A novel radiomics signature based on T2-weighted imaging accurately predicts hepatic inflammation in individuals with biopsy-proven nonalcoholic fatty liver disease: a derivation and independent validation study
Introduction
Nonalcoholic fatty liver disease (NAFLD), which affects about 25% of the world’s adult population, has become one of the most common causes of chronic liver disease, representing a considerable health concern and an economic burden to several societies (1,2). The severity, assessment and risk stratification of people with NAFLD is currently best undertaken by assessment of liver histology obtained by biopsy (3). It is well established that hepatic inflammation can promote fibrogenesis that may eventually develop into cirrhosis or hepatocellular carcinoma, leading to poor patient outcomes (4,5). Thus, evaluation of hepatic inflammatory activity is important for effective clinical decisions and to monitor therapeutic responses in NAFLD. Currently, liver biopsy remains the ‘gold standard’ for diagnosing NAFLD and for accurately staging the severity of hepatic steatosis, hepatocellular ballooning, lobular inflammation, and fibrosis (6). In addition, liver biopsy is the only method for diagnosing hepatic inflammation in NAFLD [i.e., nonalcoholic steatohepatitis (NASH)]. However, it should be noted that undertaking a liver biopsy is costly, risky, and potentially painful (7). Moreover, frequent monitoring by performing multiple liver biopsies during management is not feasible, ethical, nor acceptable to patients.
Several studies have explored a variety of serum biomarkers [for example, the aspartate aminotransferase (AST)-to-platelet ratio index (APRI) or the fibrosis-4 index (FIB-4)] and imaging techniques [for example, vibration-controlled transient elastography, magnetic resonance imaging (MRI) proton density fat fraction, magnetic resonance spectroscopy, magnetic resonance elastography or multiparametric MRI], for the assessment of hepatic steatosis and fibrosis, which have acceptable/good diagnostic performances as non-invasive alternatives to liver biopsy (8-15). However, to date, there are no non-invasive biomarkers or imaging techniques that can accurately assess hepatic inflammation in NAFLD.
Serum levels of alanine aminotransferase (ALT) are commonly used as a non-invasive biomarker for assessing hepatic dysfunction/inflammation in clinical practice. However, it is well known that a significant number of people with NAFLD have normal serum ALT levels, and yet may have histologically severe hepatic inflammation (16).
Quantitative analysis of intravoxel-incoherent motion diffusion-weighted MRI has been used for the non-invasive assessment of hepatic inflammation in some studies, but its effectiveness remains controversial (17,18). Moreover, multiparametric MRI composed of T1 mapping and T2* mapping has been proposed for the assessment of hepatic inflammatory activity, and has good diagnostic performance (19). However, the special post-processing software and uncommon sequences of multiparametric MRI, largely limit its wider use in clinical practice.
In 2012, Lambin et al. (20) proposed the approach of radiomics, which allows the extraction of numerous quantitative features from radiographic medical imaging. Although radiomics is mostly used to analyze tumors or tumor-like lesions, it can also be applied to non-neoplastic diseases (21). Recent radiomics techniques have shown excellent capability to non-invasively assess liver fibrosis (22,23). However, to the best of our knowledge, there are very few studies that have investigated whether radiomics techniques can also be used to accurately assess hepatic inflammation (24). T2-weighted imaging (T2WI) is a widely available sequence, which is a part of the output from routine upper abdomen MRI scans. The T2 relaxation time/signal intensity of T2WI may correlate with inflammation in the liver (25,26) and radiomics may offer a promising approach for the quantitative assessment of hepatic inflammation.
Thus, the aim of our observational study was to establish a radiomics signature for hepatic inflammation based on routine T2WI in individuals with NAFLD, who had also undergone liver biopsies (i.e., the gold standard) for the assessment of hepatic inflammation. We present the following article in accordance with the TRIPOD reporting checklist (available at https://hbsn.amegroups.com/article/view/10.21037/hbsn-21-23/rc).
Methods
Study population and design
A total of 234 Chinese adult individuals with NAFLD from two independent cohorts, who underwent both MRI and liver biopsy, were included in this study (Figure S1). Subjects from a well-characterized prospective cohort (16) from the First Affiliated Hospital of Wenzhou Medical University were enrolled for the development of a radiomics signature. The Wenzhou cohort of NAFLD patients (n=152) was randomly assigned to the training or internal validation sets in a 7:3 ratio (27). An independent cohort of NAFLD patients (n=82) from the Guangdong Province Traditional Chinese Medical Hospital was used for external validation. The exclusion criteria for both patient cohorts were as follows: (I) poor quality of MRI images; (II) a time interval between liver biopsy and MRI of greater than 2 months; and (III) concomitant viral hepatitis. The study was conducted in accordance with the Declaration of Helsinki (as revised in 2013). The study was approved by the local ethics board of the First Affiliated Hospital of Wenzhou Medical University and Guangdong Provincial Hospital of Chinese Medicine (No. 2016-246) and informed consent was taken from all individual participants.
Clinical and biochemical data
Clinical and biochemical data were obtained from all participants on the same day as the liver biopsy. All blood samples were taken in the morning after an overnight fast. Hypertension was diagnosed as either systolic blood pressure ≥130 mmHg or diastolic blood pressure ≥85 mmHg and/or use of any anti-hypertensive drugs. Presence of type 2 diabetes was diagnosed as either a fasting glucose level ≥7.0 mmol/L, glycosylated hemoglobin ≥6.5% (≥48 mmol/mol), a history of self-reported diabetes, and/or by the use of any glucose-lowering drugs. Biochemical parameters, including serum liver enzyme levels (ALT, AST, γ-glutamyltranspeptidase), total bilirubin, albumin, glucose, insulin, glycosylated hemoglobin, triglycerides, total cholesterol, high-density lipoprotein cholesterol (HDL-C) and low-density lipoprotein cholesterol (LDL-C), were measured in each participant.
Liver histology
Percutaneous liver biopsies were performed under the guidance of ultrasound control. All liver biopsy samples were examined by a trained histopathologist from each center. Liver biopsies were assessed for steatosis (grade 0 to 3), ballooning (grade 0 to 2) and lobular inflammation (grade 0 to 3), according to the NASH-Clinical Research Network Scoring System (6). Liver fibrosis was staged (from 0 to 4) according to the Brunt’s histologic criteria (28). NAFLD was defined as the presence of hepatic steatosis in more than 5% of hepatocytes. The NAFLD activity score (NAS) was defined as the unweighted sum of the histologic scores for steatosis, ballooning, and lobular inflammation (6). Definitive NASH was histologically diagnosed on the basis of hepatic steatosis, lobular inflammation, and ballooning with NAS ≥5 (6). A hepatic inflammatory activity score (IAS) was calculated as the unweighted sum of the histological scores for ballooning and lobular inflammation (29). According to their histological degrees of IAS, all participants were arbitrarily categorized into two subgroups, as having either mild hepatic inflammatory activity (MIA, defined as IAS ≤2) or severe inflammatory activity (SIA, defined as IAS >2), respectively.
Perls staining of liver tissue was undertaken in 114 (75% of total) individuals from the Wenzhou cohort to measure hepatic iron content. The grade of hepatic iron staining (grade 0 to 4) was assessed visually by microscopy following the method of Searle et al. (30). Liver biopsies from the Guangzhou cohort were not available for hepatic iron assessment.
MRI acquisition
MRI images of the Wenzhou cohort were carried out on GE Discovery MR750 3.0T scanner (GE Healthcare, Waukesha, WI, USA) equipped with an 8-channel abdominal array coil. MRI images from the Guangzhou cohort were carried out on four kinds of 3.0T scanners, (i.e., Toshiba Vantage Titan, GE SIGNA excite, Siemens MAGNETOM Verio, or Siemens MAGNETOM Prisma). A fat-suppressed fast spin-echo sequence was used for acquiring axial T2WI. Main parameters of the axial T2WI sequences are listed in Table S1. All participants were asked to fast for at least 4 hours before MRI scanning and to hold their breath during the scanning procedures.
Radiomics features: extraction and selection
Original MRI data were preprocessed by intensity normalization and isotropic resampling to a voxel size of 1×1×1 mm. Then, a ‘10×10 pixel’ square region of interest (ROI) was placed on the right liver lobe of axial T2WI, avoiding hepatic vessels, focal lesions (such as cysts and hemangiomas), and artifacts in each subject, while using the ITK-SNAP version 3.8 software (https://www.itksnap.org/). Radiomics features, including 18 first-order features; 24 gray-level co-occurrence matrix (GLCM) features; 16 gray-level run length matrix (GLRLM) features; 16 gray-level size zone matrix (GLSZM) features; 14 gray-level dependence matrix (GLDM) features, and 5 neighboring gray-tone difference matrix (NGTDM) features; were calculated from the ROI area of original T2WI and its derived images (Laplacian of Gaussian, wavelet and local binary pattern), by using the AK software version 3.3.0.R (Artificial Intelligence Kit, GE Healthcare). In total, 1,032 imaging features were extracted for each participant.
Before feature selection, all of the extracted radiomics features were standardized. The method of minimum redundancy maximum relevance (mRMR), together with the least absolute shrinkage and selection operator (LASSO), were used to select the radiomics features. At first, mRMR was undertaken to eliminate the redundant and irrelevant features, and 20 features were preliminarily selected. Subsequently, the LASSO was performed to further choose the optimized subset of radiomics features and to evaluate the corresponding coefficients for these radiomics features.
Statistical analysis
Continuous variables were expressed as means ± standard deviation (SD) or medians with interquartile ranges (IQRs). Categorical variables were expressed as numbers (percentages). The unpaired Student’s t-test or the Mann-Whitney U test as appropriate were undertaken for comparison of continuous variables, whereas the chi-squared test or the Fisher’s exact test as appropriate were undertaken for comparison of categorical variables. A radiomics signature based on T2WI (T2-RS), referred to as T2-RS, was calculated by adding the selected radiomics features, weighted by their coefficients for each subject. Receiver operating characteristic (ROC) curve analysis was performed for the binary classification and calculation of the area under the ROC curve (AUROC), sensitivity, specificity, accuracy, positive predictive value (PPV), negative predictive value (NPV), positive likelihood ratio (LR+), and negative likelihood ratio (LR−); diagnostic odds ratios were also calculated to quantify the predictive performance of the T2-RS, in both the training and validation cohorts. The model calibration was evaluated using the Hosmer-Lemeshow goodness of fit test. All calculations and statistical tests were performed in R version 3.6.3 (http://www.r-project.org/). A two-sided P value <0.05 was considered to be statistically significant.
Results
Patients’ characteristics
From the initially enrolled cohort of 234 middle-aged individuals with biopsy-proven NAFLD, 31 subjects were excluded (i.e., 25 because of poor quality MRI images, 5 subjects for concomitant viral hepatitis, and one subject for a time interval between MRI and liver biopsy of more than 2 months). Therefore, 203 individuals with biopsy-proven NAFLD were included in the final analysis (Figure S1).
Table S2 summarizes the baseline characteristics of the Wenzhou cohort of NAFLD patients. Their mean age was 42.2±12 years and 72.1% of them were men. The prevalence of both type 2 diabetes and hypertension was around 16%. Overall, as shown in Table 1, in the Wenzhou cohort there were 70 subjects with MIA and 59 subjects with SIA. The mean age for MIA and SIA groups was 41.7±10.4 and 42.7±13.7 years, respectively. The proportion of men in the MIA group was 81.4% and in the SIA group 61.0% (P=0.01). As also shown in this table, most of the laboratory parameters did not differ significantly between the two groups of patients, except for serum aminotransferase, insulin and triglyceride levels.
Table 1
Characteristics | Wenzhou cohort | Guangzhou cohort | |||||
---|---|---|---|---|---|---|---|
MIA (n=70) | SIA (n=59) | P value | MIA (n=18) | SIA (n=56) | P value | ||
Demographics | |||||||
Age, years | 41.7±10.4 | 42.7±13.7 | 0.638 | 38.2±6.6 | 43.1±11.7 | 0.028 | |
Male sex, n (%) | 57 (81.4) | 36 (61.0) | 0.010 | 15 (83.3) | 36 (64.3) | 0.129 | |
Metabolic factors | |||||||
Type 2 diabetes, n (%) | 15 (21.4) | 6 (10.3) | 0.092 | 0 (0.0) | 7 (12.5) | 0.042 | |
Hypertension, n (%) | 10 (14.3) | 11 (19.0) | 0.477 | 1 (5.6) | 5 (8.9) | 0.636 | |
Laboratory parameters | |||||||
ALT, IU/L | 46.0 (26.8–74.0) | 60.0 (30.0–144.0) | 0.020 | 38.5 (26.5–56.8) | 42.5 (29.1–82.8) | 0.566 | |
AST, IU/L | 32.0 (24.8–46.5) | 49.0 (28.0–71.0) | 0.007 | 24.5 (19.0–31.0) | 30.0 (20.0–40.5) | 0.273 | |
γ-glutamyltranspeptidase, IU/L | 50.5 (29.0–76.5) | 57.0 (35.0–110.0) | 0.172 | 45.5 (25.0–84.8) | 45.5 (31.0–73.3) | 0.719 | |
Albumin, g/L | 47.2 (45.0–50.8) | 46.5 (44.7–48.8) | 0.194 | 48.8 (46.9–50.4) | 47.5 (45.0–50.0) | 0.108 | |
Total bilirubin, μmol/L | 12.0 (10.0–15.0) | 12.0 (10.0–19.0) | 0.538 | 12.2 (8.2–15.3) | 11.7 (9.5–14.7) | 0.980 | |
Fasting glucose, mmol/L | 5.1 (4.9–6.0) | 5.4 (4.8–6.2) | 0.319 | 5.1 (4.6–5.5) | 5.1 (4.8–5.9) | 0.537 | |
Fasting insulin, pmol/L | 92.2 (63.5–147.8) | 117.3 (89.0–157.0)a | 0.033 | 73.6 (63.2–94.8)d | 86.2 (65.3–112.4)e | 0.261 | |
Glycosylated hemoglobin, % | 5.6 (5.3–6.3)b | 5.8 (5.4–6.2)c | 0.500 | 5.6 (5.4–6.0)f | 5.6 (5.4–6.2)g | 0.500 | |
Total cholesterol, mmol/L | 5.1±1.1 | 5.3±1.2 | 0.187 | 5.0±1.1 | 5.1±1.1 | 0.779 | |
Triglyceride, mmol/L | 1.7 (1.2–2.3) | 2.3 (1.6–3.3) | 0.002 | 1.4 (1.0–2.7) | 1.8 (1.3–2.6) | 0.236 | |
HDL-C, mmol/L | 1.0±0.2 | 1.0±0.2 | 0.107 | 1.1 (1.0–1.4) | 1.1 (0.9–1.3) | 0.659 | |
LDL-C, mmol/L | 3.1±0.9 | 3.0±0.9 | 0.729 | 3.3±1.3 | 3.4±1.0 | 0.587 | |
Liver histology features | |||||||
Fibrosis stage, n (%) | <0.001 | 0.026 | |||||
F0 | 32 (45.7) | 7 (11.9) | 1 (5.6) | 0 (0.0) | |||
F1 | 29 (41.4) | 32 (54.2) | 17 (94.4) | 45 (80.4) | |||
F2 | 7 (10.0) | 15 (25.4) | 0 (0.0) | 8 (14.3) | |||
F3 | 1 (1.4) | 3 (5.1) | 0 (0.0) | 3 (5.4) | |||
F4 | 1 (1.4) | 2 (3.4) | 0 (0.0) | 0 (0.0) | |||
Steatosis grade, n (%) | 0.006 | 0.022 | |||||
S0 | 5 (7.1) | 2 (3.4) | 4 (22.2) | 1 (1.8) | |||
S1 | 29 (41.4) | 12 (20.3) | 9 (50.0) | 22 (39.3) | |||
S2 | 24 (34.3) | 27 (45.8) | 3 (16.7) | 31 (55.4) | |||
S3 | 12 (17.1) | 18 (30.5) | 2 (11.1) | 2 (3.6) | |||
Ballooning grade, n (%) | <0.001 | <0.001 | |||||
B0 | 17 (24.3) | 0 (0.0) | 2 (11.1) | 0 (0.0) | |||
B1 | 52 (74.3) | 20 (33.9) | 16 (88.9) | 7 (12.5) | |||
B2 | 1 (1.4) | 39 (66.1) | 0 (0.0) | 49 (87.5) | |||
Lobular inflammation grade, n (%) | <0.001 | 0.007 | |||||
L0 | 9 (12.9) | 0 (0.0) | 1 (5.6) | 0 (0.0) | |||
L1 | 57 (81.4) | 17 (28.8) | 17 (94.4) | 42 (75.0) | |||
L2 | 4 (5.7) | 38 (64.4) | 0 (0.0) | 14 (25.0) | |||
L3 | 0 (0.0) | 4 (6.8) | 0 (0.0) | 0 (0.0) | |||
Definitive NASH‡ | 10 (14.3) | 47 (79.7) | <0.001 | 2 (11.1) | 34 (60.7) | <0.001 |
Continuous variables were expressed as means ± SD or medians with IQRs; categorical variables were expressed as number (percentages). a, 57 (96.6%) cases were available; b, 68 (97.1%) cases were available; c, 58 (98.3%) cases were available; d, 9 (50.0%) cases were available; e, 39 (69.6%) cases were available; f, 15 (83.3%) cases were available; g, 51 (91.1%) cases were available; ‡, definitive NASH was defined as the presence of steatosis, lobular inflammation and ballooning with NAS score ≥5. MIA, mild hepatic inflammatory activity; SIA, severe inflammation activity; ALT, alanine aminotransferase; AST, aspartate aminotransferase; HDL-C, high-density lipoprotein cholesterol; LDL-C, low-density lipoprotein cholesterol; NASH, nonalcoholic steatohepatitis; SD, standard deviation; IQRs, interquartile ranges; NAS, NAFLD activity score; NAFLD, nonalcoholic fatty liver disease.
Development of T2-RS
According to a ratio of 7:3 (27), 91 subjects from the Wenzhou cohort were randomly assigned to the training set. Their mean age was 43.1±12.5 years and they were predominantly men (72.5%). Sixteen point seven percent of these individuals had type 2 diabetes and 20% of them had hypertension (Table 2). The mean age of the MIA and SIA groups were not significantly different, but the proportion of men differed between the two groups (81.6% vs. 61.9%, P=0.036). Similarly, most of the laboratory parameters were not significantly different between the groups, except for serum AST and triglyceride levels (Table 3).
Table 2
Characteristics | Wenzhou cohort | Guangzhou cohort | ||||
---|---|---|---|---|---|---|
Training (n=91) | Internal validation (n=38) | P value* | External validation (n=74) | P value# | ||
Demographics | ||||||
Age, years | 43.1±12.5 | 39.9±10.5 | 0.168 | 41.9±10.9 | 0.528 | |
Male sex, n (%) | 66 (72.5) | 27 (71.1) | 0.865 | 51 (68.9) | 0.612 | |
Metabolic factors | ||||||
Type 2 diabetes, n (%) | 15 (16.7)a | 6 (15.8) | 0.903 | 7 (9.5) | 0.178 | |
Hypertension, n (%) | 18 (20.0)a | 3 (7.9) | 0.091 | 6 (8.1) | 0.032 | |
Laboratory parameters | ||||||
ALT, IU/L | 48.0 (27.0–91.0) | 58.0 (31.5–88.5) | 0.693 | 41.9 (28.3–69.8) | 0.187 | |
AST, IU/L | 37.0 (26.0–58.0) | 32.5 (26.0–53.5) | 0.733 | 28.0 (20.0–38.3) | <0.001 | |
γ-glutamyltranspeptidase, IU/L | 53.0 (34.0–81.0) | 54.5 (29.0–96.5) | 0.681 | 45.5 (29.5–74.0) | 0.196 | |
Albumin, g/L | 46.6 (44.3–49.8) | 47.3 (45.3–49.7) | 0.168 | 47.9 (45.3–50.1) | 0.170 | |
Total Bilirubin, μmol/L | 12.0 (10.0–16.0) | 13.5 (11.0–21.0) | 0.035 | 11.8 (9.4–14.8) | 0.434 | |
Fasting glucose, mmol/L | 5.3 (4.9–6.2) | 5.1 (4.7–6.0) | 0.282 | 5.1 (4.5–5.5) | 0.148 | |
Fasting insulin, pmol/L | 111.1 (76.7–157.4)b | 105.6 (75.6–152.2) | 0.897 | 83.5 (65.3–105.8)c | 0.007 | |
Glycosylated hemoglobin, % | 5.6 (5.4–6.3)d | 5.5 (5.2–6.2) | 0.165 | 5.6 (5.4–6.0)e | 0.470 | |
Total cholesterol, mmol/L | 5.4±1.1 | 4.7±1.0 | 0.001 | 5.1±1.1 | 0.084 | |
Triglyceride, mmol/L | 2.0 (1.4–3.1) | 1.6 (1.2–3.2) | 0.027 | 1.7 (1.2–2.6) | 0.073 | |
HDL-C, mmol/L | 1.0 (0.9–1.1) | 0.9 (0.8–1.1) | 0.244 | 1.1 (1.0–1.3) | 0.001 | |
LDL-C, mmol/L | 3.2±0.9 | 2.8±0.8 | 0.063 | 3.4±1.1 | 0.168 | |
Liver histology features | ||||||
Fibrosis stage, n (%) | 0.830 | 0.184 | ||||
F0 | 29 (31.9) | 10 (26.3) | 1 (1.4) | |||
F1 | 41 (45.1) | 20 (52.6) | 62 (83.8) | |||
F2 | 15 (16.5) | 7 (18.4) | 8 (10.8) | |||
F3 | 3 (3.3) | 1 (2.6) | 3 (4.1) | |||
F4 | 3 (3.3) | 0 (0.0) | 0 (0.0) | |||
Steatosis grade, n (%) | 0.297 | 0.006 | ||||
S0 | 5 (5.5) | 2 (5.3) | 5 (6.8) | |||
S1 | 29 (31.9) | 12 (31.6) | 31 (41.9) | |||
S2 | 31 (34.1) | 20 (52.6) | 34 (45.9) | |||
S3 | 26 (28.6) | 4 (10.5) | 4 (5.4) | |||
Ballooning grade, n (%) | 0.594 | <0.001 | ||||
B0 | 11 (12.1) | 6 (15.8) | 2 (2.7) | |||
B1 | 51 (56.0) | 21 (55.3) | 23 (31.1) | |||
B2 | 29 (31.9) | 11 (28.9) | 49 (66.2) | |||
Lobular inflammation grade, n (%) | 0.982 | 0.096 | ||||
L0 | 5 (5.5) | 4 (10.5) | 1 (1.4) | |||
L1 | 55 (60.4) | 19 (50.0) | 59 (79.7) | |||
L2 | 28 (30.8) | 14 (36.8) | 14 (18.9) | |||
L3 | 3 (3.3) | 1 (2.6) | 0 (0.0) | |||
Definitive NASH‡ | 39 (42.9) | 18 (47.4) | 0.638 | 36 (48.6) | 0.457 | |
Hepatic IAS† | 0.731 | 0.030 | ||||
0 | 2 (2.2) | 2 (5.3) | 0 (0.0) | |||
1 | 8 (8.8) | 5 (13.2) | 3 (4.1) | |||
2 | 39 (42.9) | 14 (36.8) | 16 (21.6) | |||
3 | 26 (28.6) | 9 (23.7) | 47 (63.5) | |||
4 | 15 (16.5) | 7 (18.4) | 8 (10.8) | |||
5 | 1 (1.1) | 1 (2.6) | 0 (0.0) |
Continuous variables were expressed as means ± SD or medians with IQRs; categorical variables were expressed as number (percentages). a, 90 (98.9%) cases were available; b, 89 (97.8%) cases were available; c, 48 (64.9%) cases were available; d, 88 (96.7%) cases were available; e, 66 (89.2%) cases were available; ‡, definitive NASH was defined as the presence of steatosis, lobular inflammation and ballooning with NAS score ≥5; †, hepatic IAS was defined as the sum of the histologic scores for lobular inflammation and ballooning; *, training vs. internal validation; #, training vs. external validation. NAFLD, nonalcoholic fatty liver disease; ALT, alanine aminotransferase; AST, aspartate aminotransferase; HDL-C, high-density lipoprotein cholesterol; LDL-C, low-density lipoprotein cholesterol; NASH, nonalcoholic steatohepatitis; IAS, inflammatory activity score; SD, standard deviation; IQRs, interquartile ranges; NAS, NAFLD activity score.
Table 3
Characteristics | Training set | Internal validation set | |||||
---|---|---|---|---|---|---|---|
MIA (n=49) | SIA (n=42) | P value | MIA (n=21) | SIA (n=17) | P value | ||
Demographics | |||||||
Age, years | 42.7±10.6 | 43.6±14.5 | 0.746 | 39.3±9.7 | 40.6±11.7 | 0.720 | |
Male sex, n (%) | 40 (81.6) | 26 (61.9) | 0.036 | 17 (81.0) | 10 (58.8) | 0.134 | |
Metabolic factors | |||||||
Type 2 diabetes, n (%) | 10 (20.4) | 5 (12.2) | 0.298 | 5 (23.8) | 1 (5.9) | 0.115 | |
Hypertension, n (%) | 8 (16.3) | 10 (24.4) | 0.341 | 2 (9.5) | 1 (5.9) | 0.675 | |
Laboratory parameters | |||||||
ALT, IU/L | 44.0 (25.5–67.0) | 60.5 (30.0–145.5) | 0.020 | 62.0 (31.0–87.0) | 54.0 (30.5–155.5) | 0.486 | |
AST, IU/L | 32.0 (23.0–43.0) | 49.0 (28.8–71.5) | 0.004 | 31.0 (26.5–50.0) | 33.0 (23.0–75.5) | 0.561 | |
γ-glutamyltranspeptidase, IU/L | 47.0 (29.0–66.5) | 60.5 (36.0–111.8) | 0.051 | 55.0 (31.0–97.0) | 39.0 (21.0–97.5) | 0.642 | |
Albumin, g/L | 46.6 (44.4–50.3) | 46.6 (44.1–49.2) | 0.676 | 48.2 (46.2–52.0) | 46.4 (44.8–48.3) | 0.068 | |
Total bilirubin, μmol/L | 12.0 (9.5–15.0) | 11.0 (9.8–17.3) | 0.692 | 13.0 (10.5–19.5) | 14.0 (11.5–22.5) | 0.581 | |
Fasting glucose, mmol/L | 5.1 (4.9–6.0) | 5.5 (4.8–6.5) | 0.437 | 5.0 (4.7–6.0) | 5.4 (4.8–6.1) | 0.523 | |
Fasting insulin, pmol/L | 92.5 (64.2–160.8) | 124.5 (90.6–155.7)a | 0.062 | 91.8 (61.0–142.8) | 110.6 (84.2–195.3) | 0.268 | |
Glycosylated hemoglobin, % | 5.8 (5.4–6.3)b | 5.9 (5.4–6.4)c | 0.669 | 5.4 (5.1–6.5) | 5.6 (5.3–6.1) | 0.486 | |
Total cholesterol, mmol/L | 5.3±1.0 | 5.6±1.2 | 0.199 | 4.7 (3.9–5.4) | 5.2 (4.2–5.4) | 0.642 | |
Triglyceride, mmol/L | 1.8 (1.3–2.5) | 2.5 (1.6–3.6) | 0.005 | 1.4 (1.0–1.8) | 2.0 (1.2–2.6) | 0.161 | |
HDL-C, mmol/L | 1.0±0.2 | 1.0±0.2 | 0.226 | 1.0±0.2 | 0.9±0.2 | 0.252 | |
LDL-C, mmol/L | 3.2±0.9 | 3.1±1.0 | 0.648 | 2.8±1.0 | 2.8±0.7 | 0.971 | |
Liver histology features | |||||||
Fibrosis stage, n (%) | <0.001 | 0.135 | |||||
F0 | 23 (46.9) | 6 (14.3) | 9 (42.9) | 1 (5.9) | |||
F1 | 21 (42.9) | 20 (47.6) | 8 (38.1) | 12 (70.6) | |||
F2 | 4 (8.2) | 11 (26.2) | 3 (14.3) | 4 (23.5) | |||
F3 | 0 (0.0) | 3 (7.1) | 1 (4.8) | 0 (0.0) | |||
F4 | 1 (2.0) | 2 (4.8) | 0 (0.0) | 0 (0.0) | |||
Steatosis grade, n (%) | 0.162 | 0.001 | |||||
S0 | 3 (6.1) | 2 (4.8) | 2 (9.5) | 0 (0.0) | |||
S1 | 18 (36.7) | 11 (26.2) | 11 (52.4) | 1 (5.9) | |||
S2 | 17 (34.7) | 14 (33.3) | 7 (33.3) | 13 (76.5) | |||
S3 | 11 (22.4) | 15 (35.7) | 1 (4.8) | 3 (17.6) | |||
Ballooning grade, n (%) | <0.001 | <0.001 | |||||
B0 | 11 (22.4) | 0 (0.0) | 6 (28.6) | 0 (0.0) | |||
B1 | 37 (75.5) | 14 (33.3) | 15 (71.4) | 6 (35.3) | |||
B2 | 1 (2.0) | 28 (66.7) | 0 (0.0) | 11 (64.7) | |||
Lobular inflammation grade, n (%) | <0.001 | <0.001 | |||||
L0 | 5 (10.2) | 0 (0.0) | 4 (19.0) | 0 (0.0) | |||
L1 | 41 (83.7) | 14 (33.3) | 16 (76.2) | 3 (17.6) | |||
L2 | 3 (6.1) | 25 (59.5) | 1 (4.8) | 13 (76.5) | |||
L3 | 0 (0.0) | 3 (7.1) | 0 (0.0) | 1 (5.9) | |||
Definitive NASH‡ | 9 (18.4) | 30 (71.4) | <0.001 | 1 (4.8) | 17 (100.0) | <0.001 |
Continuous variables were expressed as means ± SD or medians with IQRs; categorical variables were expressed as number (percentages). a, 40 (95.2%) cases were available; b, 47 (95.9%) cases were available; c, 41 (97.6%) cases were available; ‡, definitive NASH was defined as the presence of steatosis, lobular inflammation and ballooning with NAS score ≥5. NAFLD, nonalcoholic fatty liver disease; MIA, mild hepatic inflammatory activity; SIA, severe inflammation activity; ALT, alanine aminotransferase; AST, aspartate aminotransferase; HDL-C, high-density lipoprotein cholesterol; LDL-C, low-density lipoprotein cholesterol; NASH, nonalcoholic steatohepatitis; SD, standard deviation; IQRs, interquartile ranges; NAS, NAFLD activity score.
After feature selection (as shown in Figure 1), there were 18 radiomics features remaining, and the corresponding coefficients were calculated. The T2-RS value was developed by adding the selected radiomics features, weighted by their coefficients, with good calibration (Hosmer-Lemeshow goodness of fit test, P=0.84). The formula of the T2-RS is shown in the Appendix 1.
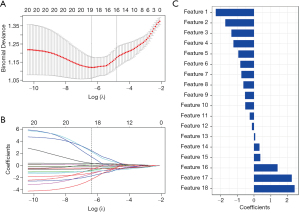
In the training set, the T2-RS was higher in the SIA group than in the MIA group (P<0.001) (Figure 2). Figure 3A shows the ROC curve for the T2-RS in the training set. The T2-RS showed good prediction for SIA with an AUROC of 0.80 [95% confidence interval (CI), 0.71–0.89], sensitivity of 76.19%, specificity of 73.47%, and accuracy of 74.73%, respectively (Table 4). The Youden cut-off value was −0.139.
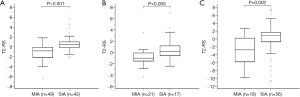
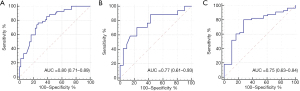
Table 4
Characteristics | Training set (n=91) | Internal validation set (n=38) | External validation set (n=74) |
---|---|---|---|
AUC (95% CI) | 0.80 (0.71–0.89) | 0.77 (0.61–0.93) | 0.75 (0.63–0.84) |
Sensitivity, % (n/N) | 76.19 (32/42) | 70.59 (12/17) | 71.43 (40/56) |
Specificity, % (n/N) | 73.47 (36/49) | 76.19 (16/21) | 72. 22 (13/18) |
Accuracy, % (n/N) | 74.73 (68/91) | 73.68 (28/38) | 71.62 (53/74) |
PPV, % (n/N) | 71.11 (32/45) | 70.59 (12/17) | 88.89 (40/45) |
NPV, % (n/N) | 78.26 (36/46) | 76.19 (16/21) | 44.83 (13/29) |
LR+ | 2.87 | 2.96 | 2.57 |
LR− | 0.32 | 0.38 | 0.40 |
Diagnostic odds ratio | 8.97 | 7.79 | 6.43 |
T2-RS, radiomics signature based on T2WI; NAFLD, nonalcoholic fatty liver disease; AUC, area under the receiver operating characteristic curve; CI, confidence interval; PPV, positive predictive value; NPV, negative predictive value; LR+, positive likelihood ratio; LR−, negative likelihood ratio.
Internal validation of T2-RS
38 individuals from the Wenzhou cohort were assigned to the internal validation set, of whom 71.1% were men. Their mean age was 39.9±10.5 years. There were few differences between the internal validation cohort and the training cohort (as shown in Table 2). Overall, there were 21 subjects with MIA and 17 subjects with SIA. None of the demographics, metabolic factors, and laboratory parameters differed significantly between the two groups (Table 3).
When applying the T2-RS to this internal validation cohort, the T2-RS was higher in the SIA group than in the MIA group (P=0.005) (Figure 2). The AUROC for the internal validation set was 0.77 (95% CI, 0.61–0.93). Using the aforementioned T2-RS cut-off value of −0.139, the sensitivity was 70.59%, the specificity was 76.19%, with an accuracy of 73.68% (Table 4). The ROC curve for the T2-RS in the internal validation cohort is shown in Figure 3B. The result of the Hosmer-Lemeshow test showed a relatively good fit (P=0.63).
External validation of T2-RS
A total of 74 individuals with biopsy-proven NAFLD from the Guangzhou cohort were included in the independent external validation analysis (Table S2). Table 2 shows the characteristics of this external validation cohort. The mean age was 41.9±10.9 years and most of these subjects were men (68.9%). Although serum levels of AST, fasting insulin and HDL-C were significantly different between the external validation and training cohorts, there were few differences between the two groups (Table 2). More subjects in the Guangzhou cohort had a higher degree of hepatic inflammatory activity (MIA/SIA: 18/56). No significant differences in demographics and main laboratory parameters were observed between the MIA and SIA groups in the Guangzhou cohort (Table 1).
As validated in the independent external cohort, the T2-RS was significantly greater in the SIA group than in the MIA group (P<0.01) (Figure 2). The AUROC for the T2-RS was 0.75 (95% CI, 0.63–0.84) with a good fit (Hosmer-Lemeshow test, P=0.25). The accuracy, sensitivity, and specificity was 71.62%, 71.43% and 72.22%, respectively (Table 4). The ROC for the T2-RS in the external validation cohort is shown in Figure 3C.
Evaluation of the effects of hepatic iron content and liver fibrosis on the T2-RS
There were no significant differences in hepatic iron staining grade between the MIA and SIA groups both in the training set (P=0.127) and in the internal validation set (P=0.225). Similarly, the T2-RS did not show any significant difference across the grades of hepatic iron staining (P=0.145 for the training set and P=0.895 for the internal validation set). With regard to liver fibrosis, no significant differences in T2-RS values were found across the histological stage of liver fibrosis in either the Wenzhou cohort (P=0.109) or the Guangzhou cohort (P=0.44).
Discussion
In this study, we have used two independent cohorts of Chinese adult individuals with biopsy-confirmed NAFLD to develop and validate a new set of T2-RS, which can be used to non-invasively assess hepatic inflammation. To our knowledge, this is the first study to use T2-RS to evaluate the hepatic inflammatory activity in people with histologically proven NAFLD.
Non-invasive blood biomarkers, such as serum ALT and cytokeratin-18 levels, have been used to non-invasively assess hepatic inflammatory activity in NAFLD/NASH. However, neither have accepted diagnostic thresholds and both have poor sensitivity to diagnose hepatic inflammation (31-33). Regarding imaging techniques used for assessing hepatic inflammation, the visual examination of imaging modalities has been used to determine hepatic inflammatory activity in the past (34). However, the high inter-observer variability and the unsatisfactory performance largely limit its use in clinical practice (24). França et al. (17) recently proposed a quantitative method of ‘intra-voxel incoherent motion diffusion-weighted MRI’ for the assessment of hepatic inflammation. In such study, the investigators showed that the apparent diffusion coefficient (ADC) and the perfusion fraction were associated with the degree of hepatic inflammation, yielding AUROCs of 0.682 for ADC, and 0.687 for perfusion fraction, respectively. However, these simple quantitative analyses are not good enough for identifying relationships between imaging and liver pathology. A multiparametric MRI protocol composed of T1 maps, T2* maps and proton magnetic resonance spectroscopy has been developed, aiming at using multi-sequence imaging to characterize liver tissue (15,19). The hepatic inflammation and fibrosis scores derived from this imaging technique using specialized, patented software (Perspectum Diagnostics, Oxford, UK, LiverMultiScanTM) provided high diagnostic accuracy for the assessment of liver pathology. However, it should be noted that the specialized software of LiverMultiScanTM limits its widespread use, because of necessary payment for this software. Recently, in a small study of 137 Chinese patients with chronic hepatitis B or other chronic liver diseases, the authors found that MRI-based radiomics models yielded good diagnostic performance for identifying hepatic inflammation (24). However, as this study was limited to a single center without any independent external validation, the robustness and generalizability of the findings remain unconfirmed. In contrast, in our study, the T2-RS, was developed and subsequently validated in two different and independent cohorts of subjects with NAFLD. Developing our radiomics signature involved various MRI scanners and its scan sequence parameters showed good diagnostic performance. The robustness of the radiomics signature was tested by re-analyzing the T2WI images by resampling a nominal voxel size and normalizing the gray levels number before extraction of radiomics features (35,36). The radiomics features, which were derived from T2WI and used to construct the T2-RS, have been reported to have good-to-excellent intra-/inter-observer reproducibility (37,38) and robustness to TR/TE changes in some degree (39). Furthermore, we observed comparable diagnostic performances in the two independent cohorts of individuals with different degrees of hepatic inflammation, thereby suggesting that the T2-RS has good reproducibility. In addition, it is important to underline that axial T2WI is a routine sequence obtained during upper abdomen MRI scans; T2WI does not require additional scan time, and it does not require extra hardware or post imaging processing software.
In our study, there were different levels of liver fibrosis in the two groups of patients with MIA or SIA. Hepatic T2 relaxation times have been reported to be associated with liver fibrosis (40,41). However, Heye et al. (42) did not find any significant difference in T2 relaxation times between patients with cirrhosis and control subjects. In contrast, they found that T1 relaxation times were significantly higher in patients with cirrhosis. This finding implies that liver fibrosis may affect the T1 signal intensity significantly more than the T2 signal intensity, and this result was also supported by data from analysis of T1WI and T2WI using a machine learning approach (43). It is possible that differential effects of hepatic inflammation and fibrosis may lead to these opposite results. In our study, we compared the TS-RS across histological stages of liver fibrosis, but did not find any significant difference in the TS-RS according to the severity of liver fibrosis. Thus, we reason that the difference in fibrosis stages we observed in our study had little effect on the ability of T2-RS to predict hepatic inflammation. That said, the effect of liver fibrosis on the ability of T2-RS to predict hepatic inflammation still needs further investigation in rigorously controlled studies.
The T2-RS developed in this study was mainly based on T2WI. The presence of hepatic iron can shorten T2 relaxation times (44). We further evaluated the effect of hepatic iron content on the T2-RS. However, we did not find any significant difference in T2-RS across different hepatic iron staining grades, both in the training set and in the internal validation set. This result suggests that the T2-RS is not markedly affected by hepatic iron content. The T2-RS was constructed from texture features, which mainly reflect the spatial distribution of different gray pixels rather than simple measurement of T2 relaxation time, and this might explain why T2-RS is not markedly affected by hepatic iron content.
For the study of liver pathology, various kinds of ROIs have been used in previous studies (45-47) to assess localized liver regions, the right liver lobe or the whole liver. There is no agreement as to which kind of ROI is preferable. Rezvani Habibabadi et al. (48) used MRI elastography to quantify liver stiffness and found that the whole liver segment was better than localized regions. In contrast, another study showed by comparing different ROIs, that there was little effect of different ROIs (49). In our study, radiomics features were extracted only from localized regions in the right liver lobe. Although a localized ROI does not represent the overall changes in liver parenchyma, a radiomics analysis of chronic liver disease by Song et al. (24) showed that the radiomics model, based on localized ROIs, yielded comparable diagnostic performance to a model based on the whole liver, suggesting that the quantitative analysis of part of the liver may be sufficient in NAFLD.
There are some limitations to our study. First, with 203 participants our sample size was relatively small. That said, however, it is very uncommon amongst individuals with biopsy-proven NAFLD to also perform simultaneous liver MRI scans in clinical practice. To our knowledge, this study is the first and largest study to date to assess the performance of T2-RS for diagnosing hepatic inflammation in people with NAFLD. The potential differential impact of sex on T2-RS and the predictive performance of T2-RS in men and women still needs further study. The possible influence of sampling errors due to the usage of a localized ROI also needs to be considered, although we think it is feasible to use a localized ROI as discussed above. Moreover, only the T2WI sequence was used in this study. Whether other MRI sequences or combinations are better, needs further study. Finally, the lack of external validation cohorts of other ethnicities is another possible limitation of our study.
In conclusion, the results of this study suggest that our newly developed T2-RS is a promising imaging-related technique for non-invasive assessment of the severity of hepatic inflammation in patients with biopsy-confirmed NAFLD.
Acknowledgments
Funding: This work was supported by grants from the National Natural Science Foundation of China (82070588), High Level Creative Talents from Department of Public Health in Zhejiang Province (S2032102600032), Project of New Century 551 Talent Nurturing in Wenzhou. GT is supported in part by grants from the University School of Medicine of Verona, Verona, Italy. CDB is supported in part by the Southampton NIHR Biomedical Research Centre (IS-BRC-20004), UK.
Footnote
Reporting Checklist: The authors have completed the TRIPOD reporting checklist. Available at https://hbsn.amegroups.com/article/view/10.21037/hbsn-21-23/rc
Data Sharing Statement: Available at https://hbsn.amegroups.com/article/view/10.21037/hbsn-21-23/dss
Conflicts of Interest: All authors have completed the ICMJE uniform disclosure form (available at https://hbsn.amegroups.com/article/view/10.21037/hbsn-21-23/coif). MHZ serves as an unpaid editorial board member of Hepatobiliary Surgery and Nutrition. The other authors have no conflicts of interest to declare.
Ethical Statement: The authors are accountable for all aspects of the work in ensuring that questions related to the accuracy or integrity of any part of the work are appropriately investigated and resolved. The study was conducted in accordance with the Declaration of Helsinki (as revised in 2013). The study was approved by the local ethics board of the First Affiliated Hospital of Wenzhou Medical University and Guangdong Provincial Hospital of Chinese Medicine (No. 2016-246) and informed consent was taken from all individual participants.
Open Access Statement: This is an Open Access article distributed in accordance with the Creative Commons Attribution-NonCommercial-NoDerivs 4.0 International License (CC BY-NC-ND 4.0), which permits the non-commercial replication and distribution of the article with the strict proviso that no changes or edits are made and the original work is properly cited (including links to both the formal publication through the relevant DOI and the license). See: https://creativecommons.org/licenses/by-nc-nd/4.0/.
References
- Younossi Z, Anstee QM, Marietti M, et al. Global burden of NAFLD and NASH: trends, predictions, risk factors and prevention. Nat Rev Gastroenterol Hepatol 2018;15:11-20. [Crossref] [PubMed]
- Zheng KI, Fan JG, Shi JP, et al. From NAFLD to MAFLD: a "redefining" moment for fatty liver disease. Chin Med J (Engl) 2020;133:2271-3. [Crossref] [PubMed]
- Eslam M, Newsome PN, Sarin SK, et al. A new definition for metabolic dysfunction-associated fatty liver disease: an international expert consensus statement. J Hepatol 2020;73:202-9. [Crossref] [PubMed]
- Lee UE, Friedman SL. Mechanisms of hepatic fibrogenesis. Best Pract Res Clin Gastroenterol 2011;25:195-206. [Crossref] [PubMed]
- Hernandez-Gea V, Friedman SL. Pathogenesis of liver fibrosis. Annu Rev Pathol 2011;6:425-56. [Crossref] [PubMed]
- Kleiner DE, Brunt EM, Van Natta M, et al. Design and validation of a histological scoring system for nonalcoholic fatty liver disease. Hepatology 2005;41:1313-21. [Crossref] [PubMed]
- Tobkes AI, Nord HJ. Liver biopsy: review of methodology and complications. Dig Dis 1995;13:267-74. [Crossref] [PubMed]
- Mikolasevic I, Orlic L, Franjic N, et al. Transient elastography (FibroScan®) with controlled attenuation parameter in the assessment of liver steatosis and fibrosis in patients with nonalcoholic fatty liver disease - where do we stand? World J Gastroenterol 2016;22:7236-51. [Crossref] [PubMed]
- Furlan A, Tublin ME, Yu L, et al. Comparison of 2D shear wave elastography, transient elastography, and MR elastography for the diagnosis of fibrosis in patients with nonalcoholic fatty liver disease. AJR Am J Roentgenol 2020;214:W20-6. [Crossref] [PubMed]
- Idilman IS, Li J, Yin M, et al. MR elastography of liver: current status and future perspectives. Abdom Radiol (NY) 2020;45:3444-62. [Crossref] [PubMed]
- Rios RS, Zheng KI, Targher G, et al. Non-invasive fibrosis assessment in non-alcoholic fatty liver disease. Chin Med J (Engl) 2020;133:2743-5. [Crossref] [PubMed]
- Zhou YJ, Zheng KI, Targher G, et al. Non-invasive diagnosis of non-alcoholic steatohepatitis and liver fibrosis. Lancet Gastroenterol Hepatol 2021;6:9-10. [Crossref] [PubMed]
- Miele L, Zocco MA, Pizzolante F, et al. Use of imaging techniques for non-invasive assessment in the diagnosis and staging of non-alcoholic fatty liver disease. Metabolism 2020; Epub ahead of print. [Crossref] [PubMed]
- Pavlides M, Banerjee R, Sellwood J, et al. Multiparametric magnetic resonance imaging predicts clinical outcomes in patients with chronic liver disease. J Hepatol 2016;64:308-15. [Crossref] [PubMed]
- Banerjee R, Pavlides M, Tunnicliffe EM, et al. Multiparametric magnetic resonance for the non-invasive diagnosis of liver disease. J Hepatol 2014;60:69-77. [Crossref] [PubMed]
- Zheng KI, Liu WY, Pan XY, et al. Combined and sequential non-invasive approach to diagnosing non-alcoholic steatohepatitis in patients with non-alcoholic fatty liver disease and persistently normal alanine aminotransferase levels. BMJ Open Diabetes Res Care 2020;8:e001174. [Crossref] [PubMed]
- França M, Martí-Bonmatí L, Alberich-Bayarri Á, et al. Evaluation of fibrosis and inflammation in diffuse liver diseases using intravoxel incoherent motion diffusion-weighted MR imaging. Abdom Radiol (NY) 2017;42:468-77. [Crossref] [PubMed]
- Sandrasegaran K, Territo P, Elkady RM, et al. Does intravoxel incoherent motion reliably stage hepatic fibrosis, steatosis, and inflammation? Abdom Radiol (NY) 2018;43:600-6. [Crossref] [PubMed]
- Pavlides M, Banerjee R, Tunnicliffe EM, et al. Multiparametric magnetic resonance imaging for the assessment of non-alcoholic fatty liver disease severity. Liver Int 2017;37:1065-73. [Crossref] [PubMed]
- Lambin P, Rios-Velazquez E, Leijenaar R, et al. Radiomics: extracting more information from medical images using advanced feature analysis. Eur J Cancer 2012;48:441-6. [Crossref] [PubMed]
- Hu W, Yang H, Xu H, et al. Radiomics based on artificial intelligence in liver diseases: where we are? Gastroenterol Rep (Oxf) 2020;8:90-7. [Crossref] [PubMed]
- Daginawala N, Li B, Buch K, et al. Using texture analyses of contrast enhanced CT to assess hepatic fibrosis. Eur J Radiol 2016;85:511-7. [Crossref] [PubMed]
- Wang K, Lu X, Zhou H, et al. Deep learning Radiomics of shear wave elastography significantly improved diagnostic performance for assessing liver fibrosis in chronic hepatitis B: a prospective multicentre study. Gut 2019;68:729-41. [Crossref] [PubMed]
- Song J, Yu X, Song W, et al. MRI-based radiomics models developed with features of the whole liver and right liver lobe: assessment of hepatic inflammatory activity in chronic hepatic disease. J Magn Reson Imaging 2020;52:1668-78. [Crossref] [PubMed]
- Guimaraes AR, Siqueira L, Uppal R, et al. T2 relaxation time is related to liver fibrosis severity. Quant Imaging Med Surg 2016;6:103-14. [Crossref] [PubMed]
- Kita K, Kita M, Sato M, et al. MR imaging of liver cirrhosis. Acta Radiol 1996;37:198-203. [Crossref] [PubMed]
- Goudsmit BFJ, Putter H, Tushuizen ME, et al. Refitting the model for end-stage liver disease for the eurotransplant region. Hepatology 2021;74:351-63. [Crossref] [PubMed]
- Brunt EM, Janney CG, Di Bisceglie AM, et al. Nonalcoholic steatohepatitis: a proposal for grading and staging the histological lesions. Am J Gastroenterol 1999;94:2467-74. [Crossref] [PubMed]
- Bedossa P, Poitou C, Veyrie N, et al. Histopathological algorithm and scoring system for evaluation of liver lesions in morbidly obese patients. Hepatology 2012;56:1751-9. [Crossref] [PubMed]
- Searle JW, Leggett BA, Crawford DHG, et al. Iron storage disease. In: MacSween RNM, Burt AD, Portmann BC, et al. editors. Pathology of the Liver. Edinburgh: Churchill Livingstone, 2002:260.
- Verma S, Jensen D, Hart J, et al. Predictive value of ALT levels for non-alcoholic steatohepatitis (NASH) and advanced fibrosis in non-alcoholic fatty liver disease (NAFLD). Liver Int 2013;33:1398-405. [Crossref] [PubMed]
- Kwok R, Tse YK, Wong GL, et al. Systematic review with meta-analysis: non-invasive assessment of non-alcoholic fatty liver disease--the role of transient elastography and plasma cytokeratin-18 fragments. Aliment Pharmacol Ther 2014;39:254-69. [Crossref] [PubMed]
- Balakrishnan M, Loomba R. The role of noninvasive tests for differentiating NASH from NAFL and diagnosing advanced fibrosis among patients with NAFLD. J Clin Gastroenterol 2020;54:107-13. [Crossref] [PubMed]
- Shu J, Zhao JN, Han FG, et al. Chronic hepatitis B: correlation of abnormal features on T2-weighted imaging and dynamic contrast-enhanced imaging with hepatic histopathology. Radiol Med 2017;122:807-13. [Crossref] [PubMed]
- Shafiq-Ul-Hassan M, Zhang GG, Latifi K, et al. Intrinsic dependencies of CT radiomic features on voxel size and number of gray levels. Med Phys 2017;44:1050-62. [Crossref] [PubMed]
- Chirra P, Leo P, Yim M, et al. Multisite evaluation of radiomic feature reproducibility and discriminability for identifying peripheral zone prostate tumors on MRI. J Med Imaging (Bellingham) 2019;6:024502. [Crossref] [PubMed]
- Baeßler B, Weiss K, Pinto Dos Santos D. Robustness and reproducibility of radiomics in magnetic resonance imaging: a phantom study. Invest Radiol 2019;54:221-8. [Crossref] [PubMed]
- Rai R, Holloway LC, Brink C, et al. Multicenter evaluation of MRI-based radiomic features: a phantom study. Med Phys 2020;47:3054-63. [Crossref] [PubMed]
- Bianchini L, Santinha J, Loução N, et al. A multicenter study on radiomic features from T2-weighted images of a customized MR pelvic phantom setting the basis for robust radiomic models in clinics. Magn Reson Med 2021;85:1713-26. [Crossref] [PubMed]
- Luetkens JA, Klein S, Träber F, et al. Quantification of liver fibrosis at T1 and T2 mapping with extracellular volume fraction MRI: preclinical results. Radiology 2018;288:748-54. [Crossref] [PubMed]
- Bashyam A, Frangieh CJ, Raigani S, et al. A portable single-sided magnetic-resonance sensor for the grading of liver steatosis and fibrosis. Nat Biomed Eng 2021;5:240-51. [Crossref] [PubMed]
- Heye T, Yang SR, Bock M, et al. MR relaxometry of the liver: significant elevation of T1 relaxation time in patients with liver cirrhosis. Eur Radiol 2012;22:1224-32. [Crossref] [PubMed]
- Schawkat K, Ciritsis A, von Ulmenstein S, et al. Diagnostic accuracy of texture analysis and machine learning for quantification of liver fibrosis in MRI: correlation with MR elastography and histopathology. Eur Radiol 2020;30:4675-85. [Crossref] [PubMed]
- Cheng HL, Stikov N, Ghugre NR, et al. Practical medical applications of quantitative MR relaxometry. J Magn Reson Imaging 2012;36:805-24. [Crossref] [PubMed]
- Naganawa S, Enooku K, Tateishi R, et al. Imaging prediction of nonalcoholic steatohepatitis using computed tomography texture analysis. Eur Radiol 2018;28:3050-8. [Crossref] [PubMed]
- Zheng Y, Xu YS, Liu Z, et al. Whole-liver apparent diffusion coefficient histogram analysis for the diagnosis and staging of liver fibrosis. J Magn Reson Imaging 2020;51:1745-54. [Crossref] [PubMed]
- Murphy P, Hooker J, Ang B, et al. Associations between histologic features of nonalcoholic fatty liver disease (NAFLD) and quantitative diffusion-weighted MRI measurements in adults. J Magn Reson Imaging 2015;41:1629-38. [Crossref] [PubMed]
- Rezvani Habibabadi R, Khoshpouri P, Ghadimi M, et al. Comparison between ROI-based and volumetric measurements in quantifying heterogeneity of liver stiffness using MR elastography. Eur Radiol 2020;30:1609-15. [Crossref] [PubMed]
- Cogneau A, Cho H, Lucidarme O, et al. Do regions of interest location and type influence liver stiffness measurement using magnetic resonance elastography? Diagn Interv Imaging 2019;100:363-70. [Crossref] [PubMed]