage and compared using the chi-square test or Fisher’s exact test where appropriate. The performance of tumor markers and imaging was assessed using the ROC curve analysis. The machine learning algorithms including Lasso regression and random forest were used to identify important features out of the available features. Lasso regression can handle the multicollinearity problem of the available features, and random forest enables the implementation of variable selection procedures based on their impact on the prediction of the outcome. The factors predictive for IHL-ICC were also identified through univariate and multivariate stepwise logistic regression analyses. OR and 95% confidence interval (CI) were calculated to estimate the strength of the association between individual risk factors and ICC. Factors found to be significantly associated with ICC in the univariate analysis and machine learning algorithms were included in the multivariate analysis. The optimal cutoff points of continuous variables were selected by SPSS’s Optimal Binning method. Bin formation is optimal with respect to a categorical guide variable that “supervises” the binning process. Bins can then be used instead of the original data values for further analysis. Some discretized variables can be treated as categorical for use in procedures that require categorical variables. All statistical analyses were carried out using SPSS (version 18.0; SPSS Company, Chicago, IL, USA) and R software version 3.22 (http://www.r-project.org). The machine learning algorithms were carried out using the R package ‘caret’. The reported statistical significance levels were all two-sided, with statistical significance set at 0.05.
A nomogram was constructed based on the machine learning algorithms and the results of multivariate analysis to predict the probability of ICC. The discriminative power of the model was quantified in terms of discrimination and calibration. The Hosmer-Lemeshow test was used to assess the fitness of the nomogram (P>0.05 indicating good fit) (23). Calibration of the model was checked by plotting the predicted probabilities against the actual probabilities. Discrimination was quantified by the area under the receiver-operating characteristic (ROC) curve (AUC) and 95% CIs were estimated. Sensitivity, specificity, and positive predictive values (PPV) and negative predictive values (NPV) and their 95% CIs were calculated for various cutoff points of the calculated risk score.
External validation was performed by using the nomogram for patients from The Second Affiliated Hospital of WZMU and The First Affiliated Hospital of Fujian Medical University (FJMU). DeLong’s test was applied to estimate the difference between the two ROC curves of the nomogram developed from the training cohort and validation cohort.
Results
Demographic and clinicopathologic characteristics
Data were obtained and analyzed on 2,269 patients in the training cohort, of whom 202 cases were confirmed to have ICC and 2,067 were confirmed to have IBI. Demographic characteristics as well as laboratory and clinical data on the IHL-ICC and IHL-IBI groups are summarized in Table 1. In order to facilitate the statistical process, some continuous variables were classified according to the optimal binning method, including age, alkaline phosphatase (ALK), CA 19-9 and CEA (Table 1). Univariate analysis showed that the potential risk factors for the development of ICC in IHL were age, biliary tract operation history, cholecystectomy, total duration of IHL, inflammatory attacks within half a year, comprehensive radiological diagnosis, ALK, alanine aminotransferase (ALT), albumin, prothrombin time (PT), alpha fetal protein (AFP), CA 19-9, CEA, and CA 125 (all P<0.05) (Table 1). Data of validation cohort were obtained and analyzed on 245 patients including 27 cases of IHL-ICC and 218 IHL-IBI (Table 1).
Table 1
Demographic or characteristic | Training cohort | Validation cohort 1 | |||||
---|---|---|---|---|---|---|---|
IHL-IBI (n=2,267) | IHL-ICC (n=202) | P value | IHL-IBI (n=218) | IHL-ICC (n=27) | P value | ||
Age, mean (SD) | 58.43 (11.70) | 64.64 (10.35) | <0.001 | 58.92 (10.71) | 65.67 (10.82) | 0.002 | |
Sex (F/M) | 1,413/654 | 137/65 | 0.938 | 156/62 | 20/7 | 0.962 | |
Smoking | 292 (14.1%) | 28 (13.9%) | 1 | 22 (10.1%) | 3 (11.1%) | 1 | |
Alcohol | 228 (11.0%) | 26 (12.9%) | 0.501 | 28 (12.8%) | 3 (11.1%) | 1 | |
Personal cancer history | 48 (2.3%) | 3 (1.5%) | 0.604 | 4 (1.9%) | 0 (0.0%) | 1 | |
Family cancer history | 45 (2.2%) | 1 (0.5%) | 0.175 | 0 (0.0%) | 0 (0.0%) | 1 | |
Cholecystectomy | 484 (23.4%) | 75 (37.1%) | <0.001 | 45 (20.6%) | 15 (55.6%) | <0.001 | |
Biliary tract surgery times, mean (SD) | 0.22 (0.57) | 0.38 (0.67) | <0.001 | 0.20 (0.55) | 0.67 (0.92) | <0.001 | |
Total duration of IHL (year), mean (SD) | 7.51 (10.34) | 9.14 (12.05) | 0.034 | 4.90 (9.46) | 5.73 (8.94) | 0.664 | |
Inflammatory attacks within half a year (≥2 times) | 82 (4.0%) | 31 (15.3%) | <0.001 | 16 (7.3%) | 3 (11.1%) | 0.001 | |
Comprehensive imagological diagnosis | <0.001 | <0.001 | |||||
No mass-related lesion found | 1,506 (73.0%) | 62 (30.7%) | 113 (51.8%) | 5 (18.5%) | |||
Inflammatory mass | 294 (14.3%) | 28 (13.9%) | 63 (28.9%) | 5 (18.5%) | |||
Suspicious of cancer | 248 (12.0%) | 69 (34.2%) | 41 (18.8%) | 6 (22.2%) | |||
Cancer | 15 (0.7%) | 43 (21.3%) | 1 (0.5%) | 11 (40.7%) | |||
Location of hepatolithiasis | 0.611 | 0.492 | |||||
Left lobe | 508 (66.75%) | 103 (73.57%) | 141 (64.68%) | 21 (77.78%) | |||
Right lobe | 193 (25.36%) | 32 (22.86%) | 52 (23.85%) | 5 (18.52%) | |||
Left and right lobes | 44 (5.78%) | 4 (2.86%) | 18 (8.26%) | 1 (3.70%) | |||
Caudate lobe | 16 (2.10%) | 1 (0.71%) | 7 (3.21%) | 0 (0.00%) | |||
Lesion size (cm), mean (SD) | 5.12 (1.69) | 5.72 (1.32) | 0.234 | 5.51 (1.95) | 5.92 (1.68) | 0.338 | |
Symptoms | |||||||
Abdominal pain | 1,875 (90.7%) | 165 (81.7%) | <0.001 | 188 (86.2%) | 21 (77.8%) | 0.377 | |
Fever | 851 (41.2%) | 68 (33.7%) | 0.056 | 70 (32.1%) | 7 (25.9%) | 0.665 | |
Vomiting | 806 (39.0%) | 48 (23.8%) | <0.001 | 64 (29.4%) | 0 (0.0%) | 0.002 | |
Jaundice | 282 (13.6%) | 29 (14.4%) | 0.862 | 14 (6.4%) | 2 (7.4%) | 1 | |
Weight loss | 48 (2.3%) | 9 (4.5%) | 0.107 | 4 (1.8%) | 0 (0.0%) | 1 | |
Ascites | 59 (2.6%) | 15 (7.3%) | 0.064 | 5 (2.29%) | 2 (7.4%) | 0.059 | |
Others | 27 (1.3%) | 5 (2.5%) | 0.302 | 2 (0.9%) | 0 (0.0%) | 0.88 | |
Laboratory | |||||||
ALK (U/L), mean (SD) | 168.28 (161.99) | 224.70 (193.14) | <0.001 | 158.31 (139.77) | 189.88 (158.17) | 0.286 | |
γ-GT (U/L), mean (SD) | 231.11 (292.87) | 231.47 (240.42) | 0.988 | 251.49 (356.56) | 173.46 (169.33) | 0.273 | |
ALT (U/L), mean (SD) | 94.07 (142.92) | 63.44 (90.04) | 0.003 | 85.00 (149.90) | 38.74 (32.46) | 0.112 | |
AST (U/L), mean (SD) | 81.02 (145.93) | 66.26 (96.01) | 0.173 | 69.94 (152.27) | 37.78 (25.85) | 0.275 | |
LDH(U/L), mean (SD) | 213.14 (108.26) | 212.49 (95.41) | 0.947 | 203.80 (133.72) | 212.22 (54.91) | 0.792 | |
Total bilirubin (mg/dL), mean (SD) | 24.87 (32.03) | 26.59 (46.35) | 0.487 | 24.32 (26.74) | 27.27 (42.30) | 0.616 | |
Albumin (g/dL), mean (SD) | 38.33 (7.35) | 36.51 (5.78) | 0.001 | 37.54 (4.93) | 38.01 (4.79) | 0.634 | |
PT (second), mean (SD) | 13.61 (1.24) | 14.20 (4.64) | <0.001 | 13.64 (1.24) | 13.51 (0.83) | 0.596 | |
INR | 1.21 (3.91) | 1.12 (0.61) | 0.751 | 1.07 (0.13) | 1.06 (0.08) | 0.6 | |
CA 19-9 (U/mL), median (IQR) | 21.40 (7.50, 105.90) | 335.00 (34.90, 1,620.75) | <0.001 | 20.45 (7.00, 98.10) | 168.70 (24.66, 1,043.63) | 0.003 | |
CEA (μg/L), median (IQR) | 1.70 (1.10, 2.40) | 3.00 (1.90, 9.80) | <0.001 | 1.69 (1.02, 2.41) | 3.05 (2.29, 5.57) | <0.001 | |
AFP (μg/L), median (IQR) | 2.56 (1.90, 3.51) | 2.97 (2.12, 3.78) | 0.002 | 2.40 (1.60, 3.41) | 2.35 (1.81, 3.36) | 0.898 | |
CA 125 (U/mL), mean (SD) | 30.62 (125.99) | 404.82 (1667.48) | 0.011 | 28.58 (139.99) | 122.71 (165.13) | 0.012 | |
Complication | |||||||
HBsAg+ | 149 (7.2%) | 20 (9.9%) | 0.211 | 18 (8.3%) | 1 (3.7%) | 0.646 | |
HBcAb+ | 721 (44.5%) | 66 (45.8%) | 0.821 | 108 (57.8%) | 14 (60.9%) | 0.951 | |
HCV | 2 (0.09%) | 1 (0.49%) | 0.317 | 1 (0.5%) | 0 (0.0%) | 1 | |
Diabetes | 117 (5.7%) | 15 (7.4%) | 0.387 | 16 (7.4%) | 0 (0.0%) | 0.295 | |
Cirrhosis | 220 (9.7%) | 28 (13.86%) | 0.082 | 21 (9.63%) | 4 (14.81%) | 0.059 | |
Fatty liver | 181 (7.84%) | 10 (5.0%) | 0.086 | 13 (5.96%) | 2 (7.41%) | 0.237 |
IHL-IBI, intrahepatic biliary inflammation associated with intrahepatic lithiasis; IHL-ICC, intrahepatic cholangiocarcinoma associated with intrahepatic lithiasis; ALK, alkaline phosphatase; γ-GT,γ-Glutamyl transferase; ALT, alanine aminotransferase; AST, aspartate aminotransferase; LDH, lactic dehydrogenase; PT, prothrombin time; INR, international normalized ratio; CA 19-9, cancer antigen 19-9; CEA, carcinoembryonic antigen; AFP, alpha-fetal protein; CA 125, cancer antigen 125.
ROC curve analysis of cross-sectional liver imaging and laboratory diagnostic tests
Liver imaging
Preoperative imaging with at least one of the liver imaging techniques was performed for each patient with US on 1,566 patients, CT on 1,787 patients, enhanced CT on 293 patients, MRI on 454 patients, and enhanced MRI on 80 patients. As shown in Figure 2A, there are the highest discriminatory capacity of enhanced CT and enhanced MRI, with the AUC (95% CI) of 0.829 (0.781–0.871) and 0.827 (0.723–0.904) respectively, the other imaging techniques including comprehensive imaging diagnosis with AUC (95% CI) of 0.758 (0.737–0.792), US with AUC (95% CI) of 0.667 (0.643–0.690), nonenhanced MRI with AUC (95% CI) of 0.747 (0.704–0.786), and nonenhanced CT with AUC (95% CI) of 0.698 (0.677–0.720). The inter-observer agreement of two radiologists was very good with Kappa-Cohen index 0.956 (95% CI, 0.947 to 0.966).
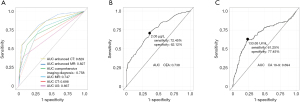
Serum CEA
Preoperative data on serum CEA tests for 157 patients in the IHL-ICC group and 1,188 cases in the IHL-IBI group were obtained and analyzed. The median level of serum CEA in the IHL-ICC patients was 3.0 µg/L, ranging 0.3–2,149.4 µg/L, which was significantly higher than 1.7 µg/L with a range, of 0.29–57.5 µg/L in the IHL-IBI patients (P<0.001). By plotting sensitivity of serum CEA against 1-specificity for each possible cutoff point, the AUC was 0.738. The optimal cutoff value for serum CEA was 2.050 µg/L, which yielded sensitivity of 72.45% and specificity of 62.12% (Figure 2B). The optimal cutoff points of serum CEA were 5 and 10 µg/L by SPSS’s Optimal Binning methods, and then carried out univariate analysis (Table 2). The difference between both groups was marked (P<0.001).
Table 2
Tumor markers | IHL-IBI | IHL-ICC | P value |
---|---|---|---|
CEA (μg/L) | |||
≤5 | 900 (96.6%) | 67 (68.4%) | <0.001 |
6~10 | 30 (3.2%) | 11 (11.2%) | |
>10 | 2 (0.2%) | 20 (20.4%) | |
CA199 (U/mL) | <0.001 | ||
≤145 | 731 (78.4%) | 40 (40.8%) | |
>145 | 201 (21.6%) | 58 (59.2%) |
IHL-IBI, intrahepatic biliary inflammation associated with intrahepatic lithiasis; IHL-ICC, intrahepatic cholangiocarcinoma associated with intrahepatic lithiasis; CA 19-9, cancer antigen 19-9; CEA, carcinoembryonic antigen
Serum CA 19-9
Levels of serum CA 19-9 were preoperatively examined in 160 cases with IHL-ICC and 1,207 patients with IHL-IBI. The median levels of serum CA 19-9 in the IHL-ICC patients (335 U/mL ranging from 0.8–35,587) were significantly greater than 21.4 U/mL (0.6–12,000) for the IHL patients (P<0.001). ROC analysis of predictive accuracy revealed an AUC of 0.694, with an optimal cutoff of 133.65 U/mL, and corresponding sensitivity of 0.612 and specificity of 0.774 (Figure 2C). In order to facilitate the development of a nomogram, the level of serum CA 19-9 was converted to categorical variable according the optimal binning method (<145, and >145 U/mL), and then carried out univariate analysis (Table 2). The difference of the two groups was marked (P<0.001).
Machine learning algorithms for selection of independent ICC predictors
Firstly, we used the LASSO algorithm to select feature variables, and got 27 feature variables (Figure 3). Secondly, 9 feature variables were identified to predict ICC though random forests method (Figure 4). Those of nine were included in the former method of LASSO algorithm.
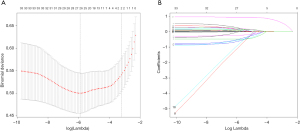
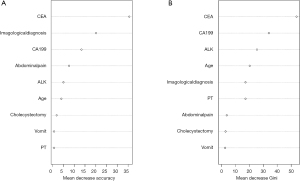
Multivariate logistic regression analysis for selection of independent ICC predictors
Nine candidate predictors, selected from machine learning algorithms, were included in multivariate regression analysis model, and seven independent predictors for ICC development were eventually identified (Table 3). For IHL patients, the serum level of CEA higher than 10.0 µg/L were associated with the highest risk of developing ICC (OR =47; 95% CI, 9.8–79.4), followed by radiological diagnosis as ICC (OR =15.8; 95% CI, 5.4–30.58), the serum level of CA 19-9 higher than 145 µg/L (OR =3.68; 95% CI, 2.1–7.5), serum level of ALK higher than 100 U/L (OR =2.33; 95% CI, 1.2–5.49), and age >60 (OR =1.95; 95% CI, 1.1–3.89). As the protective factors, abdominal pain and vomit decreased the relative risk of developing ICC with the OR of 0.57 (95% CI, 0.2–1.03) and 0.64 (95% CI, 0.3–1.21) in IHL patients.
Table 3
Characteristic | P value | OR | 95% CI |
---|---|---|---|
Age >60 | 0.02 | 1.95 | 1.11–3.89 |
Abdominal pain | 0.12 | 0.57 | 0.29–1.03 |
Vomiting | 0.14 | 0.64 | 0.36–1.21 |
ALK >100 (U/L) | 0.01 | 2.33 | 1.24–5.49 |
CEA (μg/L) | |||
≤5 | |||
6–10 | <0.001 | 3.5 | 1.48–16.72 |
>10 | <0.001 | 47.01 | 9.83–79.46 |
CA 19–9>145 (U/mL) | <0.001 | 3.68 | 2.17–7.5 |
Imagological diagnosis | |||
No mass-related lesion found | |||
Inflammatory mass | 0.01 | 2.7 | 1.32–4.95 |
Suspicious of cancer | <0.001 | 6.93 | 3.78–20.14 |
Cancer | <0.001 | 15.87 | 5.46–30.58 |
IHL-ICC, intrahepatic cholangiocarcinoma associated with intrahepatic lithiasis; ALK, alkaline phosphatase; CA 19-9, cancer antigen 19-9; CEA, carcinoembryonic antigen.
Construction and validation of the nomogram
The nomogram was constructed based upon the seven independent predictors as described above (Figure 5). In the nomogram model, each predictor was ascribed a total point or a weighted point total (top scale), which implied the probability of ICC (bottom scale). The P value for the Hosmer-Lemeshow test was 0.489, indicating good fit of the model. The calibration plot of the nomogram was subsequently developed. As illustrated in Figure 6, the nomogram was well calibrated.
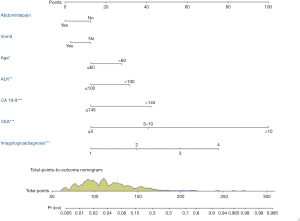
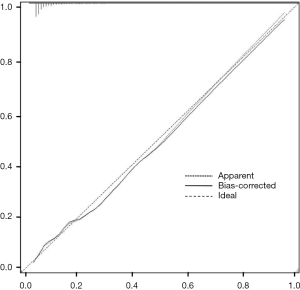
As shown in Figure 7, the AUC of the nomogram was 0.867 (95% CI, 0.845–0.887), with accuracy, sensitivity, specificity, NPV, and PPV of 81.6% (95% CI, 0.792–0.840), 30.5%, 96.6%, 82.6% and 72.4%, respectively. The Youden-derived optimal cut-off value for the nomogram was 0.107, corresponding to the total points of 140. At this threshold, the nomogram had a sensitivity of 72.4%, specificity of 82.4%. If a specificity of 90% was set as the cut-off value, the corresponding probability, total points, and sensitivity were 0.179, 153, and 60.2%, respectively. At the cut-off value for the specificity of 95%, the corresponding probability, total points, and sensitivity were 0.254, 163, and 50.0%, respectively.
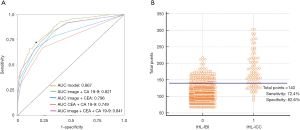
Furthermore, the AUC comparison revealed the highest discriminatory capacity of the nomogram among any combination of the two predictors, which was significantly higher than comprehensive radiological diagnosis in conjunction with CA 19-9 with AUC of 0.821 (P<0.01), comprehensive radiological diagnosis combined CEA with AUC of 0.796 (P<0.01) (Figure 7).
Next, the nomogram was then validated externally in two validation cohorts (Figure 8). The AUC was 0.881 in The Second Affiliated Hospital of WZMC, and 0.938 in The First Affiliated Hospital of FJMU. The AUC of the prediction model based on The First Affiliated Hospital of WZMU is not significantly different from The Second Affiliated Hospital of WZMC (P=0.833). The result of the external validation in The First Affiliated Hospital of FJMU even better than training cohort (P=0.036).
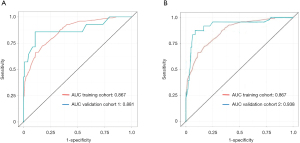
Nomogram performance in individual patients
To display the application of the nomogram, we selected two IHL patients who had received hepatectomy recently in our hospital and were not within the training and validating groups (Figure 9). The clinical features of two IHL patients are showed in Appendix 1. The intrahepatic lesions of them had been detected through enhanced CT, however, which was not confirmed. The predicted results using the nomogram are consistent with the pathology. By postoperative pathology, the former patient was diagnosed as IHL-IBL, and the latter patient was diagnosed as IHL-ICC.
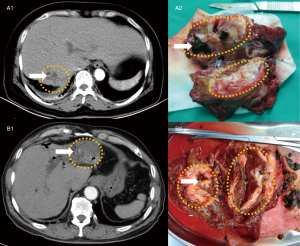
We have made the nomogram into a web page as http://www.bioinformatics.com.cn/calculate_7_factor_nomogram_total_points_for_zeng, and clinicians can get the total point and probability of ICC after inputting the data.
Discussion
The estimation of the individual risk of ICC at diagnosis of IHL is attractive because it can facilitate the decision regarding the surgical treatment at its early stage. The present work is the first attempt to propose a diagnostic nomogram to improve diagnostic accuracy of ICC in the patients with IHL. The nomogram was validated internally and externally in two large data sets.
We developed a nomogram to predict ICC for patients with IHL complicated by imagiologically diagnosed mass in a recent study (26). However, the nomogram is not applicable for all patients with IHL because the patients without radiological diagnosis of mass were not included in the study. The imaging manifestations are not obvious in the early stage of some cancerous lesions. We found 62 cases of IHL-ICC whose neoplastic lesions had not been report by both radiologists in all imaging examinations. Therefore, we developed a novel preoperative prediction model for all patients diagnosed with HIL, to estimate, with good discrimination and calibration, the risk of ICC. The nomogram included preoperative factors of age, abdominal pain, vomiting, comprehensive radiological diagnosis, ALK, CEA, and CA 19-9. The present study on IHL patients with or without ICC had the following main novel findings: (I) age, abdominal pain, vomiting, result of imaging evaluation, ALK, serum CEA, and serum CA 19-9 were potential predictors for IHL-ICC; (II) the optimal cutoff values of serum CEA and CA 19-9 for preoperative diagnosis of ICC among the IHL patients were determined as 2.05 µg/L and 133.65 U/mL, respectively; and (III) the discriminatory ability of the nomogram model was much improved and significantly greater than the image examination in combination with one of other predictors.
In terms of demography and clinical symptoms, age, absence of abdominal pain and absence of vomiting were selected as the risk factors for ICC in the IHL patients. For patients with cholelithiasis, clinical symptoms like abdominal pain and vomiting are mostly associated with biliary inflammation rather than ICC. It suggested that ICC should be especially suspected when the patients with IHL are over 60 years old and lack of clinical symptoms. However, there was no consensus on this aspect. Kim et al. (25) considered that IHL-ICC group differed from IHL-IBI group because of its aged, longer duration of stone history, lower rates of abdominal pain and higher rates of weight loss. Liu et al. (27) also reported there were higher rates of clinical symptoms in IHL-IBI compared with IHL-ICC, while, no significant difference on age. Diabetes, obesity, smoking and alcohol had been reported as risk factors for ICC in USA (28,29), however, these factors were not associated with ICC in China (30) and our study. More and more researches had found HBV and HCV infection to be the risk factors of ICC (8,10,28,30), but in our study and a previous Chinese report (27), this was not supported for patients with IHL.
In cases of IHL-ICC, there are no specific symptoms other than the clinical manifestation of hepatolithiasis. Therefore, detection of ICC in IHL is dependent on imaging modalities, such as US, CT, and MRI. ICC can generally be classified into three types on the basis of gross morphologic features: mass-forming, periductal infiltrating, and intraductal growth (31). For the patients with IHL, inflammatory pseudotumor or liver abscess often occurs at the site of intrahepatic stones, it is difficult to distinguish from mass-forming ICC, whereas proliferative cholangitis or inflammatory stenosis are difficult to distinguish from periductal infiltrating ICC. Furthermore, after long-term chronic inflammation, liver segments often become fibrotic and scarred (11), this makes IHL even more difficult to distinguish from ICC on imaging. In addition, early stage discovery of ICC and intraductal growth type ICC by imaging technology is challenging. Therefore, the preoperative diagnosis for IHL-ICC cannot be solely dependent on imaging technology, whose accuracy is only about 30–60% (7,11,12,25). Because enhanced CT and enhanced MRI examinations were not performed on some of the studied patients, data on enhanced CT and enhanced MRI examination were missing for these patients, and thus overall, the accuracy of comprehensive radiological diagnosis for all selected cases was still less than an enhanced CT or enhanced MRI alone, as demonstrated in Figure 2A. Even using high quality imaging examinations, the preoperative diagnostic coincidence rates of enhanced CT and enhanced MRI were 54.4% (31/57) and 55.6% (15/27) among the IHL-ICC enrolled in this study. The accuracy of preoperative radiological diagnosis may be unsatisfactory in the decision making for surgeons.
Tumor markers in serum are not specific for ICC but may be of diagnostic value. As also demonstrated in the present study, serum tumor markers, such as CEA and CA 19-9, can be of help in detecting IHL-ICC. Because radiological examination alone was limited for accurate diagnosis of IHL-ICC, it is necessary to combine the serum tumor markers to improve the diagnostic accuracy. For discriminating between IHL-ICC and IHL-IBI, the present study showed that the optimal cutoff value for serum CEA was 2.050 µg/L with a sensitivity of 72.24% and a specificity of 62.14%. The ideal CEA cut-off value for our cohort was significantly lower than 5.0 µg/L [as the standard CEA cut-off value adapted at most of institutions (32)] and that also reported by Kim et al. (4.2 µg/L) (25). The result suggests that we should be alert to concurrent cholangiocarcinoma when serum CEA is higher than 2.050 µg/L in IHL patients. Furthermore, the serum CEA holds the highest weight as one of predictive factors for IHL-ICC in the present nomogram. It also suggests that we need to pay great attention to serum CEA of IHL patients. This result is similar to Loosen et al.’s research (33), which considered that the ideal CEA cut-off value (1.55 µg/L) established to differentiate between cholangiocarcinoma patients and healthy controls was distinctly lower than the standard cut-off value (5 µg/L) and also when comparing between cholangiocarcinoma patients and primary sclerosing cholangitis (PSC) patients the cut-off value (1.85 µg/L) was only slightly higher. The optimal cutoff value for serum CA 19-9 was 133.65 U/mL with a sensitivity of 61.2% and a specificity of 77.4% in our cohort. It is the first time that the ideal CA 19-9 cut-off value is provided for predicting ICC in IHL patients. Some previous studies have noted that preoperative CA 19-9 values greater than standard cut-off value (37 U/mL) can distinguish ICC from benign bile duct lesions and PSC (33,34), however, there is no specific study on IHL patients. It has been reported that the serum CA 19-9 level can be elevated when the bile duct is obstructed or inflamed and free from carcinoma (35). Therefore, the cutoff value of serum CA 19-9 of 37 U/mL is inapplicable to predict IHL-ICC. On the other hands, it should also be noted that the possibility of ICC cannot be excluded when the value of serum CA 19-9 is normal. This is because about seven percent of the populations are Lewis negative and unable to express CA 19-9 (36,37).
To our knowledge, this is the first study was designed to create and validate a diagnostic nomogram for predicting IHL-ICC on the largest sample size of IHL until now. By analyzing the demography, clinical, laboratory, and imaging data from 2,269 patients with IHL, we developed a nomogram that performed well in predicting IHL-ICC. The nomogram was proven to be of good fitness through the method of calibration plot with bootstrap sampling for internal validation and applied in other two hospitals for external validation by ROC. The concise and well fitted nomogram contains the variables of age, abdominal pain, vomiting, comprehensive radiological diagnosis, ALK, CEA, and CA 19-9. Using the nomogram, the AUC for all variables in predicting IHL-ICC was 0.867 (95% CI, 0.845–0.887). The performance of the nomogram is superior to prediction model of any single risk factor or combination of risk factors (Figures 2,7).
The recent advent of machine learning approaches has shown promise to achieve superior prediction ability in various disease conditions compared to the traditional approaches (38,39). In this analysis of the largest sample size of data on IHL patients underwent hepatectomy or biopsy, the use of machine learning approaches (i.e., Lasso regression, random forest) significantly improved the accuracy for screening risk factors over the traditional approach. In addition, the nomogram had been considered as an important component of modern medical decision making, especially in oncology (40,41). Our study has backed up its diagnostic value for patients with IHL. With the probability of ICC of 0.101 corresponding to the total points of 140 through the Youden-derived cut-off value in the present nomogram, the sum of the sensitivity (72.4%) and specificity (82.4%) for ICC diagnosis reaches the maximum. Therefore, ICC can be considered for the patients with total points greater than 140, and surgical intervention is highly recommended. Whereas, for the patients with the total points less than 140 points, close observation and follow-up should be made, during which the dynamic results of serum CA 19-9, CEA, CT imaging, and the nomogram total points should be checked carefully. Furthermore, if patients have biliary tract infection or liver abscess, physicians should check up the imaging changes following antibiotic treatment. If the specificity of 82.4% is not satisfactory, a specificity of 90% or 95% as cut-off value, corresponding total points of 153 and sensitivity of 60.2%, or 163 and 50.0% can be used (Appendix 2).
As a noninvasive method, the nomogram would be a convenient application for clinicians. Because of the high incidence of ICC in patients with IHL, we recommend the clinical management algorithm (Figure 10) to treat patients with IHL based on our clinical experience and the nomogram in this study. In order to facilitate practical application, we classify the points of nomogram into three groups according to the optimal cutoff point: low-, medium- and high-risk group. The total points, probability of ICC, sensitivity and specificity are listed as follow: <100, <0.02, >0.969 and <0.364 in low risk group; 100–170, 0.02–0.3, 0.969–0.439 and 0.3640.971 in medium-risk group; >170, >0.3, <0.439 and >0.971 in high-risk group. Using the process flow, unnecessary surgical treatment can be avoided for IHL patients. Meanwhile, ICC patients also will not be missed. A treatment flow chart was advised for both symptomatic and asymptomatic hepatolithiasis from the Guidelines Committee for the Japanese Society of Gastroenterology (42). They also believed that identifying ICC was a very important part of the process of treating IHL. However, the committee and previous research did not propose any quantitative risk assessment methods to identify ICC from IHL patients.
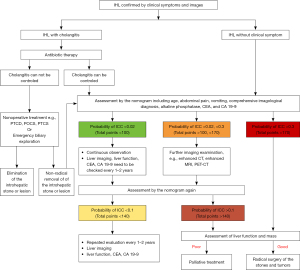
There are still some limitations in this study. Firstly, the nomogram was developed and validated in Eastern populations. However, IHL is quite uncommon in Western countries. It might be inaccurate for western patients. Secondly, the study design was retrospective, and missing data in demographic and clinical factors existed. To solve those problems, prospective and global studies with high quality imaging are urgently needed in the future.
In conclusion, age, abdominal pain, vomiting, comprehensive radiological diagnosis, ALK, CEA, and CA 19-9 are the potential independent factors for IHL-ICC. The optimal cutoff values of serum CEA and CA 19-9 are 2.05 µg/L and 133.65 U/mL, respectively. The nomogram holds promise as a novel and accurate tool to identify ICC in IHL patients for hepatectomy, and to differentiate from benign occupying lesions to avoid unnecessary surgical resection.
Acknowledgments
Funding: None.
Footnote
Reporting Checklist: The authors have completed the TRIPOD reporting checklist. Available at https://hbsn.amegroups.org/article/view/10.21037/hbsn-20-332/rc
Data Sharing Statement: Available at https://hbsn.amegroups.org/article/view/10.21037/hbsn-20-332/dss
Conflicts of Interest: All authors have completed the ICMJE uniform disclosure form (available at https://hbsn.amegroups.org/article/view/10.21037/hbsn-20-332/coif). The authors have no conflicts of interest to declare.
Ethical Statement: The authors are accountable for all aspects of the work in ensuring that questions related to the accuracy or integrity of any part of the work are appropriately investigated and resolved. The study was conducted in accordance with the Declaration of Helsinki (as revised in 2013). This retrospective study was reviewed and approved by the Institutional Review Board (IRB) of The First Affiliated Hospital of Wenzhou Medical University (No. WZhosp1-2000-1). All procedures performed in studies involving human participants were in accordance with the ethical standards of IRB, and a waiver of written informed consent was granted by the IRB due to the retrospective nature of this study in which de-identified data were used and analyzed.
Open Access Statement: This is an Open Access article distributed in accordance with the Creative Commons Attribution-NonCommercial-NoDerivs 4.0 International License (CC BY-NC-ND 4.0), which permits the non-commercial replication and distribution of the article with the strict proviso that no changes or edits are made and the original work is properly cited (including links to both the formal publication through the relevant DOI and the license). See: https://creativecommons.org/licenses/by-nc-nd/4.0/.
References
- Bridgewater J, Galle PR, Khan SA, et al. Guidelines for the diagnosis and management of intrahepatic cholangiocarcinoma. J Hepatol 2014;60:1268-89.
- Yoh T, Hatano E, Nishio T, et al. Significant Improvement in Outcomes of Patients with Intrahepatic Cholangiocarcinoma after Surgery. World J Surg 2016;40:2229-36.
- Zhang H, Yang T, Wu M, et al. Intrahepatic cholangiocarcinoma: Epidemiology, risk factors, diagnosis and surgical management. Cancer Lett 2016;379:198-205.
- Doherty B, Nambudiri VE, Palmer WC. Update on the Diagnosis and Treatment of Cholangiocarcinoma. Curr Gastroenterol Rep 2017;19:2.
- Patel T. Increasing incidence and mortality of primary intrahepatic cholangiocarcinoma in the United States. Hepatology 2001;33:1353-7.
- Patel T. Worldwide trends in mortality from biliary tract malignancies. BMC Cancer 2002;2:10.
- Guglielmi A, Ruzzenente A, Valdegamberi A, et al. Hepatolithiasis-associated cholangiocarcinoma: results from a multi-institutional national database on a case series of 23 patients. Eur J Surg Oncol 2014;40:567-75.
- Lee TY, Lee SS, Jung SW, et al. Hepatitis B virus infection and intrahepatic cholangiocarcinoma in Korea: a case-control study. Am J Gastroenterol 2008;103:1716-20.
- Zhou YM, Yin ZF, Yang JM, et al. Risk factors for intrahepatic cholangiocarcinoma: a case-control study in China. World J Gastroenterol 2008;14:632-5.
- Donato F, Gelatti U, Tagger A, et al. Intrahepatic cholangiocarcinoma and hepatitis C and B virus infection, alcohol intake, and hepatolithiasis: a case-control study in Italy. Cancer Causes Control 2001;12:959-64.
- Kubo S, Kinoshita H, Hirohashi K, et al. Hepatolithiasis associated with cholangiocarcinoma. World J Surg 1995;19:637-41.
- Uenishi T, Hamba H, Takemura S, et al. Outcomes of hepatic resection for hepatolithiasis. Am J Surg 2009;198:199-202.
- Lin CC, Lin PY, Chen YL. Comparison of concomitant and subsequent cholangiocarcinomas associated with hepatolithiasis: Clinical implications. World J Gastroenterol 2013;19:375-80.
- Kim HJ, Kim JS, Suh SJ, et al. Cholangiocarcinoma Risk as Long-term Outcome After Hepatic Resection in the Hepatolithiasis Patients. World J Surg 2015;39:1537-42.
- Suzuki Y, Mori T, Yokoyama M, et al. Hepatolithiasis: analysis of Japanese nationwide surveys over a period of 40 years. J Hepatobiliary Pancreat Sci 2014;21:617-22.
- Chen MF, Jan YY, Wang CS, et al. Intrahepatic stones associated with cholangiocarcinoma. Am J Gastroenterol 1989;84:391-5.
- Su CH, Shyr YM, Lui WY, et al. Hepatolithiasis associated with cholangiocarcinoma. Br J Surg 1997;84:969-73.
- Chen MF. Peripheral cholangiocarcinoma (cholangiocellular carcinoma): clinical features, diagnosis and treatment. J Gastroenterol Hepatol 1999;14:1144-9.
- Chen MF, Jan YY, Jeng LB, et al. Intrahepatic cholangiocarcinoma in Taiwan. J Hepatobiliary Pancreat Surg 1999;6:136-41.
- Lesurtel M, Regimbeau JM, Farges O, et al. Intrahepatic cholangiocarcinoma and hepatolithiasis: an unusual association in Western countries. Eur J Gastroenterol Hepatol 2002;14:1025-7.
- Khan SA, Thomas HC, Davidson BR, Taylor-Robinson SD. Cholangiocarcinoma. Lancet 2005;366:1303-14.
- Tyson GL, El-Serag HB. Risk factors for cholangiocarcinoma. Hepatology 2011;54:173-84.
- Nakanuma Y, Terada T, Tanaka Y, et al. Are hepatolithiasis and cholangiocarcinoma aetiologically related? A morphological study of 12 cases of hepatolithiasis associated with cholangiocarcinoma. Virchows Arch A Pathol Anat Histopathol 1985;406:45-58.
- Weber SM, Ribero D, O'Reilly EM, et al. Intrahepatic cholangiocarcinoma: expert consensus statement. HPB (Oxford) 2015;17:669-80.
- Kim YT, Byun JS, Kim J, et al. Factors predicting concurrent cholangiocarcinomas associated with hepatolithiasis. Hepatogastroenterology 2003;50:8-12.
- Chen G, Yu H, Wang Y, et al. A novel nomogram for the prediction of intrahepatic cholangiocarcinoma in patients with intrahepatic lithiasis complicated by imagiologically diagnosed mass. Cancer Manag Res 2018;10:847-56.
- Liu ZY, Zhou YM, Shi LH, et al. Risk factors of intrahepatic cholangiocarcinoma in patients with hepatolithiasis: a case-control study. Hepatobiliary Pancreat Dis Int 2011;10:626-31.
- Petrick JL, Yang B, Altekruse SF, et al. Risk factors for intrahepatic and extrahepatic cholangiocarcinoma in the United States: A population-based study in SEER-Medicare. PLoS One 2017;12:e0186643
- Welzel TM, Graubard BI, El-Serag HB, et al. Risk factors for intrahepatic and extrahepatic cholangiocarcinoma in the United States: a population-based case-control study. Clin Gastroenterol Hepatol 2007;5:1221-8.
- Tao LY, He XD, Qu Q, et al. Risk factors for intrahepatic and extrahepatic cholangiocarcinoma: a case-control study in China. Liver Int 2010;30:215-21.
- Yamasaki S. Intrahepatic cholangiocarcinoma: macroscopic type and stage classification. J Hepatobiliary Pancreat Surg 2003;10:288-91.
- Burtis CA, Bruns DE. Tietz fundamentals of clinical chemistry and molecular diagnostics. Saunders: Elsevier Health Sciences, 2014:351.
- Loosen SH, Roderburg C, Kauertz KL, et al. CEA but not CA19-9 is an independent prognostic factor in patients undergoing resection of cholangiocarcinoma. Sci Rep 2017;7:16975.
- Liang B, Zhong L, He Q, et al. Diagnostic Accuracy of Serum CA19-9 in Patients with Cholangiocarcinoma: A Systematic Review and Meta-Analysis. Med Sci Monit 2015;21:3555-63.
- Albert MB, Steinberg WM, Henry JP. Elevated serum levels of tumor marker CA19-9 in acute cholangitis. Dig Dis Sci 1988;33:1223-5.
- Alvaro D. Serum and bile biomarkers for cholangiocarcinoma. Curr Opin Gastroenterol 2009;25:279-84.
- Parra-Robert M, Santos VM, Canis SM, et al. Relationship Between CA 19.9 and the Lewis Phenotype: Options to Improve Diagnostic Efficiency. Anticancer Res 2018;38:5883-8.
- Goto T, Camargo CA, Faridi MK, et al. Machine learning approaches for predicting disposition of asthma and COPD exacerbations in the ED. Am J Emerg Med 2018;36:1650-4.
- Guo BL, Ouyang FS, Yang SM, et al. Development of a preprocedure nomogram for predicting contrast-induced acute kidney injury after coronary angiography or percutaneous coronary intervention. Oncotarget 2017;8:75087-93.
- Balachandran VP, Gonen M, Smith JJ, DeMatteo RP. Nomograms in oncology: more than meets the eye. Lancet Oncol 2015;16:e173-80.
- Wang Y, Li J, Xia Y, et al. Prognostic nomogram for intrahepatic cholangiocarcinoma after partial hepatectomy. J Clin Oncol 2013;31:1188-95.
- Tazuma S, Unno M, Igarashi Y, et al. Evidence-based clinical practice guidelines for cholelithiasis 2016. J Gastroenterol 2017;52:276-300.