A novel clinical model for risk prediction and stratification of new-onset diabetes mellitus after distal pancreatectomy
Highlight box
Key findings
• A novel clinical model for risk prediction and stratification of new-onset diabetes mellitus (NODM) in the patients with benign or low-grade malignant pancreatic lesions after distal pancreatectomy was developed and validated.
What is known and what is new?
• The incidence rate of NODM after distal pancreatectomy remains high.
• The nomogram integrated by age, body mass index, length of pancreatic resection, intraoperative blood loss, and concomitant splenectomy can predict NODM after distal pancreatectomy.
• The nomogram has satisfactory accuracy, reliability and utility in predicting NODM after distal pancreatectomy and risk stratification.
What is the implication, and what should change now?
• The nomogram can assist physicians in clinical decision making by enhancing interventions for high-risk patients and reducing unnecessary testing and treatment costs for low-risk patients.
Introduction
Diabetes mellitus (DM) is a metabolic disease characterized by persistent hyperglycemia if untreated (1). Pancreatogenic DM, or type 3c DM (T3cDM), is caused by exocrine and endocrine dysfunctions due to pancreatic diseases or pancreatic resection. It accounts for approximately 1–9% of all DM cases (2,3). Decreased insulin, glucagon, and pancreatic polypeptide serum levels can lead to fluctuation in the blood glucose levels of these patients (4). Thus, hypoglycemia is more common in T3cDM, referred to as ‘brittle diabetes’, and requires strict insulin administration (5-7).
The development of DM after pancreatectomy may be related to the surgical location and the residual pancreatic volume (8). A systematic review and meta-analysis by De Bruijn et al. indicated that the average cumulative incidence of new-onset diabetes mellitus (NODM) after distal pancreatectomy (DP) was 14% for benign or potentially malignant lesions in 1,731 patients, while another systematic review and meta-analysis by Yu et al. suggested that the NODM rate after DP for pancreatic neoplasms was 23% in 2,356 patients (9,10). Patients receiving DP are more likely to develop NODM than those undergoing pancreaticoduodenectomy, which may be related to the asymmetric distribution of β-islet cells (11,12). Islet cells are spread throughout the pancreas, and their density gradually increases from the head to the body and tail of the pancreas. In contrast, pancreatic polypeptide-secreting PP cells and glucagon-secreting α cells are mainly located in the head and the tail of the pancreas, respectively (13-15). The high prevalence of diabetes after DP necessitates more attention.
Nevertheless, the incidence and risk factors for NODM after DP are diverse and elusive. In addition, most patients do not receive adequate long-term follow-up and blood glucose monitoring, resulting in postoperative blood glucose abnormalities, which remain unidentified until the occurrence of diabetic complications, which is of great concern. Therefore, to ensure that postoperative NODM could be noticed in time, we conducted a follow-up survey. The post-DP patients with pathologically diagnosed pancreatic benign and low-grade malignant lesions (PBLML) were selected as the study population. A prediction model was constructed with identified relevant risk predictors to screen the candidates for postoperative follow-up. Although previous studies have constructed prediction models for NODM after pancreatectomy (16,17), our study is the first to predict NODM in patients with PBLML after DP from multiple centers in the form of the nomogram. The findings are expected to provide clinicians with guidance for surgical planning and early postoperative hyperglycemic control. We present this article in accordance with the TRIPOD reporting checklist (available at https://hbsn.amegroups.com/article/view/10.21037/hbsn-22-382/rc).
Methods
Patient selection and data collection
The study enrolled patients with PBLML (including chronic pancreatitis, serous or mucinous cystic tumors, intraductal papillary mucinous tumors, solid pseudopapillary tumors, neuroendocrine tumors, and so on) who have received DP at Peking Union Medical College Hospital (PUMCH) and Guangdong Provincial People’s Hospital (GDPH) in recent years. Each patient signed informed consent before the surgery and the follow-up survey. The surgical operations were successfully performed and completed by experienced senior surgeons. The study was conducted in accordance with the Declaration of Helsinki (as revised in 2013). Ethical approval of this study was obtained from the Medical Research Ethics Committee of Guangdong Provincial People’s Hospital (No. GDREC2018305H).
The clinical data of these patients, including demographic features [age, gender, family history of DM in first-degree relatives, American Society of Anesthesiologists (ASA) score, and body mass index (BMI)], pathological information (tumor size, tumor component, length of the resected pancreas, and pathological diagnosis), and surgical parameters (surgical approach, operative time, estimated intraoperative blood loss, concomitant splenectomy, and postoperative complications, especially postoperative pancreatic fistula), were retrieved and acquired from the electronic medical record systems of the hospitals. Patients with preoperative DM and pancreatic malignancy or without complete clinicopathological data were excluded.
Postoperative long-term follow-up of the participants was conducted through outpatient clinics, telephone and internet consultations. The primary endpoint of the study was NODM after DP. According to the diagnostic criteria of the American Diabetes Association, these patients were classified into two groups based on the final known blood glucose levels. One group was diagnosed with DM, and the other group was diagnosed with non-diabetes or pre-diabetes [impaired glucose tolerance (IGT) or impaired fasting glucose (IFG)].
Development and validation of nomogram
The PUMCH and GDPH cohorts served as the training and validation cohorts, respectively. According to the sample size requirements for Cox regression analysis, the number of NODM should be more than 10 times the number of predictors included in the prediction model. The overall incidence rate and the cumulative incidence rate of NODM at 1, 3, and 5 years were calculated, and the cumulative risk curves were plotted for two cohorts. A subgroup analysis for the incidence of NODM was performed based on the patients’ pathological diagnoses.
In the training cohort, univariate Cox regression and least absolute shrinkage and selection operator (LASSO) analyses were performed to identify risk factors significantly associated with NODM after DP. Stepwise multivariate Cox regression analysis was applied to construct a prediction model with the optimal goodness of fit according to the minimum value of Akaike Information Criterion (AIC). Based on the results of stepwise Cox regression analysis, the nomogram was plotted to illustrate the prediction model via rms package in R.
The area under the receiver operating characteristic (ROC) curves (AUC) was used to compare and demonstrate the prediction accuracy and discriminatory ability of the nomogram and its components. Calibration curves were plotted to assess the agreement between the nomogram-predicted and actual non-incidence rates of NODM at 1, 3, and 5 years. Decision curve analysis (DCA) was utilized to evaluate the clinical utility of the nomogram for assessing clinical benefits for these patients at 1, 3, and 5 years. Data from the validation cohort were used as an external validation of the nomogram. Risk scores were calculated as predictors based on the nomogram in the validation cohort. The concordance index (C-index) in the validation cohort was calculated. Calibration curves and DCA at 1, 3, and 5 years were plotted for the validation cohort described above. Finally, maximally selected rank statistics were computed using R package, maxstat, to select the optimal cut-off value of risk scores for the classification of the patients in the training cohort. The cumulative probability curves for high- and low-risk groups in the training and validation cohorts were plotted.
Statistical analysis
Continuous variables were presented as mean ± standard deviation, and categorical variables as frequency and percentage. Wilcoxon rank-sum test or Chi-squared test was used to compare and analyze the risk factors of patients with and without NODM according to the data distribution. Figure illustration and statistical analyses were performed using IBM SPSS Statistics version 25, X-tile software version 3.6.1, GraphPad Prism version 7.0, and R version 4.1.0. A P value <0.05 was considered statistically significant.
Results
Characteristics of training and validation cohorts
After long-term follow-up, the study enrolled 448 patients who underwent DP between January 6th, 2004, and January 14th, 2016, at PUMCH and 178 patients who underwent DP between July 29th, 2010, and May 26th, 2022, at GDPH. Follow-up for the PUMCH cohort ended in June 2020, while follow-up for the GDPH cohort ended in October 2022; 108 patients (108/448, 24.1%) in the PUMCH cohort, and 37 patients (37/178, 20.8%) in the GDPH cohort developed postoperative NODM, respectively. The median follow-up time in the PUMCH cohort was 42.8 months [95% confidence interval (CI): 36.9–48.7 months], while in the GDPH cohort, it was 42.9 months (95% CI: 34.5–51.4 months). The cumulative risk curves for NODM of the two cohorts were shown in Figure 1, whereby cumulative incidence increased over time. The 1-, 3- and 5-year cumulative incidence rates of postoperative NODM in the PUMCH cohort were 13.7%, 23.7%, and 29.1%, respectively; correspondingly, these were 9.3%, 15.7%, and 22.1% in the GDPH cohort, respectively. The highest incidence of postoperative NODM in the PUMCH cohort was found in chronic pancreatitis (41.67%), while the highest incidence of postoperative NODM in the GDPH cohort was observed in intraductal papillary mucinous neoplasm (62.5%) (Table 1).
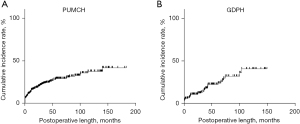
Table 1
Pathological diagnosis | Incidence of NODM after DP (PUMCH), % | Incidence of NODM after DP (GDPH), % |
---|---|---|
Serous cystic neoplasm | 21.25 | 11.36 |
Mucinous cystic neoplasm | 27.59 | 25 |
Neuroendocrine tumor | 27.12 | 26.47 |
Solid pseudopapillary neoplasm | 10.71 | 9.09 |
Intraductal papillary mucinous neoplasm | 38.47 | 62.5 |
Pseudocyst | 23.53 | 50 |
Chronic pancreatitis | 41.67 | 40 |
Other | 29.17 | 13.64 |
NODM, New-onset diabetes mellitus; PUMCH, Peking Union Medical College Hospital; GDPH, Guangdong Provincial People’s Hospital; DP, distal pancreatectomy.
Identification of NODM risk factors
The results of univariate and multivariate Cox regression analyses were shown in Table 2. In univariate Cox regression analysis, age, gender, BMI, ASA score, length of the resected pancreas, operative approach, operative time, intraoperative blood loss, concomitant splenectomy, pathological diagnosis, and postoperative complications were identified as candidate risk factors. With a minimum value of lambda (λ), 9 variables (age, gender, BMI, length of resected pancreas, operative approach, operative time, intraoperative blood loss, splenectomy, and postoperative complications) were screened after LASSO regression (Figure 2). According to AIC minimum, age (1.0304765, 95% CI: 1.016–1.045, P<0.001), BMI (1.0608107, 95% CI: 1.012–1.112, P<0.05), length of the resected pancreas (1.0933642, 95% CI: 1.041–1.148, P<0.001), intraoperative blood loss (1.0003717, 95% CI: 1.000–1.001, P<0.01), and concomitant splenectomy (1.8725660, 95% CI: 1.152–3.043, P<0.05) were determined as independent risk factors at AIC =1,150.55. These were significantly related to the outcome of NODM after DP.
Table 2
Factors | HR (95% CI) | P value |
---|---|---|
Univariate Cox regression analysis | ||
Age (years) | 1.034 (1.021–1.048) | 3.57e-07*** |
Gender | ||
Female | – | |
Male | 1.703 (1.157–2.508) | 0.00701** |
Family history of type 2 diabetes (first-degree relatives) | ||
No | – | |
Yes | 0.7369 (0.2712–2.002) | 0.549 |
Body mass index (kg/m2) | 1.085 (1.04–1.132) | 0.000153*** |
American Society of Anesthesiology score | ||
I | – | |
II | 1.718 (1.1343–2.602) | 0.0106* |
III | 1.151 (0.2744–4.833) | 0.8471 |
Component of tumor | ||
Cystic | – | |
Cystic and solid | 0.7811 (0.4549–1.341) | 0.371 |
Solid | 1.1011 (0.7263–1.670) | 0.650 |
Tumor size (cm) | 0.9895 (0.9306–1.052) | 0.737 |
Length of the resected pancreas (cm) | 1.094 (1.044–1.146) | 0.000165*** |
Pathological diagnosis | ||
Serous cystic neoplasm | – | |
Mucinous cystic neoplasm | 1.1091 (0.5950–2.0674) | 0.7445 |
Neuroendocrine tumor | 1.0964 (0.6081–1.9766) | 0.7596 |
Solid pseudopapillary neoplasm | 0.4204 (0.1873–0.9435) | 0.0356* |
Intraductal papillary mucinous neoplasm | 1.9876 (0.9096–4.3433) | 0.0850 |
Pseudocyst | 1.0200 (0.3431–3.0324) | 0.9716 |
Chronic pancreatitis | 2.2989 (0.8471–6.2384) | 0.1022 |
Other | 1.2634 (0.5234–3.0495) | 0.6030 |
Operative approach | ||
Minimally invasive surgery | – | |
Open surgery | 1.726 (1.128–2.64) | 0.0119* |
Operative time (min) | 1.003 (1–1.005) | 0.0206* |
Intraoperative blood loss (mL) | 1 (1–1.001) | 3.49e-08*** |
Concomitant splenectomy | ||
No | – | |
Yes | 2.716 (1.7–4.339) | 2.92e-05*** |
Postoperative complication | ||
No | – | |
Yes | 1.988 (1.328–2.976) | 0.000843*** |
Clinical postoperative pancreatic fistula (grades B and C) | ||
No | – | |
Yes | 1.418 (0.739–2.72) | 0.294 |
Intraoperative blood transfusion | ||
No | – | |
Yes | 1.372 (0.8259– 2.279) | 0.222 |
Multivariate Cox regression analysis | ||
Age (years) | 1.0304765 (1.016–1.045) | 1.99e-05*** |
Body mass index (kg/m2) | 1.0608107 (1.012–1.112) | 0.014198* |
Length of the resected pancreas (cm) | 1.0933642 (1.041–1.148) | 0.000367*** |
Intraoperative blood loss (mL) | 1.0003717 (1.000–1.001) | 0.001436** |
Concomitant splenectomy | ||
No | – | |
Yes | 1.8725660 (1.152–3.043) | 0.011357* |
*, P<0.05; **, P<0.01; ***, P<0.001. HR, hazard ratio; CI, confidence interval.
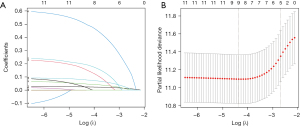
The data on risk factors for postoperative NODM in the training cohort and the validation cohort were summarized in Table 3. They were divided into two groups according to the outcome of postoperative NODM. The mean age of patients developing postoperative NODM was 50.6±12.8 years old in the training cohort and 52.8±13.5 years old in the validation cohort. Patients with postoperative NODM were significantly older than those who did not develop postoperative NODM (P<0.05). The mean BMI values of patients with newly diagnosed DM were greater than those without NODM in the training cohort (24.5±3.53 vs. 23.0±3.89 kg/m², P<0.05) and the validation cohort (24.8±4.00 vs. 22.3±3.92 kg/m², P<0.05). The longer the resected pancreas, the higher the risk of NODM. In the training and the validation cohorts, the average lengths of the resected pancreas for newly diagnosed diabetic patients were 10.7±3.68 and 8.44±2.90 cm, respectively. Only in the training cohort, the resected pancreatic length was markedly longer in patients with postoperative NODM than those without (P<0.05). The mean intraoperative blood loss in the training cohort was higher in patients with postoperative NODM than in those without (631±1,100 vs. 388±478 mL, P<0.05), whereas no significant difference was found in the validation cohort (236±389 vs. 178±248 mL, P>0.05). Patients with concomitant splenectomy had an increased risk of NODM in the training cohort (P<0.05). However, the patients with concomitant splenectomy did not have a greater chance of developing the postoperative NODM in the validation cohort (P>0.05).
Table 3
Factors | Training set | Validation set | |||||
---|---|---|---|---|---|---|---|
No (N=340) | Yes (N=108) | Total (N=448) | No (N=141) | Yes (N=37) | Total (N=178) | ||
Age (years) | 42.5±15.3 | 50.6±12.8 | 44.4±15.1 | 44.2±14.2 | 52.8±13.5 | 46.0±14.4 | |
Body mass index (kg/m2) | 23.0±3.89 | 24.5±3.53 | 23.4±3.85 | 22.3±3.92 | 24.8±4.00 | 22.8±4.05 | |
Length of the resected pancreas (cm) | 9.32±3.46 | 10.7±3.68 | 9.66±3.56 | 8.48±3.40 | 8.44±2.90 | 8.47±3.30 | |
Intraoperative blood loss (mL) | 388±478 | 631±1,100 | 446±690 | 178±248 | 236±389 | 190±283 | |
Concomitant splenectomy | |||||||
No | 151 (44.4) | 22 (20.4) | 173 (38.6) | 83 (58.9) | 22 (59.5) | 105 (59.0) | |
Yes | 189 (55.6) | 86 (79.6) | 275 (61.4) | 58 (41.1) | 15 (40.5) | 73 (41.0) |
Data are presented as mean ± SD or n (%). N, number; SD, standard deviation.
Construction and validation of the nomogram for predicting NODM
The nomogram for predicting postoperative NODM in patients after DP, comprised of age, BMI, length of the resected pancreas, intraoperative blood loss, and concomitant splenectomy, was shown in Figure 3. By summing the scores corresponding to each factor to obtain a total score, we estimated and inferred the incidence of NODM for different patients at 1, 3, and 5 years to achieve the targeted detection for high-risk patients in advance (Figure 3).
The C-index of the prediction model was 0.739 (95% CI: 0.628–0.826) and 0.719 (95% CI: 0.518–0.859) in the training and validation cohorts, respectively. As shown in Figure 4, the AUC of the prediction model at 1, 3, and 5 years represented the comparative predictive accuracy of the total scores calculated using the nomogram and each independent predictor. The risk scores derived from the nomogram had greater AUC than each independent predictor for the 1-, 3-, and 5-year incidence rates, and all were more than 0.6 in the training cohort (AUC =0.779, 0.778, and 0.744, respectively) and the validation cohort (AUC =0.805, 0.751, and 0.666, respectively), indicating that the nomogram had an excellent predictive ability.
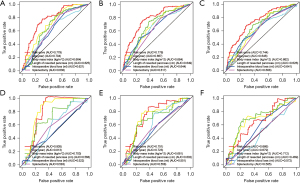
Calibration plots were used to evaluate the goodness-of-fit of the prediction model. There was a considerable agreement between the non-incidence rate predicted by the nomogram and the non-incidence rate calculated in the training cohort at 1, 3, and 5 years (Figure 5). Nevertheless, the calibration curves did not completely coincide with the fitting dashed line in the validation group and were mainly located around its sides, suggesting that the prediction model could estimate the risk of postoperative NODM in the patients after DP surgery. DCA curves indicated that the prediction model had a certain clinical utility but might overestimate the outcomes of the validation group (Figure 6).
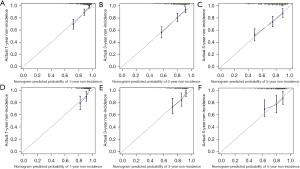
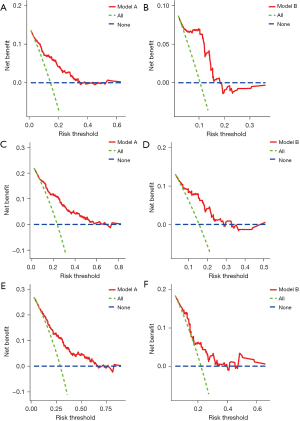
Risk stratification assessment of the nomogram for predicting NODM
To assess the association between the concurrence of NODM and the identified risk predictors, we divided the patients into high- and low-risk groups according to the risk scores calculated using the nomogram based on maximally selected rank statistics. Figure 7A showed the best cut-off point for the risk prediction value was 2.88 in the PUMCH cohort. According to this value, the patients were stratified by risk scores in the PUMCH and the GDPH cohorts. Cumulative event probability curves showed a significant difference between the high- and low-risk groups in the PUMCH cohort. The same result was observed in the GDPH cohort (Figure 7B,7C, both P<0.05).
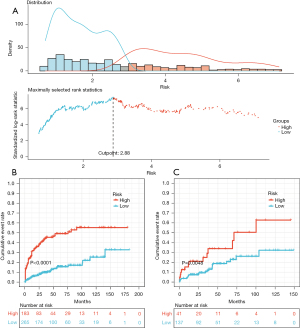
Discussion
Literature reviews suggest that research on the prediction model of NODM after DP is scanty. Nomograms have been widely used in recent years for their ability to estimate probabilities and provide easy-to-understand information through simple graphics (18). To assist in assessing postoperative diabetic risk and guide early detection and treatment of DM, we analyzed extensive follow-up data from large-scale PBLML patients undergoing DP in two tertiary hospitals, identified five clinically accessible risk factors by LASSO and Cox proportional-hazards model, and established the first nomogram model showing high predictive efficiency. The ROC curve, calibration curve, DCA analysis, and risk stratification analysis indicated the accuracy and reliability of the nomogram.
Because of the favorable survival prognosis in this study, the endpoint event (NODM) could be observed with a sufficiently long follow-up period, thus ensuring the observation of the abnormal progression from IFG or IGT to apparent DM in these patients. The cumulative incidence of NODM after DP increased over time. The nomogram to predict NODM after DP was established and verified in different data cohorts, showing practical and reliable risk prediction and discrimination capabilities. This nomogram could predict the likelihood of NODM in patients with PBLML after DP by calculating five easy-to-obtain clinical variables. In addition, physicians can classify high- and low-risk patients according to the specific cut-off point, enhancing interventions for high-risk patients and reducing unnecessary testing and treatment costs for low-risk patients.
Metabolic deterioration in the aging body promotes the occurrence of metabolic diseases such as DM (19). In this study, older age and higher BMI were significantly associated with NODM after DP. Previous studies suggest that elderly patients are at an increased risk of postoperative DM (20,21). Obesity is a risk factor for diabetes due to pancreatic fatty infiltration leading to β-cell dysfunction and visceral fat deposition leading to insulin resistance (22,23). Besides, Kang et al. and Kwon et al. found that higher preoperative BMI significantly predicted endocrine dysfunction in glucose metabolism after DP (24,25).
According to the nomogram scale, the length of the resected pancreas was the most important predictor. The length of the resected pancreas, measured from the distal to the proximal end of the surgical specimen, roughly represents the removed volume. We are able to infer the residual pancreas volume based on the length of the resected pancreas and the preoperative imaging reports. This method is relatively simple and feasible, even if the patients do not undergo postoperative imaging review or have inconsistent postoperative review times. Although the length of the resected pancreas may be a non-adjustable factor due to the site and size of the lesions, this does not affect its predictive effect on the risk of postoperative diabetes (26). The results of a systematic review indicated that the higher the percentage of pancreatic volume removed, the greater the risk of developing DM after pancreatectomy (27). This might be related to the loss of pancreatic islet cells, thus affecting the endocrine and exocrine function of the residual pancreas. Kang et al. found that the resected pancreatic volume greater than 25% was a risk factor for impaired pancreatic endocrine function (24). Wen et al. suggested that the resected pancreatic volume greater than 40% was more likely to cause early postoperative hyperglycemia (28). Therefore, appropriate pancreatic resection can prevent the development and progression of postoperative DM.
Intriguingly, increased intraoperative blood loss and concomitant splenectomy were closely associated with the occurrence of postoperative NODM. Intraoperative blood loss is related to pancreatic texture and lesion blood supply. Excessive blood loss may lead to stress responses and inflammatory damage in the residual pancreas. There is a lack of adequate perfusion of the pancreas due to excessive blood loss. Meanwhile, excessive intraoperative fluid supplements can lead to pancreatic edema and reperfusion injury. Subsequently, these factors may lead to endocrine insufficiency of the pancreas (29,30). The pancreas is a source of pluripotent stem cells, including precursor cells for pancreatic beta cells. Hox11+ stem cells from the spleen can differentiate into pancreatic islets, as demonstrated in animal models (31). Pancreatectomy plus splenectomy has been flexibly applied to construct experimental animal models of DM (32). Previous studies have shown that patients who undergo splenectomy with pancreatectomy are more likely to develop postoperative diabetes than those who undergo spleen-preserving pancreatectomy. In addition, a higher incidence of postoperative diabetes or abnormal glucose tolerance has been found in patients who have undergone splenectomy due to splenic trauma or hematological disorders (33). Although there were a greater number of newly diagnosed patients with postoperative DM in the validation cohort who also did not receive splenectomy, no significant proportional differences were found. Moreover, preserving the spleen can reduce postoperative complications, including infection and bleeding, shorten hospital stays, and avoid postoperative DM (34).
However, some limitations of this study warrant further consideration. First, although the sample size was large and the follow-up time was long enough, some cases were lost to follow-up, and the outcome was ambiguous, which might have caused information bias. The patients’ final follow-up and complete perioperative data were included in the analyses to avoid data loss. The diagnosis of NODM was made according to the test results for fast blood glucose, along with the need for glucose-lowering drugs or insulin. NODM was not clearly defined as T3cDM or T2DM. Second, we acknowledge the omission of investigations into the preoperative assessment of pancreatic endocrine function (e.g., blood C-peptide and urinary C-peptide levels). A significant positive correlation between the postoperative 24 h urinary C-peptide excretion rate and the multiplication of the residual pancreatic volume ratio and the preoperative 24 h urinary C-peptide excretion rate is reported, particularly in patients undergoing DP (35). Therefore, perioperative 24 h urinary C-peptide levels might be important in predicting the development of NODM after DP. Finally, although we validated the prediction model with data from another institution and the validation results were favorable, further research and evaluation of the applicability of this prediction tool to other populations are necessary.
Conclusions
In conclusion, a novel clinical model showing satisfactory risk prediction performance and risk stratification capability was developed and validated. The nomogram might be conducive to preoperative surgical planning and prevention and treatment of postoperative DM.
Acknowledgments
Funding: This work was supported by a grant from
Footnote
Reporting Checklist: The authors have completed the TRIPOD reporting checklist. Available at https://hbsn.amegroups.com/article/view/10.21037/hbsn-22-382/rc
Data Sharing Statement: Available at https://hbsn.amegroups.com/article/view/10.21037/hbsn-22-382/dss
Conflicts of Interest: All authors have completed the ICMJE uniform disclosure form (available at https://hbsn.amegroups.com/article/view/10.21037/hbsn-22-382/coif). The authors have no conflicts of interest to declare.
Ethical Statement: The authors are accountable for all aspects of the work in ensuring that questions related to the accuracy or integrity of any part of the work are appropriately investigated and resolved. The study was conducted in accordance with the Declaration of Helsinki (as revised in 2013). Ethical approval of this study was obtained from the Medical Research Ethics Committee of Guangdong Provincial People’s Hospital (No. GDREC2018305H) and the patients signed informed consent before the surgery and the follow-up survey.
Open Access Statement: This is an Open Access article distributed in accordance with the Creative Commons Attribution-NonCommercial-NoDerivs 4.0 International License (CC BY-NC-ND 4.0), which permits the non-commercial replication and distribution of the article with the strict proviso that no changes or edits are made and the original work is properly cited (including links to both the formal publication through the relevant DOI and the license). See: https://creativecommons.org/licenses/by-nc-nd/4.0/.
References
- Zheng Y, Ley SH, Hu FB. Global aetiology and epidemiology of type 2 diabetes mellitus and its complications. Nat Rev Endocrinol 2018;14:88-98. [Crossref] [PubMed]
- Hart PA, Bellin MD, Andersen DK, et al. Type 3c (pancreatogenic) diabetes mellitus secondary to chronic pancreatitis and pancreatic cancer. Lancet Gastroenterol Hepatol 2016;1:226-37. [Crossref] [PubMed]
- Wei Q, Qi L, Lin H, et al. Pathological Mechanisms in Diabetes of the Exocrine Pancreas: What's Known and What's to Know. Front Physiol 2020;11:570276. [Crossref] [PubMed]
- Wynne K, Devereaux B, Dornhorst A. Diabetes of the exocrine pancreas. J Gastroenterol Hepatol 2019;34:346-54. [Crossref] [PubMed]
- Duggan SN, Ewald N, Kelleher L, et al. The nutritional management of type 3c (pancreatogenic) diabetes in chronic pancreatitis. Eur J Clin Nutr 2017;71:3-8. [Crossref] [PubMed]
- Bhattamisra SK, Siang TC, Rong CY, et al. Type-3c Diabetes Mellitus, Diabetes of Exocrine Pancreas - An Update. Curr Diabetes Rev 2019;15:382-94. [Crossref] [PubMed]
- Vonderau JS, Desai CS. Type 3c: Understanding pancreatogenic diabetes. JAAPA 2022;35:20-4. [Crossref] [PubMed]
- Cho J, Scragg R, Pandol SJ, et al. Exocrine Pancreatic Dysfunction Increases the Risk of New-Onset Diabetes Mellitus: Results of a Nationwide Cohort Study. Clin Transl Sci 2021;14:170-8. [Crossref] [PubMed]
- De Bruijn KM, van Eijck CH. New-onset diabetes after distal pancreatectomy: a systematic review. Ann Surg 2015;261:854-61. [Crossref] [PubMed]
- Yu J, Sun R, Han X, et al. New-Onset Diabetes Mellitus After Distal Pancreatectomy: A Systematic Review and Meta-Analysis. J Laparoendosc Adv Surg Tech A 2020;30:1215-22. [Crossref] [PubMed]
- Tariq M, Jajja MR, Maxwell DW, et al. Diabetes development after distal pancreatectomy: results of a 10 year series. HPB (Oxford) 2020;22:1034-41. [Crossref] [PubMed]
- Burkhart RA, Gerber SM, Tholey RM, et al. Incidence and severity of pancreatogenic diabetes after pancreatic resection. J Gastrointest Surg 2015;19:217-25. [Crossref] [PubMed]
- Wang X, Misawa R, Zielinski MC, et al. Regional differences in islet distribution in the human pancreas--preferential beta-cell loss in the head region in patients with type 2 diabetes. PLoS One 2013;8:e67454. [Crossref] [PubMed]
- Wang X, Zielinski MC, Misawa R, et al. Quantitative analysis of pancreatic polypeptide cell distribution in the human pancreas. PLoS One 2013;8:e55501. [Crossref] [PubMed]
- Ravi PK, Singh SR, Mishra PR. Redefining the tail of pancreas based on the islets microarchitecture and inter-islet distance: An immunohistochemical study. Medicine (Baltimore) 2021;100:e25642. [Crossref] [PubMed]
- Yamamoto-Kataoka S, Shimizu S, Yamazaki H, et al. Development of a preoperative prediction model for new-onset diabetes mellitus after partial pancreatectomy: A retrospective cohort study. Medicine (Baltimore) 2021;100:e26311. [Crossref] [PubMed]
- Borakati A, Banu Z, Raptis DA, et al. New onset diabetes after partial pancreatectomy: development of a novel predictive model using machine learning. HPB (Oxford) 2021;23:S814.
- Balachandran VP, Gonen M, Smith JJ, et al. Nomograms in oncology: more than meets the eye. Lancet Oncol 2015;16:e173-80. [Crossref] [PubMed]
- Tamura Y, Omura T, Toyoshima K, et al. Nutrition Management in Older Adults with Diabetes: A Review on the Importance of Shifting Prevention Strategies from Metabolic Syndrome to Frailty. Nutrients 2020;12:3367. [Crossref] [PubMed]
- Nguyen A, Demirjian A, Yamamoto M, et al. Development of Postoperative Diabetes Mellitus in Patients Undergoing Distal Pancreatectomy Whipple Procedure. Am Surg 2017;83:1050-3.
- Donovan AL, Furlan A, Borhani AA, et al. Evaluation of clinical and imaging biomarkers for the prediction of new onset diabetes following pancreatic resection. Abdom Radiol (NY) 2021;46:2628-36. [Crossref] [PubMed]
- Barazzoni R, Gortan Cappellari G, Ragni M, et al. Insulin resistance in obesity: an overview of fundamental alterations. Eat Weight Disord 2018;23:149-57. [Crossref] [PubMed]
- Inaishi J, Saisho Y. Beta-Cell Mass in Obesity and Type 2 Diabetes, and Its Relation to Pancreas Fat: A Mini-Review. Nutrients 2020;12:3846. [Crossref] [PubMed]
- Kang JS, Jang JY, Kang MJ, et al. Endocrine Function Impairment After Distal Pancreatectomy: Incidence and Related Factors. World J Surg 2016;40:440-6. [Crossref] [PubMed]
- Kwon JH, Kim SC, Shim IK, et al. Factors Affecting the Development of Diabetes Mellitus After Pancreatic Resection. Pancreas 2015;44:1296-303. [Crossref] [PubMed]
- Hwang HK, Park J, Choi SH, et al. Predicting new-onset diabetes after minimally invasive subtotal distal pancreatectomy in benign and borderline malignant lesions of the pancreas. Medicine (Baltimore) 2017;96:e9404. [Crossref] [PubMed]
- Wu L, Nahm CB, Jamieson NB, et al. Risk factors for development of diabetes mellitus (Type 3c) after partial pancreatectomy: A systematic review. Clin Endocrinol (Oxf) 2020;92:396-406. [Crossref] [PubMed]
- Wen K, Cen C, Wu L, et al. Relationship between pancreatic parenchyma loss and early postoperative hyperglycemia in patients with benign pancreatic diseases. Abdom Radiol (NY) 2021;46:4210-7. [Crossref] [PubMed]
- Dinić S, Arambašić Jovanović J, Uskoković A, et al. Oxidative stress-mediated beta cell death and dysfunction as a target for diabetes management. Front Endocrinol (Lausanne) 2022;13:1006376. [Crossref] [PubMed]
- Callery MP, Pratt WB, Kent TS, et al. A prospectively validated clinical risk score accurately predicts pancreatic fistula after pancreatoduodenectomy. J Am Coll Surg 2013;216:1-14. [Crossref] [PubMed]
- Faustman DL, Davis M. Stem cells in the spleen: therapeutic potential for Sjogren's syndrome, type I diabetes, and other disorders. Int J Biochem Cell Biol 2010;42:1576-9. [Crossref] [PubMed]
- Heinke S, Ludwig B, Schubert U, et al. Diabetes induction by total pancreatectomy in minipigs with simultaneous splenectomy: a feasible approach for advanced diabetes research. Xenotransplantation 2016;23:405-13. [Crossref] [PubMed]
- Wu SC, Fu CY, Muo CH, et al. Splenectomy in trauma patients is associated with an increased risk of postoperative type II diabetes: a nationwide population-based study. Am J Surg 2014;208:811-6. [Crossref] [PubMed]
- Pendola F, Gadde R, Ripat C, et al. Distal pancreatectomy for benign and low grade malignant tumors: Short-term postoperative outcomes of spleen preservation-A systematic review and update meta-analysis. J Surg Oncol 2017;115:137-43. [Crossref] [PubMed]
- Yamada D, Takahashi H, Asukai K, et al. Investigation of the influence of pancreatic surgery on new-onset and persistent diabetes mellitus. Ann Gastroenterol Surg 2021;5:575-84. [Crossref] [PubMed]