Prognostic risk scores for liver transplantation: game changers or statistical artworks?
Several prognostic liver transplant-related risk scores for hepatocellular carcinoma (HCC) have been developed in recent years. The most prominent scores, displayed in Table 1, are based exclusively on HCC-related variables (1-6). Given that non-HCC-related variables can also influence post-transplant outcomes, Goldberg et al. sought to develop a continuous risk score predicting post-transplant survival for patients using both HCC- and non-HCC-related variables (1). Their LiTES-HCC score, recently published in the Journal of Hepatology, was developed by analyzing national registry (OPTN/UNOS) data of 6,502 adult HCC patients that received a deceased-donor liver transplant. The score comprised eleven variables and two interaction terms (Table 1) and showed similar performance to the Metroticket and HALT-HCC at 5- and 10-years post-transplant.
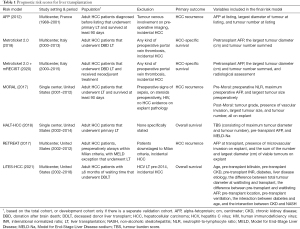
Full table
Although this well-designed study shows the LiTES-HCC score can estimate post-transplant survival quite well, the development of yet another prognostic post-transplant score calls into question the utility of such algorithms: in what capacity can they be used to augment clinical decision-making?
To address this question, the core principles of liver transplantation should be outlined. Generally, liver transplantation should offer equal or superior survival outcomes to any other curative-intent treatment. However, given the scarcity of suitable grafts, listing does not guarantee receipt of a life-saving transplant. Hence, decisions must be made to select only those whom will benefit most from liver transplantation (2). For patients with end-stage liver disease, treatment options short of liver transplantation are limited, justifying the risk of becoming ineligible for transplant while waiting. The decision of transplantation in HCC patients is more complex, given that selection must be based on not only expected transplant-related survival but also cancer recurrence and how such oncologic outcomes compare to alternative treatment options (2,3). This complexity gap can potentially be bridged by risk models, guiding patient selection and prioritization. Nonetheless, before these models can be applied to clinical practice, several methodological concerns must be considered.
Each scientific study is designed with a particular hypothesis and aim, specifying the target population and outcome of interest. This clarifies to whom and in what setting the study inferences apply. However, in the absence of clear inclusion/exclusion criteria, inferences may be incorrectly extrapolated to larger populations than targeted by the study. For example, the LiTES-HCC score’s study included waitlisted HCC patients, but not those with a waiting time of <6 months (1). Though the rationale for exclusion is described, it presents critical information about applicability. Inclusion/exclusion criteria aid in making study results more relevant and clinically applicable. However, if overabundant or erroneously applied, inferences may only apply to a superselected population with limited to no external and real-life applicability. Similarly, due to healthcare-related changes and modifications to organ allocation policies, studies spanning a lengthy period or using outdated data may reduce both model performance and clinical applicability.
The study’s outcome of interest dictates the stage of patient care where the results may offer clinical guidance. For the LiTES-HCC score, post-transplant survival was chosen, highlighting that the outcome prediction only applies to patients who are fortunate to be allocated a liver graft. This omits patients who drop out of the waitlist due to tumour progression, deterioration, or death—occurring in 20–30% of waitlisted HCC patients (1,4,5). Studying patients’ outcomes after transplantation, the intended treatment, is referred to as a per-protocol analysis (6). Accordingly, the prognostic information from such a model only applies to HCC patients who are able to receive a transplant. Therefore, if the risk score is used to select or prioritize patients, the expected benefit of treatment initiation (here, a favorable survival) may be overestimated as the score assumes a patient will survive long enough to receive a transplant. Whereas in reality, some patients will die or experience tumour progression outside of transplantable criteria before being able to benefit from a transplant and drop out from the waitlist. It is conceivable that another treatment may have prolonged their survival, albeit less than a successful transplantation. Such alternative treatments have no associated waitlist mortality and can be performed shortly after treatment decision (e.g., liver resection). Consequently, the estimated average survival of an alternative treatment is guaranteed. A risk score that can compare expected outcomes of liver transplantation with alternative treatments would require an intention-to-treat analysis. In contrast to the per-protocol analysis, the outcome is not only based on a guaranteed receipt of the originally allocated treatment but also considers waitlist dropouts, a scenario that is unfortunately relatively common (6). Meaning, if a patient has a LiTES-HCC score predicted 5-year post-transplant survival of 100%, but a 50% chance of waitlist dropout before transplant receipt, the intention-to-treat analysis would take this into account and would predict that the estimated average 5-year survival rate after liver transplant listing would be closer to 50%. Then, the benefit of an alternative treatment with an estimated 5-year survival of 70% could outweigh that of listing for a liver transplant. When considering tumour recurrence as the outcome of interest, a per-protocol analysis is obligatory, as recurrence cannot occur before a treatment has been applied. This, in turn, automatically excludes the use of the risk model as a pre-transplant selection tool. In conclusion, if one wants to use a transplant-related prognostic risk score for liver transplant eligibility or allocation purposes and to compare the estimated outcomes with alternative treatments, an intention-to-treat survival analysis should be applied. If interested in post-treatment outcomes such as recurrence or the effectual treatment-related survival, a per-protocol analysis will suffice, with the understanding that the model is precluded from serving as a decision tool for determining transplant eligibility or allocating grafts. Figure 1 illustrates the models mentioned above and how they, based on their study design, can be applied in a clinical setting (1,7-12).
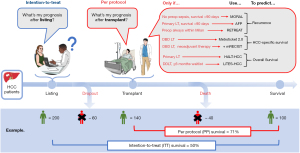
The framework of a risk model will ultimately consist of several predefined variables. Consequently, the rationale for selecting such variables is crucial. For transplantation, variables can be collected at listing, during listing, at transplant/waitlist dropout, per-operatively, and post-operatively. Only those timepoints most relevant to the outcome of interest should be considered. Meaning, if one wants to predict post-transplant survival, variables at all time points up to and including the transplantation itself, the starting point of measuring the outcome of interest, can be collected. However, suppose the aim is to predict survival rates for all patients listed for transplantation. In that case, the starting point of the outcome of interest will be listing and should only include variables at listing. The variables collected at these different time points should be literature-based or informed by clinical experience, and associated with the outcome of interest. Importantly, the total set of variables chosen may ultimately determine the model’s external utility since center/region-specific variables may be unavailable at other centers. More specific variables, the more powerful but also less generalizable the results may become, highlighting the potential for overfitting. On the other hand, less specific variables, the less robust but more generalizable the results may become, emphasizing model parsimony.
Validation is obligatory in determining any prediction model’s performance. After all, a risk score is expected to perform best in the dataset on which it was developed. In developing a model, internal validation is performed, typically through split-sample, cross-validation, or bootstrapping. Though this evaluates the model’s performance on data not used to train/develop the model, it does not supplant the need for external validation, as the validation cohort often bears high resemblance to the development cohort, potentially leading to overly optimistic performance. Moreover, the model’s transportability and performance in other similar settings remain to be clarified (13). Therefore, the need for external validation should be stressed in any prediction model’s development.
Though the models in Figure 1 have resulted in furthering our understanding of HCC, none can be used to determine transplant eligibility, allocation prioritization, or to compare their oncologic outcomes with risk models for alternative HCC treatments (1,7-12). Therefore, to increase the relevance of future liver transplant-related survival risk scores, we would like to suggest a more meaningful standardized study design where the analysis is based on the intention-to-treat principle, the variables analyzed are available at the moment of listing, and with an obligatory external validation. This will allow maximizing the models’ predictive performance, improve clinical applicability, and enable direct model comparison to allow future refinement.
In conclusion, we read with interest the study of Goldberg et al. and want to congratulate the authors for a well-conducted study providing a continuous risk score for predicting post-transplant survival. Concurrently, we wonder if an intention-to-treat survival analysis with variables (both HCC- and non-HCC-related) at the time of listing would have resulted in a more clinically meaningful and impactful risk score. After all, the current risk score is unsuitable for prioritizing waitlisted patients and only informative for patients already transplanted. Consequently, it risks resulting as an ingenious statistical quality injection to the contemporary literature without offering much in terms of clinical use.
Acknowledgments
Funding: None.
Footnote
Provenance and Peer Review: This article was commissioned by the editorial office of Hepatobiliary Surgery and Nutrition. The article did not undergo external peer review.
Conflicts of Interest: All authors have completed the ICMJE uniform disclosure form (available at https://hbsn.amegroups.org/article/view/10.21037/hbsn-21-258/coif). The authors have no conflicts of interest to declare.
Ethical Statement: The authors are accountable for all aspects of the work in ensuring that questions related to the accuracy or integrity of any part of the work are appropriately investigated and resolved.
Open Access Statement: This is an Open Access article distributed in accordance with the Creative Commons Attribution-NonCommercial-NoDerivs 4.0 International License (CC BY-NC-ND 4.0), which permits the non-commercial replication and distribution of the article with the strict proviso that no changes or edits are made and the original work is properly cited (including links to both the formal publication through the relevant DOI and the license). See: https://creativecommons.org/licenses/by-nc-nd/4.0/.
References
- Goldberg D, Mantero A, Newcomb C, et al. Predicting survival after liver transplantation in patients with hepatocellular carcinoma using the LiTES-HCC score. J Hepatol 2021;74:1398-406. [Crossref] [PubMed]
- Cillo U, Vitale A, Volk ML, et al. Liver Transplantation for T2 Hepatocellular Carcinoma during the COVID-19 Pandemic: A Novel Model Balancing Individual Benefit against Healthcare Resources. Cancers (Basel) 2021;13:1416. [Crossref] [PubMed]
- Llovet JM, Kelley RK, Villanueva A, et al. Hepatocellular carcinoma. Nat Rev Dis Primers 2021;7:6. [Crossref] [PubMed]
- Mehta N, Dodge JL, Hirose R, et al. Increasing Liver Transplantation Wait-List Dropout for Hepatocellular Carcinoma With Widening Geographical Disparities: Implications for Organ Allocation. Liver Transpl 2018;24:1346-56. [Crossref] [PubMed]
- Yang JD, Larson JJ, Watt KD, et al. Hepatocellular Carcinoma Is the Most Common Indication for Liver Transplantation and Placement on the Waitlist in the United States. Clin Gastroenterol Hepatol 2017;15:767-775.e3. [Crossref] [PubMed]
- Ranganathan P, Pramesh CS, Aggarwal R. Common pitfalls in statistical analysis: Intention-to-treat versus per-protocol analysis. Perspect Clin Res 2016;7:144-6. [Crossref] [PubMed]
- Halazun KJ, Najjar M, Abdelmessih RM, et al. Recurrence After Liver Transplantation for Hepatocellular Carcinoma: A New MORAL to the Story. Ann Surg 2017;265:557-64. [Crossref] [PubMed]
- Sasaki K, Firl DJ, Hashimoto K, et al. Development and validation of the HALT-HCC score to predict mortality in liver transplant recipients with hepatocellular carcinoma: a retrospective cohort analysis. Lancet Gastroenterol Hepatol 2017;2:595-603. [Crossref] [PubMed]
- Mazzaferro V, Sposito C, Zhou J, et al. Metroticket 2.0 Model for Analysis of Competing Risks of Death After Liver Transplantation for Hepatocellular Carcinoma. Gastroenterology 2018;154:128-39. [Crossref] [PubMed]
- Cucchetti A, Serenari M, Sposito C, et al. Including mRECIST in the Metroticket 2.0 criteria improves prediction of hepatocellular carcinoma-related death after liver transplant. J Hepatol 2020;73:342-8. [Crossref] [PubMed]
- Duvoux C, Roudot-Thoraval F, Decaens T, et al. Liver transplantation for hepatocellular carcinoma: a model including α-fetoprotein improves the performance of Milan criteria. Gastroenterology 2012;143:986-94.e3; quiz e14-5. [Crossref] [PubMed]
- Mehta N, Heimbach J, Harnois DM, et al. Validation of a Risk Estimation of Tumor Recurrence After Transplant (RETREAT) Score for Hepatocellular Carcinoma Recurrence After Liver Transplant. JAMA Oncol 2017;3:493-500. [Crossref] [PubMed]
- Ramspek CL, Jager KJ, Dekker FW, et al. External validation of prognostic models: what, why, how, when and where? Clin Kidney J 2020;14:49-58. [Crossref] [PubMed]