Predictive model for very early recurrence of patients with perihilar cholangiocarcinoma: a machine learning approach
Highlight box
Key findings
• In this multi-institutional cohort study, 15.0% of patients developed very early recurrence (VER) following resection for perihilar cholangiocarcinoma (pCCA) with a detrimental association with overall survival (OS) (3-year OS, 9.2%). Predictive models and an easy-to-use risk calculator were developed to identify high-risk patients for VER with good predictive accuracy in the training as well as the internal validation data sets.
What is known and what is new?
• While surgical treatment is the only potentially curative option for patients with localized pCCA, it is associated with high mortality rates and sometimes poor oncological outcomes.
• The frequency, prognosis, and risk factors of VER in patients who underwent curative-intent surgery for pCCA have been identified.
What is the implication, and what should change now?
• These data emphasize that VER is associated with poor prognosis after pCCA resection and highlight the need for individualize management of perioperative treatment and surveillance according to the risk of recurrence in the early postoperative period.
Introduction
Perihilar cholangiocarcinoma (pCCA) is the most common primary bile duct malignancy with an increasing incidence over the last several decades (1,2). Unfortunately, surgical treatment, which is the only potentially curative treatment for patients with localized pCCA, has been associated with high mortality and sometimes poor oncological outcomes (3). Of note, 44.0% to 83.2% of patients with pCCA will have a recurrence after curative-intent resection (3-5). Additionally, postoperative adjuvant chemotherapy has only modest benefits among patients following resection of pCCA (6). Accurate identification of patients who are at high risk of developing recurrence may help select individuals who benefit the most from surgical resection. In particular, a subset of patients may experience early recurrence within the first year of surgery (3-5,7-9). Patients with early versus late recurrence of pCCA generally have a worse prognosis following resection (3). In turn, patients who have an early recurrence often experience decisional regret, depression, and worse quality-of-life (10-12).
Early recurrence for pCCA, as well as other hepatobiliary cancers, has been defined as a recurrence occurring within 12 to 30 months after surgical resection (3,13-15). Notably, a previous study from our own group reported that approximately one-quarter of patients with intrahepatic cholangiocarcinoma (ICC) had very early recurrence (VER) (i.e., recurrence within 6 months after initial resection) (16,17). Patients with ICC who experienced VER had a very poor overall survival (OS) that was similar to patients with advanced cholangiocarcinoma who received systemic therapy only, indicating that patients at risk for VER may derive little benefit from resection (16,17). Prediction of VER may also help frame individualized surveillance strategies following resection, as well as inform adjunct treatment including perioperative systemic chemotherapy (3). To date, there are no tools to predict VER among pCCA patients following curative-intent resection for pCCA. Most predictive models have utilized conventional statistical techniques and have not employed novel machine learning (ML) methods (18). As such, the objective of the current study was to develop a predictive model to identify individuals at risk of VER after curative-intent surgery for pCCA. We developed and validated an ML model [i.e., eXtreme gradient boosting (XGBoost) algorithm] to predict VER using a large international multi-institutional database. Furthermore, to facilitate the clinical applicability of the model, an easy-to-use online calculator to predict the risk of VER among individuals undergoing curative-intent surgery for pCCA was provided. This article is presented in accordance with the TRIPOD reporting checklist (available at https://hbsn.amegroups.com/article/view/10.21037/hbsn-24-385/rc).
Methods
Study population and data collection
Patients who underwent curative-intent resection for pCCA between 2000 and 2023 were identified from an international multi-institutional database from eight major hepatobiliary institutions across the globe. VER of pCCA was defined as recurrence within 6 months after resection, consistent with the definition used in previous studies (16,19-22). Patients who had extrahepatic metastasis, R2 resection margins, or who died within 30 days following surgery were excluded. Additionally, individuals who had palliative surgery or who had missing data on key clinicodemographic characteristics were also not included. The study was conducted in accordance with the Declaration of Helsinki (as revised in 2013). This study was approved by the Institutional Review Board of The Ohio State University Wexner Medical System (IRB#2108C0025). All participating institutions (Yokohama City University, University of Verona, Curry Cabral Hospital, Emory University, Erasmus University Medical Centre, University of Sydney, Cleveland Clinic, and The Ohio State University Wexner Medical System) were informed and agreed the study. Obtaining informed consent from all individual participants was not required as the study relied on secondary data analysis of de-identified patient data.
Variables and outcomes of interest
Patient demographic and clinicopathologic variables included age, sex, year of surgery (i.e., 2000–2010 or 2011–2023), region (i.e., western or eastern), receipt of preoperative systemic chemotherapy, preoperative jaundice, portal vein embolization (PVE), preoperative carbohydrate antigen 19-9 (CA19-9), type of surgery (i.e., extrahepatic bile duct resection only, right hepatectomy, left hepatectomy, extended right hepatectomy, extended left hepatectomy, right trisectionectomy, left trisectionectomy, central hepatectomy, or other), combined vascular resection, lymphadenectomy, intraoperative blood loss, operation time, intraoperative frozen section analysis of margin status, number of harvested lymph node (LN) (i.e., <6 or ≥6), T-category (i.e., T1/T2 versus T3/T4), LN metastasis, pathological stage, pathological tumor size, tumor differentiation (i.e., well, moderately differentiated versus poorly, undifferentiated), microvascular invasion (MVI), perineural invasion (PNI), pathological margin status, as well as postoperative severe complications and receipt of adjuvant chemotherapy. The American Joint Committee on Cancer (AJCC) 8th edition staging manual was used for T, N-category classification, and staging (23). Severity of postoperative complications was defined according to the Clavien-Dindo classification system (grade I–V); severe complications were defined as Clavien-Dindo classification ≥ III (24).
In this multi-institutional study, the indication of surgical treatment was guided by the protocols of each participating institution. The standard approach for pCCA generally involved a combination of liver resection and bile duct resection. If R0 resection was deemed achievable with extrahepatic bile duct resection alone, this approach was very seldomly used as certain institutions. Decisions regarding the type of resection and surgical techniques were, therefore, tailored to each institution and treating surgeon. Following curative-intent resection, patients were monitored for recurrence based on serum tumor markers and imaging, such as computed tomography and/or magnetic resonance imaging. Patients were followed once every 3 to 4 months during the first 3 years, once every 6 months during the 4th and 5th years, and then annually (16). The criteria for administering adjuvant chemotherapy were based on each institution’s protocol. Recurrence-free survival (RFS) was defined as the time elapsed between the date of resection and recurrence confirmed on biopsy or evidence of a suspicious lesion on follow-up imaging; OS was defined as the time interval between the date of resection for pCCA and date of death or last follow-up. Recurrence was categorized as either local or distant. Local recurrence was defined as a recurrence at the hepaticojejunostomy or in an area where surgical procedures had been performed, including the liver hilum and hepatoduodenal ligament. All other recurrences were defined as distant (25). Treatment of tumor recurrence was based on consensus among the multidisciplinary team at each institution.
Statistical analysis
Descriptive statistics were presented as median values [interquartile range (IQR)] and frequency (%) for continuous and categorical variables, respectively. Continuous variables were compared using the Mann-Whitney U or Kruskal-Wallis tests, as appropriate. While categorical variables were compared with the Fishers exact test (χ2). Multiple imputations with chain equations (MICE) procedures were utilized to handle missing values (26). Survival was assessed using Kaplan-Meier curves along with log-rank tests and statistical significance was determined at α=0.05.
The XGBoost-algorithm-powered survival model was employed to develop a predictive model of VER following curative-intent resection of pCCA (27). XGBoost is an advanced implementation of the gradient boosting framework designed for speed and performance (28). This approach builds an ensemble of decision trees in which each new tree corrects the errors of the previous trees, resulting in a robust predictive model (28). Candidate variables for the XGBoost model, which were selected based on the literature, included age, preoperative jaundice, preoperative CA19-9 level, the number of harvested LN, AJCC T category, LN metastasis, tumor size, tumor differentiation, MVI, PNI, margin status, severe complication, and adjuvant chemotherapy (3,9,29-31). In the present study, hypermeters, which are used to control the learning process of ML models, were selected by grid search cross-validation (28). The c-index was calculated for the entire dataset followed by the bootstrapping resampling method (n=5,000) to assess the performance of the predictive model. SHapley Additive exPlanations (SHAP) values were used to interpret the predictions of the predictive model for VER (32). This approach is based on cooperative game theory and the concept of Shapley values, ensuring a fair distribution of “payout” (feature importance) among features (32). Subsequently, the patients were stratified into low-, intermediate-, or high-risk for VER using the model based on optimal cutoffs determined using the X-tile program (33). An easy-to-use web application to calculate risk at 6 months after resection was made freely available (https://junkawashima.shinyapps.io/VER_hilar/). The predictive ability of recurrence within 6 months after surgery was compared between the proposed predictive model and the AJCC 8th Edition Staging System, which includes stages I, II, IIIA, IIIB, IIIC, and IVA (23). Model discrimination was estimated using the area under the time-dependent receiver operating characteristic curve (time-dependent AUC) at 6 months after curative-intent resection.
An additional analysis to construct a preoperative predictive model was performed, which excluded data from three institutions that lacked preoperative examination information. This model incorporated preoperative clinical factors such as age, lymph node disease detected on preoperative imaging, portal vein or hepatic artery invasion observed on preoperative imaging, preoperative serum total bilirubin, systemic immune-inflammation index (SII), and CA19-9. The SII was calculated by multiplying the platelet-to-lymphocyte ratio by platelet counts (33). The preoperative predictive model was constructed using the XGBoost algorithm. The c-index was calculated for the additional analysis dataset, and the bootstrapping resampling method (n=5,000) was applied to evaluate the performance of the preoperative predictive model. SHAP values were applied to interpret the model’s predictions for VER. Patients were then stratified into low- or high-risk groups for VER based on optimal cutoffs determined through the X-tile program, and the model was integrated into the online calculator. All statistical analyses were performed using R version 4.2.0 (R Project for Statistical Computing, Vienna, Austria).
Results
Baseline cohort characteristics
Overall, 496 patients underwent curative-intent surgery for pCCA; 28 patients were excluded due to 30-day postoperative mortality [the 30-day mortality: 5.6% (n=28)]; an additional 34 patients were excluded related to other exclusion criteria. Among 434 patients included in the analytic cohort, median patient age was 68.0 (IQR: 58.0–74.5) years and 255 (58.8%) patients were male (Table 1). Overall, most patients (n=329, 75.8%) had preoperative jaundice and some patients underwent PVE (n=107, 24.7%) with a median preoperative serum CA19-9 of 114.5 (IQR: 24.7–563.8) U/mL. Only a small number of patients (n=23, 5.3%) received preoperative systemic chemotherapy. Most patients underwent liver resection combined with bile duct resection (n=403, 92.9%) with lymphadenectomy (n=415, 95.6%), vascular resection (n=150, 34.6%); roughly half of the patients had ≥6 LNs harvested (n=247, 56.9%). On postoperative pathology, primary tumor was classified as T3/T4 disease (n=117, n=27.0%) with LN metastasis reported in 176 (40.6%) patients. Most patients had a moderate to well-differentiated tumor (n=331, 76.3%) with PNI (n=364, 83.9%) and a median size of 3.0 (IQR: 2.0–4.0) cm. Some patients had MVI (n=179, 41.2%) and a positive resection margin (n=160, 36.9%); a total of 169 patients (38.9%) experienced a postoperative severe complication, and 20 (4.3%) patients died within 90-day. Among the 160 (36.9%) patients who received adjuvant chemotherapy, 61 (14.0%) were treated with Gemcitabine-based regimens, 39 (9.0%) with capecitabine, and 32 (7.4%) with other regimens. Information on the remaining 28 (6.4%) patients was not available. Of note, roughly two-thirds of patients still experienced a recurrence (n=269, 62.0%).
Table 1
Characteristics | Total, n=434 | Non-VER, n=369 (85.0%) | VER, n=65 (15.0%) | P value |
---|---|---|---|---|
Age, years, median (IQR) | 68.0 (58.0–74.5) | 68.0 (58.0–74.0) | 69.0 (58.0–75.0) | 0.38 |
Sex, male, n (%) | 255 (58.8) | 220 (59.6) | 35 (53.8) | 0.46 |
Year of surgery, n (%) | >0.99 | |||
2000–2010 | 119 (27.4) | 101 (27.4) | 18 (27.7) | |
2011–2023 | 315 (72.6) | 268 (72.6) | 47 (72.3) | |
Region, n (%) | 0.24 | |||
Western | 402 (92.6) | 339 (91.9) | 63 (96.9) | |
Eastern | 32 (7.4) | 30 (8.1) | 2 (3.1) | |
Preoperative chemotherapy, n (%) | 23 (5.3) | 21 (5.7) | 2 (3.0) | 0.57 |
Jaundice, n (%) | 329 (75.8) | 275 (74.5) | 54 (83.1) | 0.18 |
Portal vein embolization, n (%) | 107 (24.7) | 94 (25.5) | 13 (20.0) | 0.43 |
CA19-9 (U/mL), median (IQR) | 114.5 (24.7–563.8) | 107.0 (23.0–560.0) | 175.5 (42.7–830.0) | 0.23 |
Type of resection, n (%) | – | |||
Extrahepatic bile duct resection only | 31 (7.1) | 25 (6.8) | 6 (9.2) | |
Right hepatectomy | 50 (11.5) | 47 (12.7) | 3 (4.6) | |
Left hepatectomy | 22 (5.0) | 22 (6.0) | 0 (0.0) | |
Extended right hepatectomy | 69 (15.9) | 64 (17.3) | 5 (7.7) | |
Extended left hepatectomy | 77 (17.7) | 69 (18.7) | 8 (12.3) | |
Right trisectionectomy | 64 (14.7) | 46 (12.5) | 18 (27.7) | |
Left trisectionectomy | 67 (15.4) | 57 (15.4) | 10 (15.4) | |
Central hepatectomy | 30 (6.9) | 20 (5.4) | 10 (15.4) | |
Others | 24 (5.5) | 19 (5.1) | 5 (7.7) | |
Lymphadenectomy, n (%) | 415 (95.6) | 355 (96.2) | 60 (92.3) | 0.28 |
Combined vascular resection, n (%) | 150 (34.6) | 117 (31.7) | 33 (50.8) | 0.005* |
Intraoperative frozen section analysis, n (%) | <0.001* | |||
Yes | 237 (54.6) | 220 (59.6) | 17 (4.6) | |
No | 37 (8.5) | 36 (9.8) | 1 (1.5) | |
Unknown | 160 (36.9) | 113 (30.6) | 47 (72.3) | |
Intraoperative blood loss, mL, median (IQR) | 524 (300–1,100) | 500 (300–1,028) | 1000 (462–1,665) | 0.11 |
Operative time, minute, median (IQR) | 540 (361–633) | 540 (364–632) | 536 (393–636) | 0.76 |
Number of harvested LN, n (%) | 0.08 | |||
<6 | 187 (43.1) | 152 (41.2) | 35 (53.8) | |
≥6 | 247 (56.9) | 217 (58.8) | 30 (46.2) | |
AJCC T category, n (%) | 0.37 | |||
T1/T2 | 317 (73.0) | 273 (74.0) | 44 (67.7) | |
T3/T4 | 117 (27.0) | 96 (26.0) | 21 (32.3) | |
AJCC N category, n (%) | 0.03* | |||
N0 | 258 (59.4) | 228 (61.8) | 30 (46.2) | |
N1/N2 | 176 (40.6) | 141 (38.2) | 35 (53.8) | |
AJCC stage, n (%) | 0.02* | |||
Stage I | 32 (7.4) | 32 (8.7) | 0 (0.0) | |
Stage II | 157 (36.2) | 139 (37.7) | 18 (27.7) | |
Stage IIIA | 53 (12.2) | 42 (11.4) | 11 (16.9) | |
Stage IIIB | 16 (3.7) | 15 (4.1) | 1 (1.5) | |
Stage IIIC | 142 (32.7) | 113 (30.6) | 29 (44.6) | |
Stage IVA | 34 (7.8) | 28 (7.6) | 6 (9.2) | |
Tumor size, cm, median (IQR) | 3.0 (2.0–4.0) | 2.7 (2.0–4.0) | 3.0 (2.2–4.0) | 0.09 |
Tumor differentiation, n (%) | 0.20 | |||
Well or moderate | 331 (76.3) | 286 (77.5) | 45 (69.2) | |
Poor | 103 (23.7) | 83 (22.5) | 20 (30.8) | |
Microvascular invasion, n (%) | 179 (41.2) | 144 (39.0) | 35 (53.8) | 0.04* |
Perineural invasion, n (%) | 364 (83.9) | 305 (82.7) | 59 (90.8) | 0.15 |
Margin status, n (%) | <0.001* | |||
R=0 | 274 (63.1) | 246 (66.7) | 28 (43.1) | |
R=1 | 160 (36.9) | 123 (33.3) | 37 (56.9) | |
Severe complications, n (%) | 169 (38.9) | 134 (36.3) | 35 (53.8) | 0.01* |
Adjuvant chemotherapy, n (%) | 160 (36.9) | 141 (38.2) | 19 (29.2) | 0.21 |
Recurrence, n (%) | 269 (62.0) | 204 (55.3) | 65 (100.0) | <0.001* |
*, P value <0.05. VER, very early recurrence; IQR, interquartile range; CA19-9, carbohydrate antigen 19-9; LN, lymph node; AJCC, The American Joint Committee on Cancer.
Association of clinicopathologic characteristics and survival with VER
Overall, 65 patients (15.0%) experienced a VER. Among the 369 (85.0%) patients who did not have a VER, 204 patients (47.0%) had a recurrence >6 months after resection, while 165 individuals (38.1%) did not experience a recurrence during the follow-up period. The recurrence pattern at the time of initial recurrence was detailed in Table S1. After a median follow-up of 21.1 (IQR: 10.5–41.3) months, patients with VER demonstrated worse median OS (8.4, IQR 6.6–11.3 vs. 38.5, IQR 31.9–45.7) months and 3-year OS (9.2% vs. 32.3%) than individuals without VER (P<0.001) (Figure 1). Patients with VER were more likely to have had concomitant hepatic resection with vascular resection (n=33, 50.8% vs. n=117, 31.7%; P=0.005), as well as had experienced a severe postoperative complication (n=35, 53.8% vs. n=134, 36.3%; P=0.01). Patients with VER more frequently had LN metastases (n=35, 53.8% vs. n=141, 38.2%; P=0.03), MVI (n=35, 53.8% vs. n=144, 39.0%; P=0.04), and a positive resection margin (n=37, 56.9% vs. n=123, 33.3%; P<0.001) (Table 1).
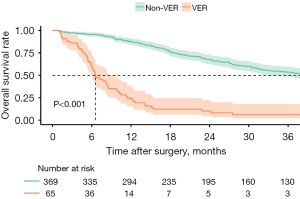
Development and validation of the predictive model of VER
The contributions of the different variables in the XGBoost model to predict VER following the resection of pCCA are presented in Figure 2. Notably, the five most important risk factors predictive of VER included LN metastasis, preoperative CA19-9 value, age, tumor size, and tumor differentiation; AJCC T stage, MVI, severe complications, and PNI were also associated with VER. The discriminative accuracy of the predictive model based on these factors was favorable in both the derivative [c-index: 0.74; 95% confidence interval (CI): 0.67–0.81] and validation (c-index: 0.77; 95% CI: 0.71–0.83) cohorts. Based on the predictive model, patients were categorized into three distinct risk groups using the X-tile program relative to VER: low-risk (n=186, 42.9%, 6-month RFS: 94.6%), intermediate-risk (n=184, 42.4%, 6-month RFS: 88.3%), and high-risk (n=64, 14.7%, 6-month RFS: 40.0%) (log-rank, P<0.001) (Figure 3A); an estimated incidence of recurrence less than 5.7% within 6 months was classified as low risk, while a rate of 25% or more was classified as high risk. Similarly, patients were also stratified relative to OS: low-risk (3-year OS: 75.3%), intermediate-risk (3-year OS; 19.5%), and high-risk (3-year OS; 4.6%) (log-rank, P<0.001) (Figure 3B). To facilitate the clinical applicability of the proposed risk model, an easy-to-use online calculator to predict VER of pCCA patients undergoing curative-intent resection was constructed and made available at: https://junkawashima.shinyapps.io/VER_hilar/ (Figure S1).
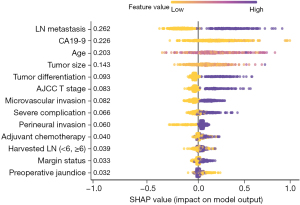
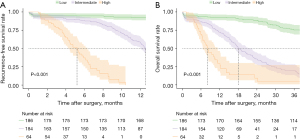
Notably, the predictive accuracy of AJCC staging system (c-index: 0.59, 95% CI: 0.54–0.65) performed poorly compared with the proposed ML model (c-index: 0.74; 95% CI: 0.67–0.81). In fact, the 6-month time-dependent AUC for AJCC staging system was only 0.62 (95% CI: 0.55–0.68) versus 0.80 (95% CI: 0.73–0.86) for the proposed ML VER predictive model (P<0.001) (Figure 4).
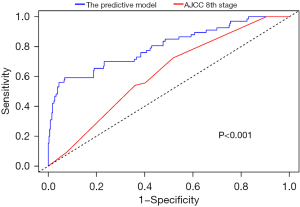
Additional analysis for development and validation of the preoperative predictive model
An additional analysis was conducted to develop a preoperative predictive model using data from 198 patients, among whom 18 (9.1%) individuals experienced VER (Table S2). The contributions of variables in the preoperative model to predict VER were reported in Figure S2. Preoperative CA19-9 level, age, and SII were the three factors most strongly associated with likelihood of VER. The model demonstrated good discriminative accuracy with a c-index of 0.70 (95% CI: 0.59–0.80) in the derivation cohort and 0.69 (95% CI: 0.60–0.79) in the internal validation cohort. Using the X-tile program, patients were stratified into low-risk (n=109, 55%; 6-month RFS: 95.4%, 12-month RFS: 89.0%) versus high-risk (n=89, 45%; 6-month RFS: 83.8%, 12-month RFS: 50.6%) relative to VER (log-rank, P<0.001) (Figure S3A). Patients were also stratified relative to OS: low-risk (3-year OS: 78.7%) and high-risk (3-year OS; 27.5%) (log-rank, P<0.001) (Figure S3B).
Discussion
Recurrence after curative-intent surgery for pCCA can be high with an incidence ranging from 44.0–83.2% (3-5). Unfortunately, recurrence after resection of pCCA can occur early with a subset of patients experiencing recurrent disease within one year after surgery (3-5,7-9). Several previous studies have used the term VER to characterize recurrence within 6 months following resection for ICC, hepatocellular carcinoma (HCC), and colorectal liver metastases (16,19-22). These studies demonstrated poor prognosis of patients with VER, emphasizing the importance of predicting VER in terms of consideration for perioperative systemic chemotherapy, clinical trials, and other non-surgical treatments (16,19,20). However, the oncological outcome and risk factors of VER have not been defined among patients undergoing curative-intent surgery for pCCA. The current study was important because we demonstrated that 15.0% of patients with pCCA had VER and, in turn, a very poor prognosis. We developed and validated a predictive model to calculate risk of VER using a ML approach that leveraged data from a large, international cohort of patients from major Eastern and Western hospitals from around the world. Using this artificial intelligence predictive model, patients were categorized into low-, intermediate-, and high-risk categories who had an incrementally worse 6-month RFS (94.6%, 88.3%, and 40.0%, P<0.001). A preoperative predictive model was also developed that had good discrimination. Furthermore, an online calculator was developed that physicians can easily access to estimate individualized risk of VER in the pre-and postoperative setting. To our knowledge, this is the first study to provide a clinical prediction tool to assess the likelihood of VER among patients undergoing curative-intent surgery for pCCA.
Surgical resection for pCCA is challenging because the tumor arises in an anatomical complex location that involve the bile ducts and abut the hepatic artery and portal vein; many cases require major liver resection with bile duct and portal vein reconstruction, which may impede postoperative recovery and lead to a higher incidence of complications (34). Indeed, a recent systematic review reported a pooled severe morbidity and 90-day mortality among patients who underwent curative intent surgery of 40.0% and 9.0%, respectively (35). In addition, pCCA is a highly aggressive malignancy with a 5-year survival of 8.0–39.7% (3). For patients who undergo curative-intent surgery, early recurrence is associated with a particularly poor prognosis (3,5,35). Zhao et al. reported that patients with recurrence within a year had a median OS of 15 months (95% CI: 12.3–17.7 months) (5). In the current study, 15.0% of patients who underwent curative-intent resection for pCCA recurred within 6 months; these patients had a very poor median OS of 8.4 months and 3-year survival of only 9.2%, which was a worse prognosis than early recurrence related to other malignant diagnoses (5,36). Of note, a recent phase 3 randomized clinical trial demonstrated that patients with advanced biliary tract cancer receiving systemic chemotherapy with gemcitabine and cisplatin, with or without pembrolizumab, had a median OS of 12.7 and 10.9 months, respectively, which was comparable to the outcomes of patients with VER in the current study (37). Given the aggressive nature of pCCA and the substantial surgical risks, it is important to balance the potential benefits of surgery against the likelihood of VER. As such, predicting VER is important in terms of the selection of patients likely to benefit the most from resection. In addition, tools such as the proposed online calculator can help to individualize management of perioperative treatment and surveillance according to the risk of recurrence in the early postoperative period.
In the current study, SHAP values were used to interpret the associations of each clinicopathological factor with the estimated risk of VER for patients with pCCA. Among the prognostic factors for pCCA, patient age, LN metastasis, preoperative CA19-9, tumor size, and tumor differentiation were the most important factors of VER based on the XGBoost based-postoperative predictive model (3,9,29-31). Among these factors, LN metastasis had the highest weight to predict the risk of VER. This finding was in line with a meta-analysis on prognostic factors of early recurrence for pCCA by Tian et al. that demonstrated LN metastasis was the factor most strongly associated with early recurrence (3). Likewise, CA19-9 also demonstrated an important role in prognosis, as noted in previous studies (38,39). CA19-9 is a well-known tumor biomarker, which reflects the aggressive tumor biology in cholangiocarcinoma. Notably, a systematic review of prognostic biomarkers in pCCA demonstrated preoperative CA19-9 was strongly associated with lower OS in ten out of thirteen studies and poorer RFS in four studies (38). In the current study, patients age also was associated with pCCA VER, which was consistent with other studies demonstrating the prognostic effect of age on survival outcomes (16,36,40). Other factors predictive of VER included tumor size and differentiation, which was consistent with other reports (3,29-31). For instance, Zhang et al. demonstrated that prognostic stratification by tumor diameter outperformed AJCC staging system (29). Additionally, a recent meta-analysis noted that poorly differentiated tumors were associated with more than a two-fold increased risk of early recurrence among patients with pCCA (3). Collectively, these pathological factors reflect the malignancy potential of pCCA, and the potential ability to use these factors collectively to more accurately predict VER. In the preoperative predictive model, both age and CA19-9 were clinical factors strongly associated with VER, which was consistent with the postoperative predictive model. Notably, SII, a relatively recent inflammatory biomarker, also was an important predictive factor (33). Inflammation is a crucial driver in tumor biology, promoting extracellular matrix breakdown, cancer progression, immunosuppression, and angiogenesis, all of which support metastatic spread (41,42). Initially identified as a prognostic marker in HCC, SII has since been applied to other gastrointestinal cancers (33). In the field of cholangiocarcinoma, some investigators have demonstrated SII to be a prognostic factor independent of other conventional clinicopathological factors (33,43,44). For instance, Toyoda et al. reported that high SII values were associated with a 1.46-fold increase in recurrence among patients with extrahepatic bile duct cancer (44). Collectively, these findings suggest that SII may capture the inflammatory microenvironment of pCCA tumors and potentially help predict VER risk.
Of note, the XGBoost-based postoperative predictive model demonstrated favorable discriminative accuracy for the entire cohort (c-index: 0.74; 95% CI: 0.67–0.81), as well as the bootstrapped resampled internal cohort (c-index: 0.77; 95% CI: 0.71–0.83). Moreover, the model stratified patients incrementally into three distinct groups relative to VER and OS: low-risk (6-month RFS: 94.6%, 3-year OS: 75.3%), intermediate-risk (6-month RFS: 88.3%, 3-year OS: 19.5%), and high-risk (6-month RFS: 40.0%, 3-year OS: 4.6%) (Figure 3). Traditional simple risk factors such as LN metastasis, CA19-9 levels, and AJCC Staging system may not fully identify risk of VER when used separately as single prognostic indicators (3,23,38,39). Specifically, the real-world clinical environment represents a web of interrelated and confounding factors that may influence patient outcomes in complex ways. To address these reality and achieve a more tailored and accurate prognostic prediction, the use of ML techniques has become increasingly popular (45). The improved performance of the XGBoost model mostly derives from its decision tree architecture, which has the ability to capture independently nuanced relationships among variables (28). Among these ML techniques, the XGBoost statistical approach may be superior to other ML techniques in terms of prediction accuracy (32,46). In fact, XGBoost-based models have been used to predict risk of cardiac surgery-associated acute kidney damage and postoperative pancreatic fistula with high discriminatory accuracy (28,47). In the current study, the proposed XGBoost-based postoperative predictive model outperformed the AJCC 8th staging system with respect to c-index and time-dependent AUC at 6 months after resection (Figure 4). Furthermore, to make this complicated predictive model accessible, an easy-to-use, online calculator was developed. An easy-to-use online calculator can facilitate use by clinicians by allowing the simple input of individual data points with subsequent real-time predictions of VER risk. The ease of use and immediate feedback provided by the calculator may help clinical decision-making, particularly in augmenting surveillance strategies and guiding the use of perioperative chemotherapy.
To assess the VER risk preoperatively, we also developed an XGBoost-based preoperative predictive model. Although the predictive ability was slightly inferior to that of the postoperative predictive model, the preoperative model demonstrated reasonable discriminative accuracy with a c-index of 0.70 (95% CI: 0.59–0.80) in the derivation cohort and 0.69 (95% CI: 0.60–0.79) in the internal validation cohort. Given the aggressive nature of pCCA and the significant surgical risks involved, it may be beneficial to consider preoperative systemic chemotherapy for high-risk VER patients. This approach mirrors the standard practice for pancreatic ductal adenocarcinoma, which also carries a poor prognosis due to its aggressive behavior (48). Preoperative systemic chemotherapy can serve both therapeutic and selection roles, helping to determine which patients are most likely to benefit from curative-intent surgery (16,33). In fact, neoadjuvant therapy has been demonstrated to be effective in increasing disease-free survival among patients with pCCA (49). By providing risk estimates for VER with reasonable accuracy, the predictive tool may assist the multidisciplinary team in decision-making regarding preoperative chemotherapy for pCCA patients, offering valuable guidance in tailoring treatment strategies.
The findings of this current study should be interpreted in light of several limitations. Even though the multi-institutional nature of the database was a strength, there may have been some heterogeneity in patient selection and surgical techniques among the different participating centers. In particular, variations in the indications and types of adjuvant chemotherapy across institutions and over time may have influenced the results. Additionally, while the predictive model for VER performed well in both the training and internal validation cohorts using bootstrapping resampling, future prospective studies should be conducted to standardize surgical indications, techniques, and postoperative management, as well as to validate the proposed models externally. A preoperative prediction model for VER was developed; however, only 198 cases had sufficient preoperative test information, resulting in a relatively small sample size. Future work should aim to build the preoperative prediction model for VER in a larger cohort to enhance its robustness and applicability.
Conclusions
In conclusion, 15% of patients undergoing curative-intent resection for pCCA developed VER, which was associated with a dismal prognosis. Various patient-, tumor-, and treatment-specific factors were associated with VER among patients with pCCA. These factors were utilized to develop ML prediction models to categorize patients into groups relative to risk of VER and survival with high accuracy. Furthermore, an easy-to-use, online calculator was made available to help with the selection of patients likely to benefit the most from resection, as well as counsel individuals about the anticipated risk of recurrence in the early post-operative period.
Acknowledgments
Funding: None.
Footnote
Reporting Checklist: The authors have completed the TRIPOD reporting checklist. Available at https://hbsn.amegroups.com/article/view/10.21037/hbsn-24-385/rc
Data Sharing Statement: Available at https://hbsn.amegroups.com/article/view/10.21037/hbsn-24-385/dss
Peer Review File: Available at https://hbsn.amegroups.com/article/view/10.21037/hbsn-24-385/prf
Conflicts of Interest: All authors have completed the ICMJE uniform disclosure form (available at https://hbsn.amegroups.com/article/view/10.21037/hbsn-24-385/coif). H.P.M. and S.K.M. serve as the unpaid editorial board members of HepatoBiliary Surgery and Nutrition. T.M.P. serves as an unpaid Deputy Editor-in-Chief of HepatoBiliary Surgery and Nutrition. The other authors have no conflicts of interest to declare.
Ethical Statement: The authors are accountable for all aspects of the work in ensuring that questions related to the accuracy or integrity of any part of the work are appropriately investigated and resolved. This study was approved by the Institutional Review Board of The Ohio State University Wexner Medical System (IRB#2108C0025). All participating institutions (Yokohama City University, University of Verona, Curry Cabral Hospital, Emory University, Erasmus University Medical Centre, University of Sydney, Cleveland Clinic, and The Ohio State University Wexner Medical System) were informed and agreed the study. Obtaining informed consent from all individual participants was not required as the study relied on secondary data analysis of de-identified patient data.
Open Access Statement: This is an Open Access article distributed in accordance with the Creative Commons Attribution-NonCommercial-NoDerivs 4.0 International License (CC BY-NC-ND 4.0), which permits the non-commercial replication and distribution of the article with the strict proviso that no changes or edits are made and the original work is properly cited (including links to both the formal publication through the relevant DOI and the license). See: https://creativecommons.org/licenses/by-nc-nd/4.0/.
References
- Gaspersz MP, Buettner S, van Vugt JLA, et al. Evaluation of the New American Joint Committee on Cancer Staging Manual 8th Edition for Perihilar Cholangiocarcinoma. J Gastrointest Surg 2020;24:1612-8.
- Elvevi A, Laffusa A, Scaravaglio M, et al. Clinical treatment of cholangiocarcinoma: an updated comprehensive review. Ann Hepatol 2022;27:100737. [Crossref] [PubMed]
- Tian Y, Wen N, Li B, et al. A meta-analysis of prognostic factors for early recurrence in perihilar cholangiocarcinoma after curative-intent resection. Eur J Surg Oncol 2023;49:106982. [Crossref] [PubMed]
- Liu ZP, Zhang QY, Chen WY, et al. Evaluation of Four Lymph Node Classifications for the Prediction of Survival in Hilar Cholangiocarcinoma. J Gastrointest Surg 2022;26:1030-40. [Crossref] [PubMed]
- Zhao J, Zhang W, Zhu YY, et al. Development and Validation of Noninvasive MRI-Based Signature for Preoperative Prediction of Early Recurrence in Perihilar Cholangiocarcinoma. J Magn Reson Imaging 2022;55:787-802. [Crossref] [PubMed]
- Nassour I, Mokdad AA, Porembka MR, et al. Adjuvant Therapy Is Associated With Improved Survival in Resected Perihilar Cholangiocarcinoma: A Propensity Matched Study. Ann Surg Oncol 2018;25:1193-201. [Crossref] [PubMed]
- Groot Koerkamp B, Wiggers JK, Allen PJ, et al. Recurrence Rate and Pattern of Perihilar Cholangiocarcinoma after Curative Intent Resection. J Am Coll Surg 2015;221:1041-9. [Crossref] [PubMed]
- Yamamoto R, Sugiura T, Ashida R, et al. Preoperative risk factors for early recurrence after resection of perihilar cholangiocarcinoma. BJS Open 2022;6:zrac115. [Crossref] [PubMed]
- Li CX, Zhang H, Wang K, et al. Preoperative Bilirubin Level Predicts Overall Survival and Tumor Recurrence After Resection for Perihilar Cholangiocarcinoma Patients. Cancer Manag Res 2019;11:10157-65. [Crossref] [PubMed]
- Wilson A, Ronnekleiv-Kelly SM, Pawlik TM. Regret in Surgical Decision Making: A Systematic Review of Patient and Physician Perspectives. World J Surg 2017;41:1454-65. [Crossref] [PubMed]
- Wilson A, Winner M, Yahanda A, et al. Factors associated with decisional regret among patients undergoing major thoracic and abdominal operations. Surgery 2017;161:1058-66. [Crossref] [PubMed]
- Bagante F, Spolverato G, Cucchetti A, et al. Defining when to offer operative treatment for intrahepatic cholangiocarcinoma: A regret-based decision curves analysis. Surgery 2016;160:106-17. [Crossref] [PubMed]
- Xu S, Zhang XP, Zhao GD, et al. Development and validation of an online calculator to predict early recurrence and long-term survival in patients with distal cholangiocarcinoma after pancreaticoduodenectomy. J Hepatobiliary Pancreat Sci 2022;29:1214-25. [Crossref] [PubMed]
- Song Y, Zhou G, Zhou Y, et al. Artificial intelligence CT radiomics to predict early recurrence of intrahepatic cholangiocarcinoma: a multicenter study. Hepatol Int 2023;17:1016-27. [Crossref] [PubMed]
- Guo Y, Linn YL, Koh YX, et al. Preoperative Predictors of Early Recurrence After Liver Resection for Multifocal Hepatocellular Carcinoma. J Gastrointest Surg 2023;27:1106-12. [Crossref] [PubMed]
- Tsilimigras DI, Sahara K, Wu L, et al. Very Early Recurrence After Liver Resection for Intrahepatic Cholangiocarcinoma: Considering Alternative Treatment Approaches. JAMA Surg 2020;155:823-31. [Crossref] [PubMed]
- Zhang XF, Beal EW, Bagante F, et al. Early versus late recurrence of intrahepatic cholangiocarcinoma after resection with curative intent. Br J Surg 2018;105:848-56. [Crossref] [PubMed]
- Altaf A, Endo Y, Munir MM, et al. Impact of an artificial intelligence based model to predict non-transplantable recurrence among patients with hepatocellular carcinoma. HPB (Oxford) 2024;26:1040-50. [Crossref] [PubMed]
- Simon R, Sasaki K, Margonis GA, et al. Risk factors for very early recurrence of hepatocellular carcinoma: a retrospective review. HPB (Oxford) 2018;20:2. [Crossref]
- Hirokawa F, Hayashi M, Asakuma M, et al. Risk factors and patterns of early recurrence after curative hepatectomy for hepatocellular carcinoma. Surg Oncol 2016;25:24-9. [Crossref] [PubMed]
- Jung SW, Kim DS, Yu YD, et al. Risk factors for cancer recurrence or death within 6 months after liver resection in patients with colorectal cancer liver metastasis. Ann Surg Treat Res 2016;90:257-64. [Crossref] [PubMed]
- Takahashi S, Konishi M, Nakagohri T, et al. Short time to recurrence after hepatic resection correlates with poor prognosis in colorectal hepatic metastasis. Jpn J Clin Oncol 2006;36:368-75. [Crossref] [PubMed]
- Amin MB, Greene FL, Edge SB, et al. The Eighth Edition AJCC Cancer Staging Manual: Continuing to build a bridge from a population-based to a more "personalized" approach to cancer staging. CA Cancer J Clin 2017;67:93-9.
- Dindo D, Demartines N, Clavien PA. Classification of Surgical Complications. Ann Surg 2004;240:205-13. [Crossref] [PubMed]
- Komaya K, Ebata T, Yokoyama Y, et al. Recurrence after curative-intent resection of perihilar cholangiocarcinoma: analysis of a large cohort with a close postoperative follow-up approach. Surgery 2018;163:732-8. [Crossref] [PubMed]
- van Buuren S, Boshuizen HC, Knook DL. Multiple imputation of missing blood pressure covariates in survival analysis. Stat Med 1999;18:681-94. [Crossref] [PubMed]
- Cao Y, Forssten MP, Sarani B, et al. Development and Validation of an XGBoost-Algorithm-Powered Survival Model for Predicting In-Hospital Mortality Based on 545,388 Isolated Severe Traumatic Brain Injury Patients from the TQIP Database. J Pers Med 2023;13:1401. [Crossref] [PubMed]
- Verma A, Balian J, Hadaya J, et al. Machine Learning-based Prediction of Postoperative Pancreatic Fistula Following Pancreaticoduodenectomy. Ann Surg 2024;280:325-31. [Crossref] [PubMed]
- Zhang XF, Beal EW, Chakedis J, et al. Defining Early Recurrence of Hilar Cholangiocarcinoma After Curative-intent Surgery: A Multi-institutional Study from the US Extrahepatic Biliary Malignancy Consortium. World J Surg 2018;42:2919-29. [Crossref] [PubMed]
- Peng D, Lu J, Hu H, et al. Lymphocyte to Monocyte Ratio Predicts Resectability and Early Recurrence of Bismuth-Corlette Type IV Hilar Cholangiocarcinoma. J Gastrointest Surg 2020;24:330-40. [Crossref] [PubMed]
- Qin H, Hu X, Zhang J, et al. Machine-learning radiomics to predict early recurrence in perihilar cholangiocarcinoma after curative resection. Liver Int 2021;41:837-50. [Crossref] [PubMed]
- Jabeur SB, Mefteh-Wali S, Viviani JL. Forecasting gold price with the XGBoost algorithm and SHAP interaction values. Ann Oper Res 2024;334:679-99. [Crossref]
- Kawashima J, Sahara K, Shen F, et al. Predicting risk of recurrence after resection of stage I intrahepatic cholangiocarcinoma. J Gastrointest Surg 2024;28:18-25. [Crossref] [PubMed]
- van Keulen AM, Buettner S, Erdmann JI, et al. Multivariable prediction model for both 90-day mortality and long-term survival for individual patients with perihilar cholangiocarcinoma: does the predicted survival justify the surgical risk? Br J Surg 2023;110:599-605. [Crossref] [PubMed]
- Franken LC, Schreuder AM, Roos E, et al. Morbidity and mortality after major liver resection in patients with perihilar cholangiocarcinoma: A systematic review and meta-analysis. Surgery 2019;165:918-28. [Crossref] [PubMed]
- Hu HJ, Jin YW, Shrestha A, et al. Predictive factors of early recurrence after R0 resection of hilar cholangiocarcinoma: A single institution experience in China. Cancer Med 2019;8:1567-75. [Crossref] [PubMed]
- Kelley RK, Ueno M, Yoo C, et al. Pembrolizumab in combination with gemcitabine and cisplatin compared with gemcitabine and cisplatin alone for patients with advanced biliary tract cancer (KEYNOTE-966): a randomised, double-blind, placebo-controlled, phase 3 trial. Lancet 2023;401:1853-65. [Crossref] [PubMed]
- Pawaskar R, Huang KZ, Pham H, et al. Systematic Review of Preoperative Prognostic Biomarkers in Perihilar Cholangiocarcinoma. Cancers (Basel) 2024;16:698. [Crossref] [PubMed]
- Hu HJ, Mao H, Shrestha A, et al. Prognostic factors and long-term outcomes of hilar cholangiocarcinoma: A single-institution experience in China. World J Gastroenterol 2016;22:2601-10. [Crossref] [PubMed]
- Ito K, Ito H, Allen PJ, et al. Adequate lymph node assessment for extrahepatic bile duct adenocarcinoma. Ann Surg 2010;251:675-81. [Crossref] [PubMed]
- Sparmann A, Bar-Sagi D. Ras-induced interleukin-8 expression plays a critical role in tumor growth and angiogenesis. Cancer Cell 2004;6:447-58. [Crossref] [PubMed]
- Coussens LM, Werb Z. Inflammation and cancer. Nature 2002;420:860-7. [Crossref] [PubMed]
- Tsilimigras DI, Moris D, Mehta R, et al. The systemic immune-inflammation index predicts prognosis in intrahepatic cholangiocarcinoma: an international multi-institutional analysis. HPB (Oxford) 2020;22:1667-74. [Crossref] [PubMed]
- Toyoda J, Sahara K, Maithel SK, et al. Prognostic Utility of Systemic Immune-Inflammation Index After Resection of Extrahepatic Cholangiocarcinoma: Results from the U.S. Extrahepatic Biliary Malignancy Consortium. Ann Surg Oncol 2022;29:7605-14. [Crossref] [PubMed]
- Tsilimigras DI, Mehta R, Moris D, et al. A Machine-Based Approach to Preoperatively Identify Patients with the Most and Least Benefit Associated with Resection for Intrahepatic Cholangiocarcinoma: An International Multi-institutional Analysis of 1146 Patients. Ann Surg Oncol 2020;27:1110-9. [Crossref] [PubMed]
- Xie L, Liu J, Lu S, et al. An Efficient Learning Framework for Federated XGBoost Using Secret Sharing and Distributed Optimization. ACM Trans Intell Syst Technol 2022;13:77. [Crossref]
- Tseng PY, Chen YT, Wang CH, et al. Prediction of the development of acute kidney injury following cardiac surgery by machine learning. Crit Care 2020;24:478. [Crossref] [PubMed]
- Motoi F, Kosuge T, Ueno H, et al. Randomized phase II/III trial of neoadjuvant chemotherapy with gemcitabine and S-1 versus upfront surgery for resectable pancreatic cancer (Prep-02/JSAP05). Jpn J Clin Oncol 2019;49:190-4. [Crossref] [PubMed]
- Darwish Murad S, Kim WR, Harnois DM, et al. Efficacy of neoadjuvant chemoradiation, followed by liver transplantation, for perihilar cholangiocarcinoma at 12 US centers. Gastroenterology 2012;143:88-98.e3; quiz e14. [Crossref] [PubMed]