Artificial intelligence is poised to revolutionize human liver allocation and decrease medical costs associated with liver transplantation
The term artificial intelligence originated in 1956, when a group of computer and research scientists gathered for a 2-month study meeting, announced as the “Dartmouth Research Project on Artificial Intelligence”, to philosophize about what would ultimately become the field of AI (1). The conference instantly succeeded in defining the mathematical models behind the concept of AI and inspired a plethora of areas of research in the following decades. In fact, applications of AI were growing exponentially and in 2016 healthcare related AI-projects attracted more investments, than AI-projects within any other sector of the global economy.
In 2019, within the Eurotransplant (ET) region, 13,985 patients were actively listed for a lifesaving organ transplant. Out of these patients, 1,417 were scheduled for liver transplantation. Due to organ shortage, 383 potential liver transplant candidates died while on the waiting list, because no life-saving organ became available for them in time (source: eutotransplant.gov).
There are few reasonable strategies, to fill-up the increasing gap between organ demand and availability in Europe. Besides the propagation of living donation and the utilization of marginal organs, and organs donated after cardiac death (DCD), organ regeneration in combination with machine perfusion has been recently called into service (2). Limited resources must be handled with care. As a matter of fact, reduced organ availability and increased demand also make a reconsideration of the current organ allocation strategy in Europe necessary.
At the moment, liver allocation follows the principle of “sickest first”, which means that patients with serious liver injury receive a preferential transplant. The severity of liver disease is thereby determined by the Model of End Stage Liver Disease (MELD) score, which has been shown to be a good predictor of 3-month patient survival while waiting for a liver transplant. However, the application of MELD scoring has also been shown to lack prognosis of patient and graft survival, following liver transplantation (3). Currently, 1-, 3- and 10-year graft survival for a liver transplant within the ET region is 77%, 70% and 55%, respectively (4). One reason for these sobering numbers, especially for early timepoints after transplantation, might be the fact that donor-recipient matching cannot always be performed with foresight and goals for long term success. And this in turn occurs, because patients frequently have reached a state of disease, where transplant surgeons are forced to accept the next available graft, independent of quality and fit.
Under these circumstances it is valid to reflect, if a distribution of organs according to a “best benefit” strategy would better fit into this dilemma. This could mean that a severely ill patient, confirmed by a high MELD score, listed on “pole position” on the liver transplant waiting list, would not get the next available organ offer, while an almost equally sick patient, listed as “runner up” receives the live saving organ and subsequent transplant instead, just because of higher graft survival probability and better outcome probability. These measures at first sight would not increase the total number of transplants, since they do not increase the donor pool, however these measures would decrease the number of re-transplant procedures, and organs saved with this strategy could be used for first liver transplants instead.
In this context, strategies aiming to predict solid organ allograft survival, using artificial intelligence (AI) based computational forecasting have been recently applied (5). The hypothetical use of AI for donor-recipient matching in a large Spanish multicenter trial (6), which was subsequently validated in a liver transplant cohort from King’s College Hospital (7), demonstrated impressive theoretical superiority over currently used allocation strategies, especially in terms of graft survival.
Liver transplantation is an expensive endeavor and from both, a medical and an economic standpoint demands low complication rates. Total costs for a liver transplant procedure range from 80,000 to 120,000 Euros, depending on the ET country and region. The postoperative course of a liver transplant patient furthermore, has a crucial impact on healthcare expenses. Good early graft function and short demand for postoperative intensive care, make a liver transplant procedure profitable for the health care institution. High postoperative morbidity, early graft loss, and worst case, the necessity of re-transplantation, irrespective of the underlying personal tragedy, drastically increases costs and commonly leaves behind a relevant budgetary deficit (Figure 1).
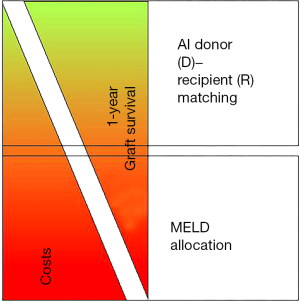
Decisions made by artificial intelligence prevail all major sectors of modern civilization including Agriculture, Science, Economy and Medicine. A technology that not only can outperform but also outthink its creators has created a certain amount of unease, however from both a medical and economic standpoint it is important to continue to think and discuss about the potential fact that one time software might decide who will get a graft and who will not, and thus will die (8).
Acknowledgments
We acknowledge support from the German Research Foundation (DFG) and Leipzig University within the program of Open Access Publishing.
Funding: None.
Footnote
Provenance and Peer Review: This article is a free submission to the editorial office, Hepatobiliary Surgery and Nutrition. The article did not undergo external peer review.
Conflicts of Interest: Both authors have completed the ICMJE uniform disclosure form (available at https://hbsn.amegroups.com/article/view/10.21037/hbsn-20-458/coif). The authors have no conflicts of interest to declare.
Ethical Statement: The authors are accountable for all aspects of the work in ensuring that questions related to the accuracy or integrity of any part of the work are appropriately investigated and resolved.
Open Access Statement: This is an Open Access article distributed in accordance with the Creative Commons Attribution-NonCommercial-NoDerivs 4.0 International License (CC BY-NC-ND 4.0), which permits the non-commercial replication and distribution of the article with the strict proviso that no changes or edits are made and the original work is properly cited (including links to both the formal publication through the relevant DOI and the license). See: https://creativecommons.org/licenses/by-nc-nd/4.0/.
References
- McCarthy J, Minsky M, Rochester N, et al. A Proposal for the Dartmouth Summer Research Project on Artificial Intelligence. Available online: http://raysolomonoffcom/dartmouth/boxa/dart564propspdf 1955.
- Schneeberger S. Life of a liver awaiting transplantation. Nature 2018;557:40-1. [Crossref] [PubMed]
- Desai NM, Mange KC, Crawford MD, et al. Predicting outcome after liver transplantation: utility of the model for end-stage liver disease and a newly derived discrimination function. Transplantation 2004;77:99-106. [Crossref] [PubMed]
- Adam R, Karam V, Delvart V, et al. Evolution of indications and results of liver transplantation in Europe. A report from the European Liver Transplant Registry (ELTR). J Hepatol 2012;57:675-88. [Crossref] [PubMed]
- Briceño J. Artificial intelligence and organ transplantation: challenges and expectations. Curr Opin Organ Transplant 2020;25:393-8. [Crossref] [PubMed]
- Briceno J, Cruz-Ramirez M, Prieto M, et al. Use of artificial intelligence as an innovative donor-recipient matching model for liver transplantation: results from a multicenter Spanish study. J Hepatol 2014;61:1020-8. [Crossref] [PubMed]
- Ayllon MD, Ciria R, Cruz-Ramirez M, et al. Validation of artificial neural networks as a methodology for donor-recipient matching for liver transplantation. Liver Transpl 2018;24:192-203. [Crossref] [PubMed]
- Wingfield LR, Ceresa C, Thorogood S, et al. Artificial Intelligence and Liver Transplant: Predicting Survival of Individual Grafts. Liver Transpl 2020;26:922-34. [Crossref] [PubMed]