Epigenome-wide development and validation of a prognostic methylation score in intrahepatic cholangiocarcinoma based on machine learning strategies
Introduction
Intrahepatic cholangiocarcinoma (ICC), the second most common primary liver cancer after hepatocellular carcinoma, is an aggressive malignancy with an increasing incidence worldwide (1). At present, liver resection is the main treatment option with curative intent. However, the long-term outcome of ICC patients after surgical resection is dismal due to high incidence of recurrence. Even after resection, the 5-year overall survival (OS) rate is 25.5–41.0%, and the cumulative recurrence rate at 5 years can go as high as 70.5–73.4% (2,3). Although no high-level evidence from randomized clinical trials exists, studies from various centers have found that select ICC patients with high-risk features can benefit from postoperative adjuvant therapies, which highlights the necessity of risk stratification for avoiding a potential undertreatment or overtreatment of patients (4). Currently, the American Joint Committee on Cancer (AJCC) tumor-node-metastasis (TNM) staging system is the most frequently used staging system for stratifying patients after surgery, but it only shows a limited role in predicting long-term survival. Therefore, additional prognostic markers are needed to identify patients at high risk of recurrence or death after receiving hepatectomy.
In terms of prognostic biomarkers of cancer, DNA methylation has drawn increasing attention in recent years. The prognostic role of methylation markers or signatures has been confirmed in various types of cancer (5,6) but remains to be elucidated for ICC. Abnormal DNA methylation at 5’methylcytosine (5-mC) has been reported to be involved in the occurrence and progression of ICC (7-15). Previous studies have focused on one or several common tumor suppressor genes (TSGs) by using methylation-specific PCR or a small fraction of the genome by array-based approaches, mainly in the CpG island (CGI) or promoter regions. For example, the promoter regions or CGI of p15, GSTP, and CDH1 genes were reported to be frequently methylated in ICC compared with the benign bile duct epithelium and silence the expression of those genes, indicating a role of DNA methylation in cholangiocarcinogenesis and the potential value of differential diagnosis in clinical settings (7-10). In more recent studies, investigators have employed high-throughput methylation arrays and revealed an enrichment of methylated genes in cancer-related pathways (for example, the Wnt signaling pathway) or a stem cell-like phenotype, and methylation-based molecular subtypes of cholangiocarcinoma (11-15). Although these studies have often reported ICC and extrahepatic cholangiocarcinoma (ECC) together and consisted of ICC cohorts with a small sample size, their findings have further affirmed the deep involvement of DNA methylation in the carcinogenesis of ICC, and implied the potential of DNA methylation as a predictor of prognosis at the same time. To explore the prognostic value of DNA methylation in ICC, we performed this multicenter study using whole-genome bisulfite sequencing (WGBS), which is currently the state-of-the-art technology for methylation detection. Given the fact that ICC differs from ECC at the anatomical, clinicopathological, and molecular levels, we only included ICC patients in the current study. We present this article in accordance with the TRIPOD reporting checklist (available at https://hbsn.amegroups.com/article/view/10.21037/hbsn-21-424/rc).
Methods
Study design
The current study comprised a multicenter and retrospective cohort of ICC patients. From May 2010 to July 2019, a total of 164 patients in the West China Hospital of Sichuan University (WCHSU cohort), 117 patients in the Zhongshan Hospital of Fudan University (ZSHFU cohort), and 53 patients in the Tianjin Medical University Cancer Institute and Hospital (TMUCIH cohort) who received curative-intent hepatectomy were included in our study. All patients were histologically diagnosed with ICC for the first time, and no recurrent ICC patients were included. Patients with recurrent ICC, or missing follow-up, or OS of less than 1 month were excluded. As demonstrated in Figure 1, the WCHSU cohort was used as the discovery cohort to construct a prognostic model, and the ZSHFU and TMUCIH cohorts were combined into one external validation cohort in view of the low statistical power of a small sample size. The study was approved by the Ethical Committee of West China Hospital of Sichuan University (No. 2019-833), Zhongshan Hospital of Fudan University (No. B2017-060R), and Tianjin Medical University Cancer Institute and Hospital (No. bc2020012), and individual consent for this retrospective analysis was waived. The study was conducted in accordance with the Declaration of Helsinki (as revised in 2013).
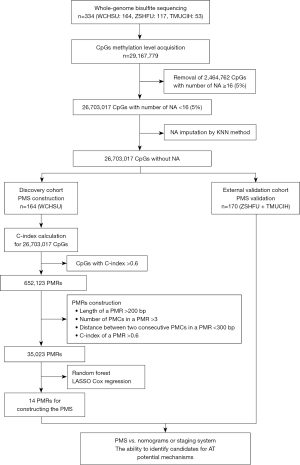
WGBS experiments and data analysis
ICC samples were acquired from the biobank of each center, and samples were immediately snap frozen in liquid nitrogen after surgery and stored at −80 ℃ or liquid nitrogen. Genomic DNA isolation, WGBS library generation, sequencing, quality control and WGBS data processing are described in the Appendix 1.
Prognostically methylated regions (PMRs) definition
Previous methylation analysis based on the Infinium HumanMethylation450 BeadChip (450k array) has often used methylation levels of individual CpGs corresponding to probes in a 450k array as candidate features, and reported differentially methylated CpG sites (DMCs) (14,15). However, investigators have focused on differentially methylated regions (DMRs) instead of DMCs in recent studies based on WGBS (16,17), as there were enough DMCs to be united into DMRs. Similarly, previous studies based on a 450k array constructed prognostic signatures using individual prognostic CpG site (5,6). Owing to the employment of WGBS in this study, we propose the concept of PMRs, which is formed by connecting prognostically methylated CpG (PMCs) sites. PMCs are defined as CpG sites with the concordance index (C-index) for OS more than 0.6. The C-index of AJCC TNM staging system is 0.61, and we use 0.6 as the cutoff to define PMCs. Similar to the construction of DMRs, PMRs are required to meet the following criteria: the minimum length of a PMR is more than 200 bps; a PMR consists of at least 3 PMCs; the maximum distance between consecutive PMCs is less than 300 bps; the C-index of a PMR is more than 0.6 (16-18) (Figure 1). The C-index is calculated using the R package “rms”, and the PMRs are constructed using the “merge” command of “bedtools”.
Prognostic methylation score (PMS) construction using machine learning strategies
The random forest is a widely used machine learning algorithm for classification, regression, and other tasks and an efficient way to select significant variables from high-dimensional data. The R package “randomForestSRC” enables investigators to apply this algorithm to survival data (19). As indicated by Ishwaran et al. (19), we used the grid research method to determine the optimal combination of mtry and node based on out-of-bag (OOB) error, which was used for the next random forest model construction and variable hunting process (Figure S1A-S1C). PMRs were filtered by the relative variable importance (VIMP), which is a measurement for the variation of predicted error rate by the random forest model when a PMR was randomly added into the model. Standard deviation (SD) was used as a method for dimension reduction, considering that a good biomarker should show sufficient variation among individuals. After dimension reduction using VIMP, SD, and C-index, candidate PMRs were entered into the least absolute shrinkage and selector operation (LASSO) Cox algorithm to select appropriate variables for building a prognostic model in the training cohort (Figure S1D-S1F). When performing LASSO Cox, cross-validation was run 100 times, and the best lambda with the minimum mean cross-validation error was selected. The LASSO Cox was performed using the R package “glmnet”.
Nomogram construction
To reproduce the nomogram reported by Wang et al. (20) for the Eastern Hepatobiliary Surgery Hospital (EHBSH) nomogram, we used the same coefficients [coefficient = ln(HR)] for the variables that were included. The EHBSH nomogram score was calculated as follow: ln(1.001) × CA19-9 + ln(1.011) × CEA + ln(1.605) × vascular invasion + ln(1.592) × direct invasion and local extrahepatic metastasis + ln(2.057) × lymph node metastasis + ln(1.078) × tumor diameter + ln(1.582)/ln(6.096) × tumor number. Then, the score was used for the next-step comparison.
The core of the nomogram reported by Hyder et al. (21) for the Johns Hopkins University School of Medicine (JHUSM) nomogram lied in transformation of continuous variables (age and tumor size) by restricted cubic splines. As the coefficients for each variable after transformation were not given by the authors, we were not able to regenerate the JHUSM nomogram as the EHBSH nomogram. As an alternative, the same restricted cubic splines transformation and clinical parameters was applied in our own data to validate the JHUSM nomogram. The C-index (0.69) of JHUSM nomogram by our data was similar as the original one (0.70/0.71) with good calibration (P=0.691, Grønnesby and Borgan test), and Kaplan-Meier survival curves demonstrated the same trend (smallest gap between quartile 1 and quartile 2) (Figure S2).
Statistics
All statistics were performed by the RStudio 1.1.463, SPSS 25.0, and GraphPad Prism 8 software. Major hepatectomy was defined as a removal of ≥3 segments, and minor hepatectomy was defined as the resection of <3 segments. Univariate survival analyses for clinicopathologic characteristics and PMS were performed using the univariate Cox method, and factors with P<0.05 was entered into the Cox proportional hazard model to identify independent risk factors. The R package “rms” was used for C-index calculation. A time-dependent receiver operating characteristic curve (ROC) was generated, and the area under the curve (AUC) was measured by the R package “timeROC”. The performance of our model, nomograms, and the AJCC TNM staging system (8th edition) was compared and demonstrated by the C-index, AUC, and Kaplan-Meier survival curves. Survival risk stratification was carried out based on the PMS, nomogram scores, MEGNA scores, or TNM stages. Survival difference was compared between patients receiving adjuvant therapy (AT) and those receiving no AT in different quartiles of PMS or nomogram score, MEGNA score groups, or TNM stages. When survival difference (log-rank P<0.05) was detected in a risk stratification group, it was concluded that AT could improve survival in the risk stratification group and patients in the risk stratification group could benefit from AT like before (22). For all tests, a two-tailed P<0.05 was considered statistically significant.
Results
Study population
There were 164 and 170 patients in the discovery and validation cohorts, respectively. The median follow-up in the discovery cohort was 28.5 months, and 106 (64.3%) patients died in the follow-up period. The median follow-up in the validation cohort was 19.0 months, and 62 (36.5%) patients died in the validation cohort. The early mortality (90-day) rate in the whole cohort after surgery was 0.9% (3/334). As indicated in Table S1, some baseline characteristics were not balanced between the two cohorts, including general condition [age, ascites, total bilirubin (TB), aspartate aminotransferase (AST), and prothrombin time (PT)], tumor characteristics (macrovascular vascular invasion, microvascular invasion, liver capsule invasion, lymph node metastasis and TNM stage), and treatment strategy (major/minor hepatectomy, R0 margin and AT) related factors, which are interpreted in the Discussion section.
PMCs and PMRs
Among 26,703,017 CpG sites, a total of 652,123 CpG sites with C-index more than 0.6 (P<0.05) were defined as PMCs, and 35,023 PMRs were identified from these PMCs. A major challenge in biomarker hunting is the efficacy in the validation cohort. In the original discovery and validation cohorts, 47.0% PMCs detected in the discovery cohort remained PMCs in the validation cohort, and 78.8% PMRs in the discovery cohort were confirmed in the validation cohort. That is, PMRs defined in the discovery cohort had a larger possibility to be validated as PMRs in the validation cohort, indicating that PMRs were better prognostic biomarkers than PMCs. To further confirm our inference, we randomly split the whole cohort into discovery and validation cohorts nine times using the “createDataPartition” function in the R package “caret” and calculated the proportions of PMCs and PMRs that were detected in the discovery cohort and gained confirmation in the validation cohort. As indicated in Figure 2A, PMRs showed higher proportions than PMCs each time, implying the stability of our findings.
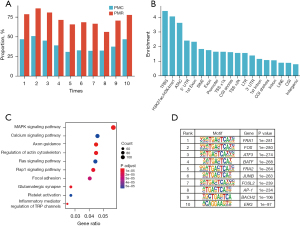
To characterize the potential functions of PMRs, the functional genome enrichment analysis of PMRs was performed. The results indicated that the PMRs tended to be enriched at ICC-specific transcription factor binding sites (TFBS), ICC-specific putative enhancer regions marked by H3K27ac/H3K4me1, and ICC-specific assay for transposase-accessible chromatin (ATAC)-seq peaks (Figure 2B). In contrast, the PMRs were less likely to be enriched at long interspersed nuclear elements (LINE), CGI, and intergenic regions (Figure 2B). Notably, CGI has been most prominent in previous studies. Kyoto Encyclopedia of Genes and Genomes (KEGG) pathway analysis using genes whose promoter regions and bodies overlapped with the PMRs demonstrated that several cancer-related pathways were significantly enriched, for example, the MAPK signaling pathway and calcium signaling pathway (Figure 2C). Motif enrichment analysis demonstrated that several important transcription factors (TFs) that were reported to be involved in cholangiocarcinoma occurrence were enriched for PMRs (Figure 2D) (23-25). These findings indicated that the PMRs possessed potential biological functions and might be suitable for being used as prognostic markers.
PMS construction
The data preprocess is shown in Figure 1. As indicated in Figure S1D, 362 regions met the defined criteria as follows: C-index >0.65, VIMP >0.1, and SD >0.1. Finally, 14 regions were selected by the LASSO Cox algorithm to construct the PMS (Figure S1E,S1F). PMS is a combination index of each candidate PMR methylation level and corresponding coefficient. Information on the 14 PMRs is shown in Table 1, and the detailed formula of the PMS is listed in the Appendix 1. As demonstrated in Figure S3, the individual C-index of 14 PMRs was less than that of the PMS, and the C-indices of 13 regions were still larger than 0.6 in the validation cohort.
Table 1
Region | Width | Coefficient | Annotated features | Annotated genes | Gene type |
---|---|---|---|---|---|
chr1:171,854,763-171,854,992 | 229 | −0.353 | Intron/LINE | DNM3 | Protein coding gene |
chr2:21,710,188-21,710,438 | 250 | −0.202 | Promote/1st exon/3' UTRa | LINC01822 | LncRNA gene |
chr2:62,464,507-62,465,170 | 663 | −0.232 | 1st intron/LINE/LTR | TMEM17/LOC105374764 | Protein coding/LncRNA gene |
chr3:179,767,886-179,768,188 | 302 | −0.593 | Intron | USP13 | Protein coding gene |
chr6:14,396,913-14,397,254 | 341 | −0.742 | 3' UTR/exona | LOC105374943 | LncRNA gene |
chr7:149,987,544-149,987,810 | 266 | −0.187 | 1st intron | ACTR3C | Protein coding gene |
chr9:22,005,150-22,006,798 | 1,648 | −0.464 | 3' UTR/exon/intron/CGI/CGI shelves/H3K27ac/H3K4me1a | CDKN2B/CDKN2B-AS1 | Protein coding/LncRNA gene |
chr10:239,222-239,510 | 288 | −0.899 | 5' UTR/exon/introna | ZMYND11 | Protein coding gene |
chr11:57,165,910-57,166,366 | 456 | −0.387 | Intron/LTR | LOC105369309 | LncRNA gene |
chr12:64,671,264-64,671,938 | 674 | −0.626 | ATAC/H3K27ac/H3K4me1/intron | RASSF3 | Protein coding gene |
chr12:103,319,240-103,319,760 | 520 | −0.477 | 1st intron | C12orf42 | Protein coding gene |
chr15:85,545,689-85,545,931 | 242 | −0.363 | Intron | AKAP13 | Protein coding gene |
chr15:97,383,669-97,383,930 | 261 | −0.358 | 1st intron | LINC02253 | LncRNA gene |
chr18:26,881,728-26,881,977 | 249 | −0.261 | Intron | AQP4-AS1 | LncRNA gene |
a, there are multiple transcripts for one gene, and one PMR can be annotated by different features of different transcripts. PMRs, prognostically methylated regions; PMS, prognostic methylation score; LINE, long interspersed nuclear elements; DNM3, dynamin 3; UTR, untranslated region; LINC01822, long intergenic non-protein coding RNA 1822; LTR, long terminal repeat; TMEM17, transmembrane protein 17; LncRNA, long non-coding RNA; USP13, ubiquitin specific peptidase 13; ACTR3C, actin related protein 3C; CGI, CpG island; CDKN2B, cyclin dependent kinase inhibitor 2B; CDKN2B-AS1, CDKN2B antisense RNA 1; ZMYND11, zinc finger MYDN-type containing 11; ATAC, assay for transposase-accessible chromatin; RASSF3, Ras association domain family member 3; C12orf42, chromosome 12 open reading frame 42; AKAP13, A-kinase anchoring protein 13; LINC02253, long intergenic non-protein coding RNA 2253; AQP4-AS1, aquaporin 4 antisense RNA 1.
PMS validation
To substantiate the stability of PMS, we first performed a PMS investigation in the discovery cohort (WCHSU cohort). The C-index for OS was 0.79 (95% CI: 0.75–0.84). As shown in Figure 3A, the 1-, 2-, and 3-year AUCs for OS were 0.86 (95% CI: 0.79–0.92), 0.86 (95% CI: 0.80–0.92), and 0.89 (95% CI: 0.83–0.94), respectively. The optimal cutoff of the PMS was −4.32, which was determined by the “surv_cutpoint” function in the R package “survminer”. The PMS successfully categorized 90 patients (54.9%) into the PMS-low group and 74 patients (45.1%) into the PMS-high group. The OS of the PMS-low group (median OS: 61.8±12.4 months; 1-, 3-, and 5-year survival rates: 94.4%, 77.3%, and 51.9%) was significantly better than the PMS-high group (median OS: 11.9±1.7 months; 1-, 3-, and 5-year survival rates: 50.0%, 11.4%, and 0%) (log-rank P<0.001; HR =5.63; 95% CI: 3.64–8.70) (Figure 3B).
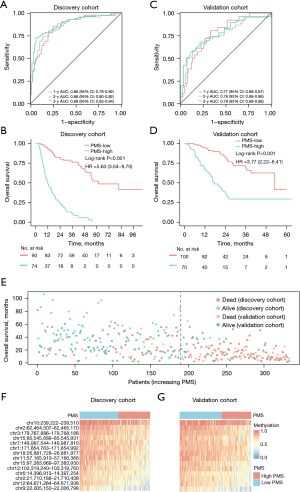
In the external validation cohort (ZSHFU and TMUCIH cohorts), the PMS was calculated by the same PMRs and coefficients as in the discovery cohort, and the C-index for OS was 0.74 (95% CI: 0.68–0.80). As shown in Figure 3C, the 1-, 2-, and 3-year AUCs were 0.77 (95% CI: 0.68–0.87), 0.76 (95% CI: 0.66–0.86), and 0.78 (95% CI: 0.68–0.88), respectively. Similarly, the PMS successfully classified 100 (58.8%) and 70 (41.1%) patients into PMS-low and PMS-high groups by the same cutoff. The OS of the PMS-low group (median OS: 53.0±11.6 months; 1-, 3-, and 5-year survival rates: 94.5%, 71.7%, and 41.6%, respectively) was also significantly better than the PMS-high group (median OS: 17.8±2.3 months; 1-, 3-, and 5-year survival rates: 67.1%, 29.4%, and 29.4%, respectively) (log-rank P<0.001; HR =3.77; 95% CI: 2.22–6.41) (Figure 3D).
As indicated in Figure 3E, with the increase of PMS, both the discovery and validation cohorts displayed a similar trend of mounting patients who died a short time after surgery. The methylation level distributions of 14 PMRs in the discovery and validation cohorts also demonstrated similar patterns (Figure 3F,3G). Collectively, these findings indicate high accuracy of the PMS in the discovery cohort and of satisfying validation effectiveness in the validation cohort.
Performance of PMS
The AJCC TNM staging system and nomograms are often used to predict patient survival, and there are two highly cited nomograms (JHUSM and EHBSH nomograms) and one prognostic score (MEGNA) for ICC published in leading academic journals to date (20,21,26).
First, the PMS was an independent predictor of OS in multivariate analysis with clinical parameters included (P<0.001; HR =8.12; 95% CI: 5.48–12.04) (Table S2), and other independent factors included ascites (P<0.001; HR =2.68; 95% CI: 1.59–4.51), tumor number (P=0.022; HR =1.54; 95% CI: 1.06–2.24), tumor size (P<0.001; HR =1.12; 95% CI: 1.05–1.19), and lymph node metastasis (P<0.001; HR =1.90; 95% CI: 1.33–2.72). The pairwise comparisons demonstrated that the C-index of the PMS (0.78; 95% CI: 0.74–0.81) was significantly higher than that of the JHUSM nomogram (0.69; 95% CI: 0.64–0.73) (P<0.001), EHBSH nomogram (0.67; 95% CI: 0.63–0.72) (P<0.001), AJCC TNM staging system (0.61; 95% CI: 0.56–0.65) (P<0.001), and MEGNA prognostic score (0.60; 95% CI: 0.56–0.65). Time-dependent ROCs showed that the dynamic AUCs for different time periods (1 year to 8 years) of PMS were larger than those of JHUSM (P<0.001), EHBSH (P<0.001), AJCC TNM staging system (P<0.001), and MEGNA prognostic score (P<0.001) (Figure 4A). The risk stratification results showed that the Kaplan-Meier method displayed four clearly separated survival curves (P<0.001 for the whole) with gradually decreasing survival according to the PMS (quartile 2 vs. 1: HR =3.51, 95% CI: 1.93–6.37, log-rank P<0.001; quartile 3 vs. 2: HR =2.01, 95% CI: 1.30–3.11, log-rank P=0.002; quartile 4 vs. 3: HR =2.44, 95% CI: 1.68–3.55, log-rank P<0.001) (Figure 4B). In contrast, although the P values reached statistical significance for the whole in the JHUSM nomogram (P<0.001), EHBSH nomogram (P<0.001), AJCC TNM staging system (P=0.001), and MEGNA prognostic score (P<0.001), none of them had the same step-by-step descending trend across the four quartiles or stages (JHUSM nomogram quartile 2 vs. 1: HR =1.37, 95% CI: 0.81–2.31, log-rank P=0.249; EHBSH nomogram quartile 2 vs. 1: HR =1.08, 95% CI: 0.62–1.87, log-rank P=0.793; EHBSH nomogram quartile 4 vs. 3: HR =1.32, 95% CI: 0.91–1.92, log-rank P=0.135; TNM stage III vs. II: HR =0.89, 95% CI: 0.54–1.45, log-rank P=0.614; MEGNA score of 1 vs. 0: HR =1.58, 95% CI: 0.78–3.21, log-rank P=0.284; MEGNA score of 2 vs. 1: HR =1.22, 95% CI: 0.84–1.77, log-rank P=0.289) (Figure 4C-4F).
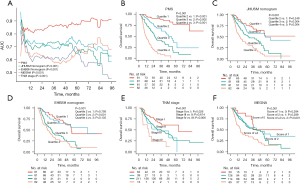
Additionally, subgroup analyses showed that the PMS remained significant in most subgroups according to clinical parameters, except for two subgroups with too few patients (8 and 14 patients in the hepatolithiasis and stage IV subgroups, respectively) (Figure S4).
PMS and AT
In our study, 21.6% (72/334) patients received AT after surgery. Among the 72 patients who had AT, 49 (68.1%) patients received gemcitabine-based chemotherapy; 20 (27.8%) patients had Transarterial Chemoembolization (TACE); and 3 (4.2%) patients had other regimens (FOLFOX, Tegafur or capecitabine). In contrast, only 1.5% (5/334) patients received preoperative TACE as neoadjuvant therapy. It has been suggested that not all ICC patients are suitable candidates for AT after surgery, and only patients at high risk of recurrence or death can benefit from AT (4). Therefore, we compared the ability of PMS and other nomograms or the staging system as tools to identify those who might benefit from AT. It was found that AT could improve OS in the quartile 4 patients of the PMS (HR =0.54; 95% CI: 0.32–0.91; log-rank P=0.043), whereas it failed to improve OS in the quartile 1, 2, and 3 patients (HR =1.01; 95% CI: 0.61–1.70; log-rank P=0.957) (Figure 5A,5B). In contrast, no survival differences were observed between patients receiving AT and these receiving no AT in the quartile 4 patients of the JHUSM nomogram (HR =0.97; 95% CI: 0.51–1.85; log-rank P=0.922), quartile 4 of EHBSH nomogram (HR =1.11; 95% CI: 0.58–2.13; log-rank P=0.743), TNM stage III B and IV (HR =0.95; 95% CI: 0.53–1.70; log-rank P=0.870), and MEGNA score of ≥3 (HR =1.19; 95% CI: 0.53–2.67; log-rank P=0.647) (Figure 5C-5F).
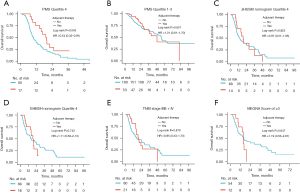
Underlying mechanism
In view of the good performance of the PMS in predicting prognosis and identifying appropriate candidates for AT, we explored the underlying mechanism of this prognosis’ relevance. First, the correlation analysis showed that the PMS was significantly associated with malignant biological behaviors (adjacent organ invasion, P=0.001; macrovascular invasion, P=0.014; microvascular invasion, P=0.014; lymph node metastasis, P<0.001; distant metastasis, P=0.021; TNM stage, P=0.023; differentiation, P<0.001; CA19-9, P<0.001) and background hepatitis B virus infection (P=0.029) (Figure 6A). However, no significant correlation (categorical variable, P<0.05 for t-test; continuous variable, r>0.3 and P<0.05) was observed between the PMS and other clinical or pathological parameters other than sex (P=0.033). Next, DMR analysis identified 34 hypermethylated and 2,564 hypomethylated regions in high-PMS patients compared with low-PMS patients. The genes whose promoter regions and bodies overlapped with hypomethylated regions were enriched for GTPase activity and cell adhesion pathways in gene ontology (GO) analysis and cancer-related pathways (calcium, Rap1, chemokine, and oxytocin signaling pathways) in KEGG pathway analysis (Figure 6B-6E). Considering the role of the chemokine signaling pathway in regulating immune response, we analyzed the immune-infiltration status using “MethylResolver” (27). The deconvolution results showed that high-PMS patients were associated with a decreased fraction of macrophages (P<0.001), eosinophils (P<0.001), naive T cells (P=0.032), memory T cells (P<0.001), CD8+ T cells (P=0.016), natural killer cells (NK cells) (P=0.001), and B cells (P=0.006) (Figure 6F). Meanwhile, high-PMS patients had significantly higher dendritic cells (P<0.001) and neutrophils (P<0.001) infiltration (Figure 6F). In brief, the PMS might represent tumor biology, pathway activation, and immunocyte infiltration to some extent, and constituent genes are discussed in the Discussion section.
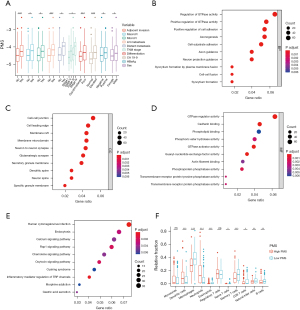
Discussion
Targetable genetic alterations offer an opportunity for eligible patients to receive target therapy, but an analysis of 117 patients in the validation cohort that were included in a recently published multi-omic study demonstrated that common mutations (FGFR2, IDH1/2, KRAS, TP53) provided little prognostic value (FGFR2, IDH1/2 and KRAS: P>0.05; TP53: P=0.038) (28,29). The prognosis predicting role of DNA methylation has been confirmed for various types of cancers (5,6) but received little attention for ICC. Therefore, we carried out this study to explore the effect of DNA methylation on prognosis of ICC. The prognostic signature in these studies were all based on methylation levels of individual CpG sites, which were mapped to individual probes in a 450k array (5,6). However, with the decrease of high-throughput sequencing cost, WGBS is increasingly being used in the research field of cancer epigenetics, which covers far more CpG sites than a 450k array (28 vs. 0.48 million CpG sites) (16,17). Correspondingly, investigators have paid more attention to regions instead of individual CpG sites. For example, DMRs, connected by consecutive differentially methylated CpG sites, have often been used as features to clarify methylation patterns and alterations, or to explore molecular subtypes (16,17). Inspired by this improvement, we proposed the concept of PMRs herein, which were constituted by successive PMC sites.
Compared with PMCs, our analysis showed that PMRs detected in the discovery cohort had a larger probability to be confirmed to be associated with prognosis in the validation cohort, which is a major concern in the biomarker hunting process. Next, functional genome enrichment analysis, KEGG pathway analysis, and motif enrichment analysis demonstrated that PMRs were biologically meaningful. Notably, most PMRs were enriched in potential regulatory regions (TFBS, ATAC-seq peaks, and regions marked by H3K27ac/H3K4me1), whereas only a small fraction of PMRs were enriched in CGI, which were the main regions of concern in the 450k array. It has also been observed by other investigators that most recurrent hypomethylated regions were outside CGI, CGI shores, or CGI shelves, and overlapped with putative regulatory regions instead (17). These findings imply that many important regulatory regions are located beyond regions covered by a 450k array, and WGBS is required to systematically examine the whole genome.
On the basis of PMRs, we developed the PMS based on methylation levels of 14 PMRs that precisely predicted long-term survival in the discovery cohort. Patients in the validation cohort tended to have better general condition, less advanced disease stages, and more frequently received AT, as socioeconomic conditions in the eastern coastal areas (validation/ZSHFU and TMUCIH cohort) are superior to those in southwest mainland China (discovery/WCHSU cohort). Despite the differences in clinicopathological factors and treatment strategies, PMS was confirmed in the validation cohort, which further illustrates the validity of the PMS. The PMS outperformed existing clinical parameter-based nomograms, staging system, or prognostic score, and the performance of the PMS was intact in subgroup analyses. Notably, the PMS represents a promising tool to identify suitable candidates for AT, and other nomograms or TNM staging systems failed to do so. However, due to relatively low proportion of patients receiving AT and no standard AT regimens in the study period, these results should be interpreted with caution. Future studies with higher proportion of patients receiving standard AT regimens are needed to confirm our findings. Collectively, these findings indicate a great prognostic value of the PMS, and there might be several explanations for its good performance. First, WGBS detects many more CpG sites than the array-based method, which provides an opportunity to incorporate more useful biomarkers into the PMS outside conditionally profiled areas in arrays. Next, we build the signature using methylation regions instead of individual CpG sites, as methylation regions have more universality and less occasionality compared with CpG sites. Additionally, the combination of two machine learning methods might increase the reasonability of selecting appropriate candidate PMRs included in the model from the high-dimensional data.
All PMRs in the PMS were located within the body, promoter region, or untranslated regions of specific genes, and none of them were in intergenic regions. Among 14 PMRs, nine PMRs were assigned to at least one regulatory element such as ATAC-seq peaks, potential enhancer regions marked by H3K27ac/H3K4me1, CGI, 5' UTR, 3' UTR, and the first intron. Whereas DNA methylation in the promoter region and CGI has been well characterized in terms of regulation of gene expression, an increasing number of studies has also revealed the role of DNA methylation in other regions in regulating gene expression, for example, 3' UTR or the first intron (30,31). Additionally, five PMRs fell into non-first introns and repetitive elements, in which the effect of DNA methylation on gene expression has been little characterized. The 14 PMRs included in our model corresponded with 16 genes (nine protein-coding genes, and seven long non-coding RNA genes), and most genes have been reported to be involved in tumorigenesis and progression in various types of cancers (32-46). The exact and specific roles of these genes in ICC warrant further exploration.
Apart from reviewing relevant research on constitutive genes, we also explored potential mechanisms by correlation analysis, pathway enrichment analysis, and immunological deconvolution. First, higher PMS was associated with more aggressive clinicopathologic behaviors (fox example, macrovascular invasion, lymph node metastasis), indicating that the PMS might be able to represent tumor biology nature to some degree. Next, compared with low-PMS patients, hypomethylated regions in high-PMS patients were enriched in cancer-related pathways. Considering the negative relationship between DNA methylation and gene expression in most cases, the enrichment results implied the activation of these pathways in high PMS patients, which was consistent with poorer survival of high PMS patients. Then, using “MethylResolver”, we found that high-PMS patients were associated with higher infiltration of neutrophils and dendritic cells and lower infiltration of macrophages, eosinophils, naive T cells, memory T cells, CD8+ T cells, NK cells and B cells. An adverse role of neutrophils and a favorable role of NK cells in prognosis of ICC have been reported, which is consistent with our results. However, various roles in predicting prognosis have been reported for macrophages and CD8+ T cells (47-54), and the roles of other immune subsets’ infiltration have not been reported yet in ICC. In short summary, the PMS can reflect tumor biological behaviors, signaling pathway activation, and immune microenvironment to some degree, which further confirms the validity of the PMS and might facilitate therapeutic strategies making (fox example, immunotherapy) in ICC patients.
Our study has several limitations. First, the follow-up time was relatively short and not balanced in the training and validation cohorts, partially accounting for the C-index gap between the two cohorts. However, ICC patients in our study were diagnosed with relatively advanced stages (stage III and IV: 68.5%), and most death events occurred within 2 years (120/168, 71.4%), which mitigated the effects of follow-up time to some extent. Second, none of the patients included in our study received adjuvant immunotherapies, and we are not able to determine the value of the PMS in predicting response to immunotherapies, although our preliminary analysis showed close relationship between the PMS and various immune cells infiltration. Third, we only included Chinese patients in our study, and the PMS needs to be validated first in a Western cohort before applying it to the Western population. A customized methylation panel covering all CpGs in the PMS might be more appropriate for further validation, as we already have enough WGBS data and the customized methylation panel will make it more affordable and convenient in future clinical practice.
Conclusions
To the best of our knowledge, our study is the first to generate deep WGBS data for tumor samples from a large cohort of ICC patients. Utilizing the base-resolution methylation profiles, we proposed the concept of PMRs and identified 14 PMRs to construct a methylation signature called the PMS, which could robustly predict long-term survival and identify candidate patients for AT after surgery. The PMS outperformed the existing clinical parameter-derived nomograms, prognostic scores or staging system. Our findings may facilitate the decisions in the treatment of ICC patients and help avoid the undertreatment for patients with high risk of recurrence or death and reduce unnecessary overtreatment for patients with favorable prognosis.
Acknowledgments
We are most grateful to West China Biobanks, Department of Clinical Research Management, West China Hospital, Sichuan University for their support of human tissue samples.
Funding: This work was supported by The National Key Technologies R&D Program of China (No. 2018YFC1106800), The 1.3.5 project for disciplines of excellence, West China Hospital, Sichuan University (No. ZYJC18008), and The Natural Science Foundation of China (Nos. 91859105, 81773012, 81872004, 81802302 and 81902401).
Footnote
Reporting Checklist: The authors have completed the TRIPOD reporting checklist. Available at https://hbsn.amegroups.com/article/view/10.21037/hbsn-21-424/rc
Data Sharing Statement: Available at https://hbsn.amegroups.com/article/view/10.21037/hbsn-21-424/dss
Conflicts of Interest: All authors have completed the ICMJE uniform disclosure form (available at https://hbsn.amegroups.com/article/view/10.21037/hbsn-21-424/coif). The authors have no conflicts of interest to declare.
Ethical Statement: The authors are accountable for all aspects of the work in ensuring that questions related to the accuracy or integrity of any part of the work are appropriately investigated and resolved. The study was conducted in accordance with the Declaration of Helsinki (as revised in 2013). The study was approved by the Ethical Committee of West China Hospital of Sichuan University (No. 2019-833), Zhongshan Hospital of Fudan University (No. B2017-060R) and Tianjin Medical University Cancer Institute and Hospital (No. bc2020012), and individual consent for this retrospective analysis was waived.
Open Access Statement: This is an Open Access article distributed in accordance with the Creative Commons Attribution-NonCommercial-NoDerivs 4.0 International License (CC BY-NC-ND 4.0), which permits the non-commercial replication and distribution of the article with the strict proviso that no changes or edits are made and the original work is properly cited (including links to both the formal publication through the relevant DOI and the license). See: https://creativecommons.org/licenses/by-nc-nd/4.0/.
References
- Kudo M, Izumi N, Kokudo N, et al. Report of the 22nd nationwide follow-up Survey of Primary Liver Cancer in Japan (2012-2013). Hepatol Res 2022;52:5-66. [Crossref] [PubMed]
- Zhang XF, Beal EW, Bagante F, et al. Early versus late recurrence of intrahepatic cholangiocarcinoma after resection with curative intent. Br J Surg 2018;105:848-56. [Crossref] [PubMed]
- Hu LS, Zhang XF, Weiss M, et al. Recurrence Patterns and Timing Courses Following Curative-Intent Resection for Intrahepatic Cholangiocarcinoma. Ann Surg Oncol 2019;26:2549-57. [Crossref] [PubMed]
- Chun YS, Javle M. Systemic and Adjuvant Therapies for Intrahepatic Cholangiocarcinoma. Cancer Control 2017;24:1073274817729241. [Crossref] [PubMed]
- Xu RH, Wei W, Krawczyk M, et al. Circulating tumour DNA methylation markers for diagnosis and prognosis of hepatocellular carcinoma. Nat Mater 2017;16:1155-61. [Crossref] [PubMed]
- Gündert M, Edelmann D, Benner A, et al. Genome-wide DNA methylation analysis reveals a prognostic classifier for non-metastatic colorectal cancer (ProMCol classifier). Gut 2019;68:101-10. [Crossref] [PubMed]
- Lee S, Kim WH, Jung HY, et al. Aberrant CpG island methylation of multiple genes in intrahepatic cholangiocarcinoma. Am J Pathol 2002;161:1015-22. [Crossref] [PubMed]
- Yang B, House MG, Guo M, et al. Promoter methylation profiles of tumor suppressor genes in intrahepatic and extrahepatic cholangiocarcinoma. Mod Pathol 2005;18:412-20. [Crossref] [PubMed]
- Kim BH, Cho NY, Choi M, et al. Methylation profiles of multiple CpG island loci in extrahepatic cholangiocarcinoma versus those of intrahepatic cholangiocarcinomas. Arch Pathol Lab Med 2007;131:923-30. [Crossref] [PubMed]
- Andresen K, Boberg KM, Vedeld HM, et al. Novel target genes and a valid biomarker panel identified for cholangiocarcinoma. Epigenetics 2012;7:1249-57. [Crossref] [PubMed]
- Goeppert B, Konermann C, Schmidt CR, et al. Global alterations of DNA methylation in cholangiocarcinoma target the Wnt signaling pathway. Hepatology 2014;59:544-54. [Crossref] [PubMed]
- Sriraksa R, Zeller C, Dai W, et al. Aberrant DNA methylation at genes associated with a stem cell-like phenotype in cholangiocarcinoma tumors. Cancer Prev Res (Phila) 2013;6:1348-55. [Crossref] [PubMed]
- Farshidfar F, Zheng S, Gingras MC, et al. Integrative Genomic Analysis of Cholangiocarcinoma Identifies Distinct IDH-Mutant Molecular Profiles. Cell Rep 2017;19:2878-80. [Crossref] [PubMed]
- Jusakul A, Cutcutache I, Yong CH, et al. Whole-Genome and Epigenomic Landscapes of Etiologically Distinct Subtypes of Cholangiocarcinoma. Cancer Discov 2017;7:1116-35. [Crossref] [PubMed]
- Goeppert B, Toth R, Singer S, et al. Integrative Analysis Defines Distinct Prognostic Subgroups of Intrahepatic Cholangiocarcinoma. Hepatology 2019;69:2091-106. [Crossref] [PubMed]
- Li J, Xu C, Lee HJ, et al. A genomic and epigenomic atlas of prostate cancer in Asian populations. Nature 2020;580:93-9. [Crossref] [PubMed]
- Zhao SG, Chen WS, Li H, et al. The DNA methylation landscape of advanced prostate cancer. Nat Genet 2020;52:778-89. [Crossref] [PubMed]
- Sun D, Xi Y, Rodriguez B, et al. MOABS: model based analysis of bisulfite sequencing data. Genome Biol 2014;15:R38. [Crossref] [PubMed]
- Ishwaran H, Kogalur UB, Gorodeski EZ, et al. Highdimensional variable selection for survival data. J Am Stat Assoc 2010;105:205-17. [Crossref]
- Wang Y, Li J, Xia Y, et al. Prognostic nomogram for intrahepatic cholangiocarcinoma after partial hepatectomy. J Clin Oncol 2013;31:1188-95. [Crossref] [PubMed]
- Hyder O, Marques H, Pulitano C, et al. A nomogram to predict long-term survival after resection for intrahepatic cholangiocarcinoma: an Eastern and Western experience. JAMA Surg 2014;149:432-8. [Crossref] [PubMed]
- Li J, Wang Q, Lei Z, et al. Adjuvant Transarterial Chemoembolization Following Liver Resection for Intrahepatic Cholangiocarcinoma Based on Survival Risk Stratification. Oncologist 2015;20:640-7. [Crossref] [PubMed]
- Vallejo A, Erice O, Entrialgo-Cadierno R, et al. FOSL1 promotes cholangiocarcinoma via transcriptional effectors that could be therapeutically targeted. J Hepatol 2021;75:363-76. [Crossref] [PubMed]
- You Z, Xu J, Li B, et al. The mechanism of ATF3 repression of epithelial-mesenchymal transition and suppression of cell viability in cholangiocarcinoma via p53 signal pathway. J Cell Mol Med 2019;23:2184-93. [Crossref] [PubMed]
- Jeon BS, Lee BW, Yoon BI. Abstract 1473: Fra-2 and JunB are overexpressed in precancerous bile ducts and primary/transplanted hamster cholangiocarcinomas with a strong positive correlation. Cancer Res 2010;70:1473. [Crossref]
- Raoof M, Dumitra S, Ituarte PHG, et al. Development and Validation of a Prognostic Score for Intrahepatic Cholangiocarcinoma. JAMA Surg 2017;152:e170117. [Crossref] [PubMed]
- Arneson D, Yang X, Wang K. MethylResolver-a method for deconvoluting bulk DNA methylation profiles into known and unknown cell contents. Commun Biol 2020;3:422. [Crossref] [PubMed]
- Petrowsky H, Fritsch R, Guckenberger M, et al. Modern therapeutic approaches for the treatment of malignant liver tumours. Nat Rev Gastroenterol Hepatol 2020;17:755-72. [Crossref] [PubMed]
- Dong L, Lu D, Chen R, et al. Proteogenomic characterization identifies clinically relevant subgroups of intrahepatic cholangiocarcinoma. Cancer Cell 2022;40:70-87.e15. [Crossref] [PubMed]
- McGuire MH, Herbrich SM, Dasari SK, et al. Pan-cancer genomic analysis links 3'UTR DNA methylation with increased gene expression in T cells. EBioMedicine 2019;43:127-37. [Crossref] [PubMed]
- Anastasiadi D, Esteve-Codina A, Piferrer F. Consistent inverse correlation between DNA methylation of the first intron and gene expression across tissues and species. Epigenetics Chromatin 2018;11:37. [Crossref] [PubMed]
- De Braekeleer M, Douet-Guilbert N, De Braekeleer E. Prognostic impact of p15 gene aberrations in acute leukemia. Leuk Lymphoma 2017;58:257-65. [Crossref] [PubMed]
- Ren WH, Li YW, Li R, et al. P15 gene methylation in hepatocellular carcinomas: a systematic review and meta-analysis. Int J Clin Exp Med 2015;8:4762-8. [PubMed]
- Kudo T, Ikeda M, Nishikawa M, et al. The RASSF3 candidate tumor suppressor induces apoptosis and G1-S cell-cycle arrest via p53. Cancer Res 2012;72:2901-11. [Crossref] [PubMed]
- Fukatsu A, Ishiguro F, Tanaka I, et al. RASSF3 downregulation increases malignant phenotypes of non-small cell lung cancer. Lung Cancer 2014;83:23-9. [Crossref] [PubMed]
- Wen H, Li Y, Xi Y, et al. ZMYND11 links histone H3.3K36me3 to transcription elongation and tumour suppression. Nature 2014;508:263-8. [Crossref] [PubMed]
- Li J, Galbo PM Jr, Gong W, et al. ZMYND11-MBTD1 induces leukemogenesis through hijacking NuA4/TIP60 acetyltransferase complex and a PWWP-mediated chromatin association mechanism. Nat Commun 2021;12:1045. [Crossref] [PubMed]
- Plotnik JP, Hollenhorst PC. Interaction with ZMYND11 mediates opposing roles of Ras-responsive transcription factors ETS1 and ETS2. Nucleic Acids Res 2017;45:4452-62. [Crossref] [PubMed]
- de la Rosa J, Weber J, Friedrich MJ, et al. A single-copy Sleeping Beauty transposon mutagenesis screen identifies new PTEN-cooperating tumor suppressor genes. Nat Genet 2017;49:730-41. [Crossref] [PubMed]
- Shibolet O, Giallourakis C, Rosenberg I, et al. AKAP13, a RhoA GTPase-specific guanine exchange factor, is a novel regulator of TLR2 signaling. J Biol Chem 2007;282:35308-17. [Crossref] [PubMed]
- Raponi M, Harousseau JL, Lancet JE, et al. Identification of molecular predictors of response in a study of tipifarnib treatment in relapsed and refractory acute myelogenous leukemia. Clin Cancer Res 2007;13:2254-60. [Crossref] [PubMed]
- Zhang J, Zhang P, Wei Y, et al. Deubiquitylation and stabilization of PTEN by USP13. Nat Cell Biol 2013;15:1486-94. [Crossref] [PubMed]
- Han C, Yang L, Choi HH, et al. Amplification of USP13 drives ovarian cancer metabolism. Nat Commun 2016;7:13525. [Crossref] [PubMed]
- Cheung HH, Yang Y, Lee TL, et al. Hypermethylation of genes in testicular embryonal carcinomas. Br J Cancer 2016;114:230-6. [Crossref] [PubMed]
- Shang C, Ao CN, Cheong CC, et al. Long Non-coding RNA CDKN2B Antisense RNA 1 Gene Contributes to Paclitaxel Resistance in Endometrial Carcinoma. Front Oncol 2019;9:27. [Crossref] [PubMed]
- Marchi RD, Mathias C, Reiter GAK, et al. Association between SNP rs527616 in lncRNA AQP4-AS1 and susceptibility to breast cancer in a southern Brazilian population. Genet Mol Biol 2021;44:e20200216. [Crossref] [PubMed]
- Gu FM, Gao Q, Shi GM, et al. Intratumoral IL-17+ cells and neutrophils show strong prognostic significance in intrahepatic cholangiocarcinoma. Ann Surg Oncol 2012;19:2506-14. [Crossref] [PubMed]
- Fukuda Y, Asaoka T, Eguchi H, et al. Endogenous CXCL9 affects prognosis by regulating tumor-infiltrating natural killer cells in intrahepatic cholangiocarcinoma. Cancer Sci 2020;111:323-33. [Crossref] [PubMed]
- Zhou Z, Wang P, Sun R, et al. Tumor-associated neutrophils and macrophages interaction contributes to intrahepatic cholangiocarcinoma progression by activating STAT3. J Immunother Cancer 2021;9:e001946. [Crossref] [PubMed]
- Hasita H, Komohara Y, Okabe H, et al. Significance of alternatively activated macrophages in patients with intrahepatic cholangiocarcinoma. Cancer Sci 2010;101:1913-9. [Crossref] [PubMed]
- Sun D, Luo T, Dong P, et al. CD86+/CD206+ tumor-associated macrophages predict prognosis of patients with intrahepatic cholangiocarcinoma. PeerJ 2020;8:e8458. [Crossref] [PubMed]
- Deng M, Li SH, Fu X, et al. Relationship between PD-L1 expression, CD8+ T-cell infiltration and prognosis in intrahepatic cholangiocarcinoma patients. Cancer Cell Int 2021;21:371. [Crossref] [PubMed]
- Asahi Y, Hatanaka KC, Hatanaka Y, et al. Prognostic impact of CD8+ T cell distribution and its association with the HLA class I expression in intrahepatic cholangiocarcinoma. Surg Today 2020;50:931-40. [Crossref] [PubMed]
- Zhu Y, Wang XY, Zhang Y, et al. Programmed death ligand 1 expression in human intrahepatic cholangiocarcinoma and its association with prognosis and CD8+ T-cell immune responses. Cancer Manag Res 2018;10:4113-23. [Crossref] [PubMed]